What is Sentiment Analysis? Sentiment analysis is the process of determining the sentiment of a piece of text, such as a tweet or a review.
Papers and Code
Mar 25, 2025
Abstract:Understanding how emotions are expressed across languages is vital for building culturally-aware and inclusive NLP systems. However, emotion expression in African languages is understudied, limiting the development of effective emotion detection tools in these languages. In this work, we present a cross-linguistic analysis of emotion expression in 15 African languages. We examine four key dimensions of emotion representation: text length, sentiment polarity, emotion co-occurrence, and intensity variations. Our findings reveal diverse language-specific patterns in emotional expression -- with Somali texts typically longer, while others like IsiZulu and Algerian Arabic show more concise emotional expression. We observe a higher prevalence of negative sentiment in several Nigerian languages compared to lower negativity in languages like IsiXhosa. Further, emotion co-occurrence analysis demonstrates strong cross-linguistic associations between specific emotion pairs (anger-disgust, sadness-fear), suggesting universal psychological connections. Intensity distributions show multimodal patterns with significant variations between language families; Bantu languages display similar yet distinct profiles, while Afroasiatic languages and Nigerian Pidgin demonstrate wider intensity ranges. These findings highlight the need for language-specific approaches to emotion detection while identifying opportunities for transfer learning across related languages.
Via
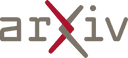
Mar 04, 2025
Abstract:This paper investigates the challenges of affect control in large language models (LLMs), focusing on their ability to express appropriate emotional states during extended dialogues. We evaluated state-of-the-art open-weight LLMs to assess their affective expressive range in terms of arousal and valence. Our study employs a novel methodology combining LLM-based sentiment analysis with multiturn dialogue simulations between LLMs. We quantify the models' capacity to express a wide spectrum of emotions and how they fluctuate during interactions. Our findings reveal significant variations among LLMs in their ability to maintain consistent affect, with some models demonstrating more stable emotional trajectories than others. Furthermore, we identify key challenges in affect control, including difficulties in producing and maintaining extreme emotional states and limitations in adapting affect to changing conversational contexts. These findings have important implications for the development of more emotionally intelligent AI systems and highlight the need for improved affect modelling in LLMs.
Via
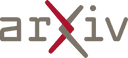
Mar 07, 2025
Abstract:In recent years, the application of behavioral testing in Natural Language Processing (NLP) model evaluation has experienced a remarkable and substantial growth. However, the existing methods continue to be restricted by the requirements for manual labor and the limited scope of capability assessment. To address these limitations, we introduce AutoTestForge, an automated and multidimensional testing framework for NLP models in this paper. Within AutoTestForge, through the utilization of Large Language Models (LLMs) to automatically generate test templates and instantiate them, manual involvement is significantly reduced. Additionally, a mechanism for the validation of test case labels based on differential testing is implemented which makes use of a multi-model voting system to guarantee the quality of test cases. The framework also extends the test suite across three dimensions, taxonomy, fairness, and robustness, offering a comprehensive evaluation of the capabilities of NLP models. This expansion enables a more in-depth and thorough assessment of the models, providing valuable insights into their strengths and weaknesses. A comprehensive evaluation across sentiment analysis (SA) and semantic textual similarity (STS) tasks demonstrates that AutoTestForge consistently outperforms existing datasets and testing tools, achieving higher error detection rates (an average of $30.89\%$ for SA and $34.58\%$ for STS). Moreover, different generation strategies exhibit stable effectiveness, with error detection rates ranging from $29.03\% - 36.82\%$.
* 15 pages, 4 figures, Under review
Via
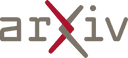
Feb 17, 2025
Abstract:Aspect-based sentiment analysis (ABSA) is a crucial task in information extraction and sentiment analysis, aiming to identify aspects with associated sentiment elements in text. However, existing ABSA datasets are predominantly English-centric, limiting the scope for multilingual evaluation and research. To bridge this gap, we present M-ABSA, a comprehensive dataset spanning 7 domains and 21 languages, making it the most extensive multilingual parallel dataset for ABSA to date. Our primary focus is on triplet extraction, which involves identifying aspect terms, aspect categories, and sentiment polarities. The dataset is constructed through an automatic translation process with human review to ensure quality. We perform extensive experiments using various baselines to assess performance and compatibility on M-ABSA. Our empirical findings highlight that the dataset enables diverse evaluation tasks, such as multilingual and multi-domain transfer learning, and large language model evaluation, underscoring its inclusivity and its potential to drive advancements in multilingual ABSA research.
Via
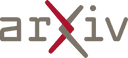
Feb 18, 2025
Abstract:Emojis are being frequently used in todays digital world to express from simple to complex thoughts more than ever before. Hence, they are also being used in sentiment analysis and targeted marketing campaigns. In this work, we performed sentiment analysis of Tweets as well as on emoji dataset from the Kaggle. Since tweets are sentences we have used Universal Sentence Encoder (USE) and Sentence Bidirectional Encoder Representations from Transformers (SBERT) end-to-end sentence embedding models to generate the embeddings which are used to train the Standard fully connected Neural Networks (NN), and LSTM NN models. We observe the text classification accuracy was almost the same for both the models around 98 percent. On the contrary, when the validation set was built using emojis that were not present in the training set then the accuracy of both the models reduced drastically to 70 percent. In addition, the models were also trained using the distributed training approach instead of a traditional singlethreaded model for better scalability. Using the distributed training approach, we were able to reduce the run-time by roughly 15% without compromising on accuracy. Finally, as part of explainable AI the Shap algorithm was used to explain the model behaviour and check for model biases for the given feature set.
* Journal of Advances in Information Technology Vol. 13, No. 2,
April 2022
Via
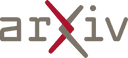
Feb 19, 2025
Abstract:Aspect-based sentiment analysis (ABSA) is a sequence labeling task that has garnered growing research interest in multilingual contexts. However, recent studies lack more robust feature alignment and finer aspect-level alignment. In this paper, we propose a novel framework, Multi-Scale and Multi-Objective optimization (MSMO) for cross-lingual ABSA. During multi-scale alignment, we achieve cross-lingual sentence-level and aspect-level alignment, aligning features of aspect terms in different contextual environments. Specifically, we introduce code-switched bilingual sentences into the language discriminator and consistency training modules to enhance the model's robustness. During multi-objective optimization, we design two optimization objectives: supervised training and consistency training, aiming to enhance cross-lingual semantic alignment. To further improve model performance, we incorporate distilled knowledge of the target language into the model. Results show that MSMO significantly enhances cross-lingual ABSA by achieving state-of-the-art performance across multiple languages and models.
Via
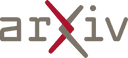
Mar 04, 2025
Abstract:The COVID-19 pandemic has profoundly affected the normal course of life -- from lock-downs and virtual meetings to the unprecedentedly swift creation of vaccines. To halt the COVID-19 pandemic, the world has started preparing for the global vaccine roll-out. In an effort to navigate the immense volume of information about COVID-19, the public has turned to social networks. Among them, $\mathbb{X}$ (formerly Twitter) has played a key role in distributing related information. Most people are not trained to interpret medical research and remain skeptical about the efficacy of new vaccines. Measuring their reactions and perceptions is gaining significance in the fight against COVID-19. To assess the public perception regarding the COVID-19 vaccine, our work applies a sentiment analysis approach, using natural language processing of $\mathbb{X}$ data. We show how to use textual analytics and textual data visualization to discover early insights (for example, by analyzing the most frequently used keywords and hashtags). Furthermore, we look at how people's sentiments vary across the countries. Our results indicate that although the overall reaction to the vaccine is positive, there are also negative sentiments associated with the tweets, especially when examined at the country level. Additionally, from the extracted tweets, we manually labeled 100 tweets as positive and 100 tweets as negative and trained various One-Class Classifiers (OCCs). The experimental results indicate that the S-SVDD classifiers outperform other OCCs.
Via
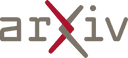
Feb 27, 2025
Abstract:Aspect-Sentiment Triplet Extraction (ASTE) is one of the most challenging and complex tasks in sentiment analysis. It concerns the construction of triplets that contain an aspect, its associated sentiment polarity, and an opinion phrase that serves as a rationale for the assigned polarity. Despite the growing popularity of the task and the many machine learning methods being proposed to address it, the number of datasets for ASTE is very limited. In particular, no dataset is available for any of the Slavic languages. In this paper, we present two new datasets for ASTE containing customer opinions about hotels and purchased products expressed in Polish. We also perform experiments with two ASTE techniques combined with two large language models for Polish to investigate their performance and the difficulty of the assembled datasets. The new datasets are available under a permissive licence and have the same file format as the English datasets, facilitating their use in future research.
Via
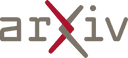
Feb 19, 2025
Abstract:The Fourth Industrial Revolution (4IR) technologies, such as cloud computing, machine learning, and AI, have improved productivity but introduced challenges in workforce training and reskilling. This is critical given existing workforce shortages, especially in marginalized communities like Underrepresented Minorities (URM), who often lack access to quality education. Addressing these challenges, this research presents gAI-PT4I4, a Generative AI-based Personalized Tutor for Industrial 4.0, designed to personalize 4IR experiential learning. gAI-PT4I4 employs sentiment analysis to assess student comprehension, leveraging generative AI and finite automaton to tailor learning experiences. The framework integrates low-fidelity Digital Twins for VR-based training, featuring an Interactive Tutor - a generative AI assistant providing real-time guidance via audio and text. It uses zero-shot sentiment analysis with LLMs and prompt engineering, achieving 86\% accuracy in classifying student-teacher interactions as positive or negative. Additionally, retrieval-augmented generation (RAG) enables personalized learning content grounded in domain-specific knowledge. To adapt training dynamically, finite automaton structures exercises into states of increasing difficulty, requiring 80\% task-performance accuracy for progression. Experimental evaluation with 22 volunteers showed improved accuracy exceeding 80\%, reducing training time. Finally, this paper introduces a Multi-Fidelity Digital Twin model, aligning Digital Twin complexity with Bloom's Taxonomy and Kirkpatrick's model, providing a scalable educational framework.
Via
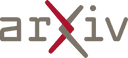
Feb 18, 2025
Abstract:Current Multimodal Sentiment Analysis (MSA) and Emotion Recognition in Conversations (ERC) methods based on pre-trained language models exhibit two primary limitations: 1) Once trained for MSA and ERC tasks, these pre-trained language models lose their original generalized capabilities. 2) They demand considerable computational resources. As the size of pre-trained language models continues to grow, training larger multimodal sentiment analysis models using previous approaches could result in unnecessary computational cost. In response to this challenge, we propose \textbf{M}ultimodal \textbf{S}entiment Analysis and \textbf{E}motion Recognition \textbf{Adapter} (MSE-Adapter), a lightweight and adaptable plugin. This plugin enables a large language model (LLM) to carry out MSA or ERC tasks with minimal computational overhead (only introduces approximately 2.6M to 2.8M trainable parameters upon the 6/7B models), while preserving the intrinsic capabilities of the LLM. In the MSE-Adapter, the Text-Guide-Mixer (TGM) module is introduced to establish explicit connections between non-textual and textual modalities through the Hadamard product. This allows non-textual modalities to better align with textual modalities at the feature level, promoting the generation of higher-quality pseudo tokens. Extensive experiments were conducted on four public English and Chinese datasets using consumer-grade GPUs and open-source LLMs (Qwen-1.8B, ChatGLM3-6B-base, and LLaMA2-7B) as the backbone. The results demonstrate the effectiveness of the proposed plugin. The code will be released on GitHub after a blind review.
Via
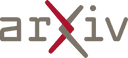