What is Topic Modeling? Topic modeling is a type of statistical modeling for discovering the abstract topics that occur in a collection of documents.
Papers and Code
Jun 05, 2025
Abstract:We introduce an approach to topic modelling with document-level covariates that remains tractable in the face of large text corpora. This is achieved by de-emphasizing the role of parameter estimation in an underlying probabilistic model, assuming instead that the data come from a fixed but unknown distribution whose statistical functionals are of interest. We propose combining a convex formulation of non-negative matrix factorization with standard regression techniques as a fast-to-compute and useful estimate of such a functional. Uncertainty quantification can then be achieved by reposing non-parametric resampling methods on top of this scheme. This is in contrast to popular topic modelling paradigms, which posit a complex and often hard-to-fit generative model of the data. We argue that the simple, non-parametric approach advocated here is faster, more interpretable, and enjoys better inferential justification than said generative models. Finally, our methods are demonstrated with an application analysing covariate effects on discourse of flavours attributed to Canadian beers.
Via
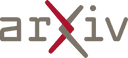
Jun 09, 2025
Abstract:Multimodal Large Language Models (MLLMs) promise advanced vision language capabilities, yet their effectiveness in visually presented mathematics remains underexplored. This paper analyzes the development and evaluation of MLLMs for mathematical problem solving, focusing on diagrams, multilingual text, and symbolic notation. We then assess several models, including GPT 4o, Pixtral, Qwen VL, Llama 3.2 Vision variants, and Gemini 2.0 Flash in a multilingual Kangaroo style benchmark spanning English, French, Spanish, and Catalan. Our experiments reveal four key findings. First, overall precision remains moderate across geometry, visual algebra, logic, patterns, and combinatorics: no single model excels in every topic. Second, while most models see improved accuracy with questions that do not have images, the gain is often limited; performance for some remains nearly unchanged without visual input, indicating underutilization of diagrammatic information. Third, substantial variation exists across languages and difficulty levels: models frequently handle easier items but struggle with advanced geometry and combinatorial reasoning. Notably, Gemini 2.0 Flash achieves the highest precision on image based tasks, followed by Qwen VL 2.5 72B and GPT 4o, though none approach human level performance. Fourth, a complementary analysis aimed at distinguishing whether models reason or simply recite reveals that Gemini and GPT 4o stand out for their structured reasoning and consistent accuracy. In contrast, Pixtral and Llama exhibit less consistent reasoning, often defaulting to heuristics or randomness when unable to align their outputs with the given answer options.
* 16 pages, 4 figures
Via
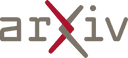
Jun 12, 2025
Abstract:We show that reinforcement learning with verifiable rewards (RLVR) can elicit strong mathematical reasoning in certain models even with spurious rewards that have little, no, or even negative correlation with the correct answer. For example, RLVR improves MATH-500 performance for Qwen2.5-Math-7B in absolute points by 21.4% (random reward), 13.8% (format reward), 24.1% (incorrect label), 26.0% (1-shot RL), and 27.1% (majority voting) -- nearly matching the 29.1% gained with ground truth rewards. However, the spurious rewards that work for Qwen often fail to yield gains with other model families like Llama3 or OLMo2. In particular, we find code reasoning -- thinking in code without actual code execution -- to be a distinctive Qwen2.5-Math behavior that becomes significantly more frequent after RLVR, from 65% to over 90%, even with spurious rewards. Overall, we hypothesize that, given the lack of useful reward signal, RLVR must somehow be surfacing useful reasoning representations learned during pretraining, although the exact mechanism remains a topic for future work. We suggest that future RLVR research should possibly be validated on diverse models rather than a single de facto choice, as we show that it is easy to get significant performance gains on Qwen models even with completely spurious reward signals.
Via
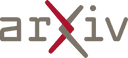
Jun 13, 2025
Abstract:People get informed of a daily task plan through diverse media involving both texts and images. However, most prior research only focuses on LLM's capability of textual plan generation. The potential of large-scale models in providing text-image plans remains understudied. Generating high-quality text-image plans faces two main challenges: ensuring consistent alignment between two modalities and keeping coherence among visual steps. To address these challenges, we propose a novel framework that generates and refines text-image plans step-by-step. At each iteration, our framework (1) drafts the next textual step based on the prediction history; (2) edits the last visual step to obtain the next one; (3) extracts PDDL-like visual information; and (4) refines the draft with the extracted visual information. The textual and visual step produced in stage (4) and (2) will then serve as inputs for the next iteration. Our approach offers a plug-and-play improvement to various backbone models, such as Mistral-7B, Gemini-1.5, and GPT-4o. To evaluate the effectiveness of our approach, we collect a new benchmark consisting of 1,100 tasks and their text-image pair solutions covering 11 daily topics. We also design and validate a new set of metrics to evaluate the multimodal consistency and coherence in text-image plans. Extensive experiment results show the effectiveness of our approach on a range of backbone models against competitive baselines. Our code and data are available at https://github.com/psunlpgroup/MPlanner.
* 18 pages, 10 figures; Accepted to ACL 2025 Findings
Via
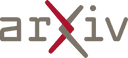
Jun 10, 2025
Abstract:Large language model (LLM) unlearning has become a critical topic in machine learning, aiming to eliminate the influence of specific training data or knowledge without retraining the model from scratch. A variety of techniques have been proposed, including Gradient Ascent, model editing, and re-steering hidden representations. While existing surveys often organize these methods by their technical characteristics, such classifications tend to overlook a more fundamental dimension: the underlying intention of unlearning--whether it seeks to truly remove internal knowledge or merely suppress its behavioral effects. In this SoK paper, we propose a new taxonomy based on this intention-oriented perspective. Building on this taxonomy, we make three key contributions. First, we revisit recent findings suggesting that many removal methods may functionally behave like suppression, and explore whether true removal is necessary or achievable. Second, we survey existing evaluation strategies, identify limitations in current metrics and benchmarks, and suggest directions for developing more reliable and intention-aligned evaluations. Third, we highlight practical challenges--such as scalability and support for sequential unlearning--that currently hinder the broader deployment of unlearning methods. In summary, this work offers a comprehensive framework for understanding and advancing unlearning in generative AI, aiming to support future research and guide policy decisions around data removal and privacy.
Via
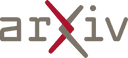
Jun 14, 2025
Abstract:Navigating the vast and rapidly growing body of scientific literature is a formidable challenge for aspiring researchers. Current approaches to supporting research idea generation often rely on generic large language models (LLMs). While LLMs are effective at aiding comprehension and summarization, they often fall short in guiding users toward practical research ideas due to their limitations. In this study, we present a novel structural framework for research ideation. Our framework, The Budget AI Researcher, uses retrieval-augmented generation (RAG) chains, vector databases, and topic-guided pairing to recombine concepts from hundreds of machine learning papers. The system ingests papers from nine major AI conferences, which collectively span the vast subfields of machine learning, and organizes them into a hierarchical topic tree. It uses the tree to identify distant topic pairs, generate novel research abstracts, and refine them through iterative self-evaluation against relevant literature and peer reviews, generating and refining abstracts that are both grounded in real-world research and demonstrably interesting. Experiments using LLM-based metrics indicate that our method significantly improves the concreteness of generated research ideas relative to standard prompting approaches. Human evaluations further demonstrate a substantial enhancement in the perceived interestingness of the outputs. By bridging the gap between academic data and creative generation, the Budget AI Researcher offers a practical, free tool for accelerating scientific discovery and lowering the barrier for aspiring researchers. Beyond research ideation, this approach inspires solutions to the broader challenge of generating personalized, context-aware outputs grounded in evolving real-world knowledge.
* Intended for AAAI's AI4Research Workshop
Via
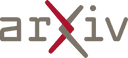
Jun 15, 2025
Abstract:The rapid growth of scientific literature demands robust tools for automated survey-generation. However, current large language model (LLM)-based methods often lack in-depth analysis, structural coherence, and reliable citations. To address these limitations, we introduce SciSage, a multi-agent framework employing a reflect-when-you-write paradigm. SciSage features a hierarchical Reflector agent that critically evaluates drafts at outline, section, and document levels, collaborating with specialized agents for query interpretation, content retrieval, and refinement. We also release SurveyScope, a rigorously curated benchmark of 46 high-impact papers (2020-2025) across 11 computer science domains, with strict recency and citation-based quality controls. Evaluations demonstrate that SciSage outperforms state-of-the-art baselines (LLM x MapReduce-V2, AutoSurvey), achieving +1.73 points in document coherence and +32% in citation F1 scores. Human evaluations reveal mixed outcomes (3 wins vs. 7 losses against human-written surveys), but highlight SciSage's strengths in topical breadth and retrieval efficiency. Overall, SciSage offers a promising foundation for research-assistive writing tools.
Via
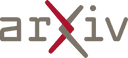
Jun 12, 2025
Abstract:The increasing adoption of artificial intelligence in telecommunications has raised interest in the capability of Large Language Models (LLMs) to address domain-specific, mathematically intensive tasks. Although recent advancements have improved the performance of LLMs in general mathematical reasoning, their effectiveness within specialized domains, such as signal processing, network optimization, and performance analysis, remains largely unexplored. To address this gap, we introduce TeleMath, the first benchmark dataset specifically designed to evaluate LLM performance in solving mathematical problems with numerical solutions in the telecommunications domain. Comprising 500 question-answer (QnA) pairs, TeleMath covers a wide spectrum of topics in the telecommunications field. This paper outlines the proposed QnAs generation pipeline, starting from a selected seed of problems crafted by Subject Matter Experts. The evaluation of a wide range of open-source LLMs reveals that best performance on TeleMath is achieved by recent models explicitly designed for mathematical or logical reasoning. In contrast, general-purpose models, even those with a large number of parameters, often struggle with these challenges. We have released the dataset and the evaluation code to ease result reproducibility and support future research.
* 6 pages
Via
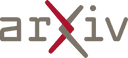
Jun 12, 2025
Abstract:Conversational search enables multi-turn interactions between users and systems to fulfill users' complex information needs. During this interaction, the system should understand the users' search intent within the conversational context and then return the relevant information through a flexible, dialogue-based interface. The recent powerful large language models (LLMs) with capacities of instruction following, content generation, and reasoning, attract significant attention and advancements, providing new opportunities and challenges for building up intelligent conversational search systems. This tutorial aims to introduce the connection between fundamentals and the emerging topics revolutionized by LLMs in the context of conversational search. It is designed for students, researchers, and practitioners from both academia and industry. Participants will gain a comprehensive understanding of both the core principles and cutting-edge developments driven by LLMs in conversational search, equipping them with the knowledge needed to contribute to the development of next-generation conversational search systems.
* Accepted by Tutorial Track in SIGIR 2025
Via
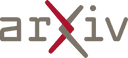
Jun 11, 2025
Abstract:In this short note, we report and analyze a striking event: OpenAI's large language model o3 has outwitted all students in a university exam on thermodynamics. The thermodynamics exam is a difficult hurdle for most students, where they must show that they have mastered the fundamentals of this important topic. Consequently, the failure rates are very high, A-grades are rare - and they are considered proof of the students' exceptional intellectual abilities. This is because pattern learning does not help in the exam. The problems can only be solved by knowledgeably and creatively combining principles of thermodynamics. We have given our latest thermodynamics exam not only to the students but also to OpenAI's most powerful reasoning model, o3, and have assessed the answers of o3 exactly the same way as those of the students. In zero-shot mode, the model o3 solved all problems correctly, better than all students who took the exam; its overall score was in the range of the best scores we have seen in more than 10,000 similar exams since 1985. This is a turning point: machines now excel in complex tasks, usually taken as proof of human intellectual capabilities. We discuss the consequences this has for the work of engineers and the education of future engineers.
* This document is the unedited Author's version of a yet to be
Submitted Work to Physical Review Physics Education Research. 15 pages, 2
figures, Graphical Abstract, Highlights and SI available (12 pages)
Via
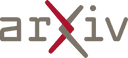