Jul 09, 2025
Abstract:We present 4KAgent, a unified agentic super-resolution generalist system designed to universally upscale any image to 4K resolution (and even higher, if applied iteratively). Our system can transform images from extremely low resolutions with severe degradations, for example, highly distorted inputs at 256x256, into crystal-clear, photorealistic 4K outputs. 4KAgent comprises three core components: (1) Profiling, a module that customizes the 4KAgent pipeline based on bespoke use cases; (2) A Perception Agent, which leverages vision-language models alongside image quality assessment experts to analyze the input image and make a tailored restoration plan; and (3) A Restoration Agent, which executes the plan, following a recursive execution-reflection paradigm, guided by a quality-driven mixture-of-expert policy to select the optimal output for each step. Additionally, 4KAgent embeds a specialized face restoration pipeline, significantly enhancing facial details in portrait and selfie photos. We rigorously evaluate our 4KAgent across 11 distinct task categories encompassing a total of 26 diverse benchmarks, setting new state-of-the-art on a broad spectrum of imaging domains. Our evaluations cover natural images, portrait photos, AI-generated content, satellite imagery, fluorescence microscopy, and medical imaging like fundoscopy, ultrasound, and X-ray, demonstrating superior performance in terms of both perceptual (e.g., NIQE, MUSIQ) and fidelity (e.g., PSNR) metrics. By establishing a novel agentic paradigm for low-level vision tasks, we aim to catalyze broader interest and innovation within vision-centric autonomous agents across diverse research communities. We will release all the code, models, and results at: https://4kagent.github.io.
Via
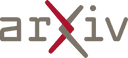