Jun 11, 2025
Abstract:Federated Learning (FL) provides a privacy-preserving paradigm for training audio classification (AC) models across distributed clients without sharing raw data. However, Federated Audio Classification (FedAC) faces three critical challenges that substantially hinder performance: data heterogeneity, model heterogeneity, and data poisoning. While prior works have attempted to address these issues, they are typically treated independently, lacking a unified and robust solution suited to real-world federated audio scenarios. To bridge this gap, we propose FedMLAC, a unified mutual learning framework designed to simultaneously tackle these challenges in FedAC. Specifically, FedMLAC introduces a dual-model architecture on each client, comprising a personalized local AC model and a lightweight, globally shared Plug-in model. Through bidirectional knowledge distillation, the Plug-in model enables global knowledge transfer while adapting to client-specific data distributions, thus supporting both generalization and personalization. To further enhance robustness against corrupted audio data, we develop a Layer-wise Pruning Aggregation (LPA) strategy that filters unreliable Plug-in model updates based on parameter deviations during server-side aggregation. Extensive experiments on four diverse audio classification benchmarks, spanning both speech and non-speech tasks, demonstrate that FedMLAC consistently outperforms existing state-of-the-art methods in terms of classification accuracy and robustness to noisy data.
* initial version
Via
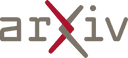