Apr 15, 2025
Abstract:Collaborative assistants, or chatbots, are data-driven decision support systems that enable natural interaction for task completion. While they can meet critical needs in modern society, concerns about their reliability and trustworthiness persist. In particular, Large Language Model (LLM)-based chatbots like ChatGPT, Gemini, and DeepSeek are becoming more accessible. However, such chatbots have limitations, including their inability to explain response generation, the risk of generating problematic content, the lack of standardized testing for reliability, and the need for deep AI expertise and extended development times. These issues make chatbots unsuitable for trust-sensitive applications like elections or healthcare. To address these concerns, we introduce SafeChat, a general architecture for building safe and trustworthy chatbots, with a focus on information retrieval use cases. Key features of SafeChat include: (a) safety, with a domain-agnostic design where responses are grounded and traceable to approved sources (provenance), and 'do-not-respond' strategies to prevent harmful answers; (b) usability, with automatic extractive summarization of long responses, traceable to their sources, and automated trust assessments to communicate expected chatbot behavior, such as sentiment; and (c) fast, scalable development, including a CSV-driven workflow, automated testing, and integration with various devices. We implemented SafeChat in an executable framework using the open-source chatbot platform Rasa. A case study demonstrates its application in building ElectionBot-SC, a chatbot designed to safely disseminate official election information. SafeChat is being used in many domains, validating its potential, and is available at: https://github.com/ai4society/trustworthy-chatbot.
Via
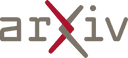