Topic:Information Extraction
What is Information Extraction? Information extraction is the process of automatically extracting structured information from unstructured text data.
Papers and Code
Jun 04, 2025
Abstract:Expressive voice conversion aims to transfer both speaker identity and expressive attributes from a target speech to a given source speech. In this work, we improve over a self-supervised, non-autoregressive framework with a conditional variational autoencoder, focusing on reducing source timbre leakage and improving linguistic-acoustic disentanglement for better style transfer. To minimize style leakage, we use multilingual discrete speech units for content representation and reinforce embeddings with augmentation-based similarity loss and mix-style layer normalization. To enhance expressivity transfer, we incorporate local F0 information via cross-attention and extract style embeddings enriched with global pitch and energy features. Experiments show our model outperforms baselines in emotion and speaker similarity, demonstrating superior style adaptation and reduced source style leakage.
* Accepted to Interspeech 2025
Via
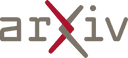
Jun 04, 2025
Abstract:A core motivation of science is to evaluate which scientific model best explains observed data. Bayesian model comparison provides a principled statistical approach to comparing scientific models and has found widespread application within cosmology and astrophysics. Calculating the Bayesian evidence is computationally challenging, especially as we continue to explore increasingly more complex models. The Savage-Dickey density ratio (SDDR) provides a method to calculate the Bayes factor (evidence ratio) between two nested models using only posterior samples from the super model. The SDDR requires the calculation of a normalised marginal distribution over the extra parameters of the super model, which has typically been performed using classical density estimators, such as histograms. Classical density estimators, however, can struggle to scale to high-dimensional settings. We introduce a neural SDDR approach using normalizing flows that can scale to settings where the super model contains a large number of extra parameters. We demonstrate the effectiveness of this neural SDDR methodology applied to both toy and realistic cosmological examples. For a field-level inference setting, we show that Bayes factors computed for a Bayesian hierarchical model (BHM) and simulation-based inference (SBI) approach are consistent, providing further validation that SBI extracts as much cosmological information from the field as the BHM approach. The SDDR estimator with normalizing flows is implemented in the open-source harmonic Python package.
* 9 pages, 1 figure. Submitted to the Open Journal of Astrophysics.
Codes available at https://github.com/astro-informatics/harmonic
Via
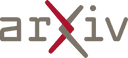
Jun 10, 2025
Abstract:In the era of information explosion, efficiently leveraging large-scale unlabeled data while minimizing the reliance on high-quality pixel-level annotations remains a critical challenge in the field of medical imaging. Semi-supervised learning (SSL) enhances the utilization of unlabeled data by facilitating knowledge transfer, significantly improving the performance of fully supervised models and emerging as a highly promising research direction in medical image analysis. Inspired by the ability of Vision Foundation Models (e.g., SAM-2) to provide rich prior knowledge, we propose SSS (Semi-Supervised SAM-2), a novel approach that leverages SAM-2's robust feature extraction capabilities to uncover latent knowledge in unlabeled medical images, thus effectively enhancing feature support for fully supervised medical image segmentation. Specifically, building upon the single-stream "weak-to-strong" consistency regularization framework, this paper introduces a Discriminative Feature Enhancement (DFE) mechanism to further explore the feature discrepancies introduced by various data augmentation strategies across multiple views. By leveraging feature similarity and dissimilarity across multi-scale augmentation techniques, the method reconstructs and models the features, thereby effectively optimizing the salient regions. Furthermore, a prompt generator is developed that integrates Physical Constraints with a Sliding Window (PCSW) mechanism to generate input prompts for unlabeled data, fulfilling SAM-2's requirement for additional prompts. Extensive experiments demonstrate the superiority of the proposed method for semi-supervised medical image segmentation on two multi-label datasets, i.e., ACDC and BHSD. Notably, SSS achieves an average Dice score of 53.15 on BHSD, surpassing the previous state-of-the-art method by +3.65 Dice. Code will be available at https://github.com/AIGeeksGroup/SSS.
Via
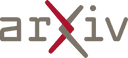
Jun 08, 2025
Abstract:Real-world surveillance often renders faces and license plates unrecognizable in individual low-resolution (LR) frames, hindering reliable identification. To advance temporal recognition models, we present FANVID, a novel video-based benchmark comprising nearly 1,463 LR clips (180 x 320, 20--60 FPS) featuring 63 identities and 49 license plates from three English-speaking countries. Each video includes distractor faces and plates, increasing task difficulty and realism. The dataset contains 31,096 manually verified bounding boxes and labels. FANVID defines two tasks: (1) face matching -- detecting LR faces and matching them to high-resolution mugshots, and (2) license plate recognition -- extracting text from LR plates without a predefined database. Videos are downsampled from high-resolution sources to ensure that faces and text are indecipherable in single frames, requiring models to exploit temporal information. We introduce evaluation metrics adapted from mean Average Precision at IoU > 0.5, prioritizing identity correctness for faces and character-level accuracy for text. A baseline method with pre-trained video super-resolution, detection, and recognition achieved performance scores of 0.58 (face matching) and 0.42 (plate recognition), highlighting both the feasibility and challenge of the tasks. FANVID's selection of faces and plates balances diversity with recognition challenge. We release the software for data access, evaluation, baseline, and annotation to support reproducibility and extension. FANVID aims to catalyze innovation in temporal modeling for LR recognition, with applications in surveillance, forensics, and autonomous vehicles.
Via
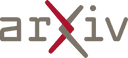
Jun 09, 2025
Abstract:Large Language Models (LLMs) memorize, and thus, among huge amounts of uncontrolled data, may memorize Personally Identifiable Information (PII), which should not be stored and, consequently, not leaked. In this paper, we introduce Private Memorization Editing (PME), an approach for preventing private data leakage that turns an apparent limitation, that is, the LLMs' memorization ability, into a powerful privacy defense strategy. While attacks against LLMs have been performed exploiting previous knowledge regarding their training data, our approach aims to exploit the same kind of knowledge in order to make a model more robust. We detect a memorized PII and then mitigate the memorization of PII by editing a model knowledge of its training data. We verify that our procedure does not affect the underlying language model while making it more robust against privacy Training Data Extraction attacks. We demonstrate that PME can effectively reduce the number of leaked PII in a number of configurations, in some cases even reducing the accuracy of the privacy attacks to zero.
* To be published at ACL 2025 (Main)
Via
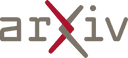
Jun 09, 2025
Abstract:With the rapid development of digital pathology, virtual staining has become a key technology in multimedia medical information systems, offering new possibilities for the analysis and diagnosis of pathological images. However, existing H&E-to-IHC studies often overlook the cross-channel correlations between cell nuclei and cell membranes. To address this issue, we propose a novel Cross-Channel Perception Learning (CCPL) strategy. Specifically, CCPL first decomposes HER2 immunohistochemical staining into Hematoxylin and DAB staining channels, corresponding to cell nuclei and cell membranes, respectively. Using the pathology foundation model Gigapath's Tile Encoder, CCPL extracts dual-channel features from both the generated and real images and measures cross-channel correlations between nuclei and membranes. The features of the generated and real stained images, obtained through the Tile Encoder, are also used to calculate feature distillation loss, enhancing the model's feature extraction capabilities without increasing the inference burden. Additionally, CCPL performs statistical analysis on the focal optical density maps of both single channels to ensure consistency in staining distribution and intensity. Experimental results, based on quantitative metrics such as PSNR, SSIM, PCC, and FID, along with professional evaluations from pathologists, demonstrate that CCPL effectively preserves pathological features, generates high-quality virtual stained images, and provides robust support for automated pathological diagnosis using multimedia medical data.
Via
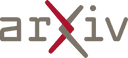
Jun 05, 2025
Abstract:Heterogeneous multi-robot systems show great potential in complex tasks requiring coordinated hybrid cooperation. However, traditional approaches relying on static models often struggle with task diversity and dynamic environments. This highlights the need for generalizable intelligence that can bridge high-level reasoning with low-level execution across heterogeneous agents. To address this, we propose a hierarchical framework integrating a prompted Large Language Model (LLM) and a GridMask-enhanced fine-tuned Vision Language Model (VLM). The LLM performs task decomposition and global semantic map construction, while the VLM extracts task-specified semantic labels and 2D spatial information from aerial images to support local planning. Within this framework, the aerial robot follows a globally optimized semantic path and continuously provides bird-view images, guiding the ground robot's local semantic navigation and manipulation, including target-absent scenarios where implicit alignment is maintained. Experiments on a real-world letter-cubes arrangement task demonstrate the framework's adaptability and robustness in dynamic environments. To the best of our knowledge, this is the first demonstration of an aerial-ground heterogeneous system integrating VLM-based perception with LLM-driven task reasoning and motion planning.
Via
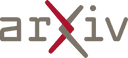
Jun 09, 2025
Abstract:With the rapid development of radar jamming systems, especially digital radio frequency memory (DRFM), the electromagnetic environment has become increasingly complex. In recent years, most existing studies have focused solely on either jamming recognition or anti-jamming strategy design. In this paper, we propose a unified framework that integrates interference recognition with intelligent anti-jamming strategy selection. Specifically, time-frequency (TF) features of radar echoes are first extracted using both Short-Time Fourier Transform (STFT) and Smoothed Pseudo Wigner-Ville Distribution (SPWVD). A feature fusion method is then designed to effectively combine these two types of time-frequency representations. The fused TF features are further combined with time-domain features of the radar echoes through a cross-modal fusion module based on an attention mechanism. Finally, the recognition results, together with information obtained from the passive radar, are fed into a Deep Q-Network (DQN)-based intelligent anti-jamming strategy network to select jamming suppression waveforms. The key jamming parameters obtained by the passive radar provide essential information for intelligent decision-making, enabling the generation of more effective strategies tailored to specific jamming types. The proposed method demonstrates improvements in both jamming type recognition accuracy and the stability of anti-jamming strategy selection under complex environments. Experimental results show that our method achieves superior performance compared to Support Vector Machines (SVM), VGG-16, and 2D-CNN methods, with respective improvements of 1.41%, 2.5%, and 14.51% in overall accuracy. Moreover, in comparison with the SARSA algorithm, the designed algorithm achieves faster reward convergence and more stable strategy generation.
Via
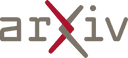
Jun 04, 2025
Abstract:In the fifth-generation communication system (5G), multipath-assisted positioning (MAP) has emerged as a promising approach. With the enhancement of signal resolution, multipath component (MPC) are no longer regarded as noise but rather as valuable information that can contribute to positioning. However, existing research often treats reflective surfaces as ideal reflectors, while being powerless in the face of indistinguishable multipath caused by diffuse reflectors. This study approaches diffuse reflectors from the perspective of uncertainty, investigating the statistical distribution characteristics of indoor diffuse and specular reflectors. Based on these insights, a task-guided disentangled representation learning method leveraging multi-time channel impulse response (CIR) observations is designed to directly map CIRs to positions, while mitigating the adverse effects of components that contribute minimally to localization accuracy (e.g., diffuse multipath).In this semi-supervised learning framework, a global feature extraction architecture based on self-attention is proposed to capture location-independent wireless environmental information, while an MLP is employed to extract the time-varying features related to user equipment (UE) positions. Variational inference based on a latent variable model (LVM) is applied to separate independent features within the CIR, with position labels guiding the LVM to express components more beneficial for localization. Additionally, we provide a feasibility proof for the separability of diffuse and specular environmental features in CIRs. Simulation results demonstrate that the proposed method achieves higher localization accuracy compared to conventional search-based localization methods, with enhanced robustness against indistinguishable multipath from diffuse reflectors.
Via
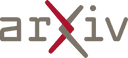
Jun 05, 2025
Abstract:With the widespread adoption of Large Language Models (LLMs), prompt injection attacks have emerged as a significant security threat. Existing defense mechanisms often face critical trade-offs between effectiveness and generalizability. This highlights the urgent need for efficient prompt injection detection methods that are applicable across a wide range of LLMs. To address this challenge, we propose DMPI-PMHFE, a dual-channel feature fusion detection framework. It integrates a pretrained language model with heuristic feature engineering to detect prompt injection attacks. Specifically, the framework employs DeBERTa-v3-base as a feature extractor to transform input text into semantic vectors enriched with contextual information. In parallel, we design heuristic rules based on known attack patterns to extract explicit structural features commonly observed in attacks. Features from both channels are subsequently fused and passed through a fully connected neural network to produce the final prediction. This dual-channel approach mitigates the limitations of relying only on DeBERTa to extract features. Experimental results on diverse benchmark datasets demonstrate that DMPI-PMHFE outperforms existing methods in terms of accuracy, recall, and F1-score. Furthermore, when deployed actually, it significantly reduces attack success rates across mainstream LLMs, including GLM-4, LLaMA 3, Qwen 2.5, and GPT-4o.
* Accepted by KSEM2025 AI & Sec Workshop
Via
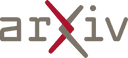