Jun 09, 2025
Abstract:Audio is inherently temporal and closely synchronized with the visual world, making it a naturally aligned and expressive control signal for controllable video generation (e.g., movies). Beyond control, directly translating audio into video is essential for understanding and visualizing rich audio narratives (e.g., Podcasts or historical recordings). However, existing approaches fall short in generating high-quality videos with precise audio-visual synchronization, especially across diverse and complex audio types. In this work, we introduce MTV, a versatile framework for audio-sync video generation. MTV explicitly separates audios into speech, effects, and music tracks, enabling disentangled control over lip motion, event timing, and visual mood, respectively -- resulting in fine-grained and semantically aligned video generation. To support the framework, we additionally present DEMIX, a dataset comprising high-quality cinematic videos and demixed audio tracks. DEMIX is structured into five overlapped subsets, enabling scalable multi-stage training for diverse generation scenarios. Extensive experiments demonstrate that MTV achieves state-of-the-art performance across six standard metrics spanning video quality, text-video consistency, and audio-video alignment. Project page: https://hjzheng.net/projects/MTV/.
Via
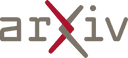