What is Music Generation? Music generation is the task of generating music or music-like sounds from a model or algorithm.
Papers and Code
Jun 26, 2025
Abstract:Singing voice synthesis (SVS) aims to generate expressive and high-quality vocals from musical scores, requiring precise modeling of pitch, duration, and articulation. While diffusion-based models have achieved remarkable success in image and video generation, their application to SVS remains challenging due to the complex acoustic and musical characteristics of singing, often resulting in artifacts that degrade naturalness. In this work, we propose SmoothSinger, a conditional diffusion model designed to synthesize high quality and natural singing voices. Unlike prior methods that depend on vocoders as a final stage and often introduce distortion, SmoothSinger refines low-quality synthesized audio directly in a unified framework, mitigating the degradation associated with two-stage pipelines. The model adopts a reference-guided dual-branch architecture, using low-quality audio from any baseline system as a reference to guide the denoising process, enabling more expressive and context-aware synthesis. Furthermore, it enhances the conventional U-Net with a parallel low-frequency upsampling path, allowing the model to better capture pitch contours and long term spectral dependencies. To improve alignment during training, we replace reference audio with degraded ground truth audio, addressing temporal mismatch between reference and target signals. Experiments on the Opencpop dataset, a large-scale Chinese singing corpus, demonstrate that SmoothSinger achieves state-of-the-art results in both objective and subjective evaluations. Extensive ablation studies confirm its effectiveness in reducing artifacts and improving the naturalness of synthesized voices.
Via
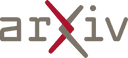
Jun 23, 2025
Abstract:Recent advancements have brought generated music closer to human-created compositions, yet evaluating these models remains challenging. While human preference is the gold standard for assessing quality, translating these subjective judgments into objective metrics, particularly for text-audio alignment and music quality, has proven difficult. In this work, we generate 6k songs using 12 state-of-the-art models and conduct a survey of 15k pairwise audio comparisons with 2.5k human participants to evaluate the correlation between human preferences and widely used metrics. To the best of our knowledge, this work is the first to rank current state-of-the-art music generation models and metrics based on human preference. To further the field of subjective metric evaluation, we provide open access to our dataset of generated music and human evaluations.
* In Proceedings of the IEEE International Conference on Acoustics,
Speech and Signal Processing (ICASSP), 2025
* Accepted at ICASSP 2025
Via
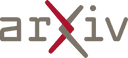
Jun 23, 2025
Abstract:Aligning the rhythm of visual motion in a video with a given music track is a practical need in multimedia production, yet remains an underexplored task in autonomous video editing. Effective alignment between motion and musical beats enhances viewer engagement and visual appeal, particularly in music videos, promotional content, and cinematic editing. Existing methods typically depend on labor-intensive manual cutting, speed adjustments, or heuristic-based editing techniques to achieve synchronization. While some generative models handle joint video and music generation, they often entangle the two modalities, limiting flexibility in aligning video to music beats while preserving the full visual content. In this paper, we propose a novel and efficient framework, termed MVAA (Music-Video Auto-Alignment), that automatically edits video to align with the rhythm of a given music track while preserving the original visual content. To enhance flexibility, we modularize the task into a two-step process in our MVAA: aligning motion keyframes with audio beats, followed by rhythm-aware video inpainting. Specifically, we first insert keyframes at timestamps aligned with musical beats, then use a frame-conditioned diffusion model to generate coherent intermediate frames, preserving the original video's semantic content. Since comprehensive test-time training can be time-consuming, we adopt a two-stage strategy: pretraining the inpainting module on a small video set to learn general motion priors, followed by rapid inference-time fine-tuning for video-specific adaptation. This hybrid approach enables adaptation within 10 minutes with one epoch on a single NVIDIA 4090 GPU using CogVideoX-5b-I2V as the backbone. Extensive experiments show that our approach can achieve high-quality beat alignment and visual smoothness.
Via
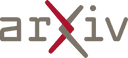
Jun 23, 2025
Abstract:We propose Legato, a new end-to-end transformer model for optical music recognition (OMR). Legato is the first large-scale pretrained OMR model capable of recognizing full-page or multi-page typeset music scores and the first to generate documents in ABC notation, a concise, human-readable format for symbolic music. Bringing together a pretrained vision encoder with an ABC decoder trained on a dataset of more than 214K images, our model exhibits the strong ability to generalize across various typeset scores. We conduct experiments on a range of datasets and demonstrate that our model achieves state-of-the-art performance. Given the lack of a standardized evaluation for end-to-end OMR, we comprehensively compare our model against the previous state of the art using a diverse set of metrics.
Via
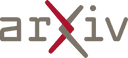
Jun 24, 2025
Abstract:We propose Kling-Foley, a large-scale multimodal Video-to-Audio generation model that synthesizes high-quality audio synchronized with video content. In Kling-Foley, we introduce multimodal diffusion transformers to model the interactions between video, audio, and text modalities, and combine it with a visual semantic representation module and an audio-visual synchronization module to enhance alignment capabilities. Specifically, these modules align video conditions with latent audio elements at the frame level, thereby improving semantic alignment and audio-visual synchronization. Together with text conditions, this integrated approach enables precise generation of video-matching sound effects. In addition, we propose a universal latent audio codec that can achieve high-quality modeling in various scenarios such as sound effects, speech, singing, and music. We employ a stereo rendering method that imbues synthesized audio with a spatial presence. At the same time, in order to make up for the incomplete types and annotations of the open-source benchmark, we also open-source an industrial-level benchmark Kling-Audio-Eval. Our experiments show that Kling-Foley trained with the flow matching objective achieves new audio-visual SOTA performance among public models in terms of distribution matching, semantic alignment, temporal alignment and audio quality.
Via
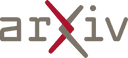
Jun 17, 2025
Abstract:We present Sleeping-DISCO 9M, a large-scale pre-training dataset for music and song. To the best of our knowledge, there are no open-source high-quality dataset representing popular and well-known songs for generative music modeling tasks such as text-music, music-captioning, singing-voice synthesis, melody reconstruction and cross-model retrieval. Past contributions focused on isolated and constrained factors whose core perspective was to create synthetic or re-recorded music corpus (e.g. GTSinger, M4Singer) and arbitrarily large-scale audio datasets (e.g. DISCO-10M and LAIONDISCO-12M) had been another focus for the community. Unfortunately, adoption of these datasets has been below substantial in the generative music community as these datasets fail to reflect real-world music and its flavour. Our dataset changes this narrative and provides a dataset that is constructed using actual popular music and world-renowned artists.
Via
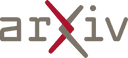
Jun 18, 2025
Abstract:Breakthroughs in text-to-music generation models are transforming the creative landscape, equipping musicians with innovative tools for composition and experimentation like never before. However, controlling the generation process to achieve a specific desired outcome remains a significant challenge. Even a minor change in the text prompt, combined with the same random seed, can drastically alter the generated piece. In this paper, we explore the application of existing text-to-music diffusion models for instrument editing. Specifically, for an existing audio track, we aim to leverage a pretrained text-to-music diffusion model to edit the instrument while preserving the underlying content. Based on the insight that the model first focuses on the overall structure or content of the audio, then adds instrument information, and finally refines the quality, we show that selecting a well-chosen intermediate timestep, identified through an instrument classifier, yields a balance between preserving the original piece's content and achieving the desired timbre. Our method does not require additional training of the text-to-music diffusion model, nor does it compromise the generation process's speed.
Via
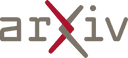
Jun 18, 2025
Abstract:Detailed captions that accurately reflect the characteristics of a music piece can enrich music databases and drive forward research in music AI. This paper introduces a multi-task music captioning model, SonicVerse, that integrates caption generation with auxiliary music feature detection tasks such as key detection, vocals detection, and more, so as to directly capture both low-level acoustic details as well as high-level musical attributes. The key contribution is a projection-based architecture that transforms audio input into language tokens, while simultaneously detecting music features through dedicated auxiliary heads. The outputs of these heads are also projected into language tokens, to enhance the captioning input. This framework not only produces rich, descriptive captions for short music fragments but also directly enables the generation of detailed time-informed descriptions for longer music pieces, by chaining the outputs using a large-language model. To train the model, we extended the MusicBench dataset by annotating it with music features using MIRFLEX, a modular music feature extractor, resulting in paired audio, captions and music feature data. Experimental results show that incorporating features in this way improves the quality and detail of the generated captions.
* Proceedings of the 6th Conference on AI Music Creativity (AIMC
2025), Brussels, Belgium, September 10th - 12th, 2025
* 14 pages, 2 figures, Accepted to AIMC 2025
Via
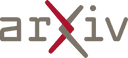
Jun 17, 2025
Abstract:Jamming requires coordination, anticipation, and collaborative creativity between musicians. Current generative models of music produce expressive output but are not able to generate in an \emph{online} manner, meaning simultaneously with other musicians (human or otherwise). We propose ReaLchords, an online generative model for improvising chord accompaniment to user melody. We start with an online model pretrained by maximum likelihood, and use reinforcement learning to finetune the model for online use. The finetuning objective leverages both a novel reward model that provides feedback on both harmonic and temporal coherency between melody and chord, and a divergence term that implements a novel type of distillation from a teacher model that can see the future melody. Through quantitative experiments and listening tests, we demonstrate that the resulting model adapts well to unfamiliar input and produce fitting accompaniment. ReaLchords opens the door to live jamming, as well as simultaneous co-creation in other modalities.
* Accepted by ICML 2024
Via
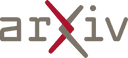
Jun 16, 2025
Abstract:Existing work in automatic music generation has primarily focused on end-to-end systems that produce complete compositions or continuations. However, because musical composition is typically an iterative process, such systems make it difficult to engage in the back-and-forth between human and machine that is essential to computer-assisted creativity. In this study, we address the task of personalizable, multi-track, long-context, and controllable symbolic music infilling to enhance the process of computer-assisted composition. We present MIDI-RWKV, a novel model based on the RWKV-7 linear architecture, to enable efficient and coherent musical cocreation on edge devices. We also demonstrate that MIDI-RWKV admits an effective method of finetuning its initial state for personalization in the very-low-sample regime. We evaluate MIDI-RWKV and its state tuning on several quantitative and qualitative metrics, and release model weights and code at https://github.com/christianazinn/MIDI-RWKV.
Via
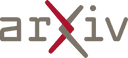