What is speech recognition? Speech recognition is the task of identifying words spoken aloud, analyzing the voice and language, and accurately transcribing the words.
Papers and Code
May 16, 2025
Abstract:Automatic Speech Recognition (ASR) technologies have transformed human-computer interaction; however, low-resource languages in Africa remain significantly underrepresented in both research and practical applications. This study investigates the major challenges hindering the development of ASR systems for these languages, which include data scarcity, linguistic complexity, limited computational resources, acoustic variability, and ethical concerns surrounding bias and privacy. The primary goal is to critically analyze these barriers and identify practical, inclusive strategies to advance ASR technologies within the African context. Recent advances and case studies emphasize promising strategies such as community-driven data collection, self-supervised and multilingual learning, lightweight model architectures, and techniques that prioritize privacy. Evidence from pilot projects involving various African languages showcases the feasibility and impact of customized solutions, which encompass morpheme-based modeling and domain-specific ASR applications in sectors like healthcare and education. The findings highlight the importance of interdisciplinary collaboration and sustained investment to tackle the distinct linguistic and infrastructural challenges faced by the continent. This study offers a progressive roadmap for creating ethical, efficient, and inclusive ASR systems that not only safeguard linguistic diversity but also improve digital accessibility and promote socioeconomic participation for speakers of African languages.
Via
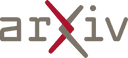
May 25, 2025
Abstract:This study presents FiLLM, a Filipino-optimized large language model, designed to enhance natural language processing (NLP) capabilities in the Filipino language. Built upon the SeaLLM-7B 2.5 model, FiLLM leverages Low-Rank Adaptation (LoRA) fine-tuning to optimize memory efficiency while maintaining task-specific performance. The model was trained and evaluated on diverse Filipino datasets to address key NLP tasks, including Named Entity Recognition (NER), Part-of-Speech (POS) tagging, Dependency Parsing, and Text Summarization. Performance comparisons with the CalamanCy model were conducted using F1 Score, Precision, Recall, Compression Rate, and Keyword Overlap metrics. Results indicate that Calamancy outperforms FILLM in several aspects, demonstrating its effectiveness in processing Filipino text with improved linguistic comprehension and adaptability. This research contributes to the advancement of Filipino NLP applications by providing an optimized, efficient, and scalable language model tailored for local linguistic needs.
Via
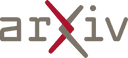
May 20, 2025
Abstract:Deep neural networks have achieved state-of-the-art results in a wide range of applications, from natural language processing and computer vision to speech recognition. However, as tasks become increasingly complex, model sizes continue to grow, posing challenges in latency and memory efficiency. To meet these constraints, post-training quantization has emerged as a promising solution. In this paper, we propose a novel hardware-efficient quantization and inference scheme that exploits hardware advantages with minimal accuracy degradation. Specifically, we introduce a W4A8 scheme, where weights are quantized and stored using 4-bit integer precision, and inference computations are performed using 8-bit floating-point arithmetic, demonstrating significant speedups and improved memory utilization compared to 16-bit operations, applicable on various modern accelerators. To mitigate accuracy loss, we develop a novel quantization algorithm, dubbed Dual Precision Quantization (DPQ), that leverages the unique structure of our scheme without introducing additional inference overhead. Experimental results demonstrate improved performance (i.e., increased throughput) while maintaining tolerable accuracy degradation relative to the full-precision model.
* Accepted at eLVM Workshop, CVPR, 2025
Via
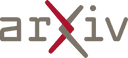
May 20, 2025
Abstract:We introduce Vox-Profile, a comprehensive benchmark to characterize rich speaker and speech traits using speech foundation models. Unlike existing works that focus on a single dimension of speaker traits, Vox-Profile provides holistic and multi-dimensional profiles that reflect both static speaker traits (e.g., age, sex, accent) and dynamic speech properties (e.g., emotion, speech flow). This benchmark is grounded in speech science and linguistics, developed with domain experts to accurately index speaker and speech characteristics. We report benchmark experiments using over 15 publicly available speech datasets and several widely used speech foundation models that target various static and dynamic speaker and speech properties. In addition to benchmark experiments, we showcase several downstream applications supported by Vox-Profile. First, we show that Vox-Profile can augment existing speech recognition datasets to analyze ASR performance variability. Vox-Profile is also used as a tool to evaluate the performance of speech generation systems. Finally, we assess the quality of our automated profiles through comparison with human evaluation and show convergent validity. Vox-Profile is publicly available at: https://github.com/tiantiaf0627/vox-profile-release.
Via
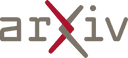
May 19, 2025
Abstract:OpenAI's Whisper has achieved significant success in Automatic Speech Recognition. However, it has consistently been found to exhibit hallucination issues, particularly in non-speech segments, which limits its broader application in complex industrial settings. In this paper, we introduce a novel method to reduce Whisper's hallucination on non-speech segments without using any pre- or post-possessing techniques. Specifically, we benchmark the contribution of each self-attentional head in the Whisper-large-v3 decoder to the hallucination problem by performing a head-wise mask. Our findings reveal that only 3 of the 20 heads account for over 75% of the hallucinations on the UrbanSound dataset. We then fine-tune these three crazy heads using a collection of non-speech data. The results show that our best fine-tuned model, namely Calm-Whisper, achieves over 80% reduction in non-speech hallucination with only less than 0.1% WER degradation on LibriSpeech test-clean and test-other.
* Accepted to Interspeech 2025
Via
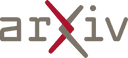
May 19, 2025
Abstract:The scope of the International Workshop on Spoken Language Translation (IWSLT) has recently broadened beyond traditional Speech Translation (ST) to encompass a wider array of tasks, including Speech Question Answering and Summarization. This shift is partly driven by the growing capabilities of modern systems, particularly with the success of Large Language Models (LLMs). In this paper, we present the Karlsruhe Institute of Technology's submissions for the Offline ST and Instruction Following (IF) tracks, where we leverage LLMs to enhance performance across all tasks. For the Offline ST track, we propose a pipeline that employs multiple automatic speech recognition systems, whose outputs are fused using an LLM with document-level context. This is followed by a two-step translation process, incorporating additional refinement step to improve translation quality. For the IF track, we develop an end-to-end model that integrates a speech encoder with an LLM to perform a wide range of instruction-following tasks. We complement it with a final document-level refinement stage to further enhance output quality by using contextual information.
Via
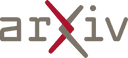
May 19, 2025
Abstract:Transferring linguistic knowledge from a pretrained language model (PLM) to acoustic feature learning has proven effective in enhancing end-to-end automatic speech recognition (E2E-ASR). However, aligning representations between linguistic and acoustic modalities remains a challenge due to inherent modality gaps. Optimal transport (OT) has shown promise in mitigating these gaps by minimizing the Wasserstein distance (WD) between linguistic and acoustic feature distributions. However, previous OT-based methods overlook structural relationships, treating feature vectors as unordered sets. To address this, we propose Graph Matching Optimal Transport (GM-OT), which models linguistic and acoustic sequences as structured graphs. Nodes represent feature embeddings, while edges capture temporal and sequential relationships. GM-OT minimizes both WD (between nodes) and Gromov-Wasserstein distance (GWD) (between edges), leading to a fused Gromov-Wasserstein distance (FGWD) formulation. This enables structured alignment and more efficient knowledge transfer compared to existing OT-based approaches. Theoretical analysis further shows that prior OT-based methods in linguistic knowledge transfer can be viewed as a special case within our GM-OT framework. We evaluate GM-OT on Mandarin ASR using a CTC-based E2E-ASR system with a PLM for knowledge transfer. Experimental results demonstrate significant performance gains over state-of-the-art models, validating the effectiveness of our approach.
* To appear in Interspeech 2025
Via
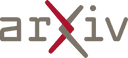
May 16, 2025
Abstract:This paper presents CAMEO -- a curated collection of multilingual emotional speech datasets designed to facilitate research in emotion recognition and other speech-related tasks. The main objectives were to ensure easy access to the data, to allow reproducibility of the results, and to provide a standardized benchmark for evaluating speech emotion recognition (SER) systems across different emotional states and languages. The paper describes the dataset selection criteria, the curation and normalization process, and provides performance results for several models. The collection, along with metadata, and a leaderboard, is publicly available via the Hugging Face platform.
* Under review at NeurIPS
Via
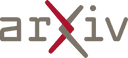
May 15, 2025
Abstract:The integration of AI speech recognition technologies into healthcare has the potential to revolutionize clinical workflows and patient-provider communication. However, this study reveals significant gaps in inclusivity, with datasets and research disproportionately favouring high-resource languages, standardized accents, and narrow demographic groups. These biases risk perpetuating healthcare disparities, as AI systems may misinterpret speech from marginalized groups. This paper highlights the urgent need for inclusive dataset design, bias mitigation research, and policy frameworks to ensure equitable access to AI speech technologies in healthcare.
Via
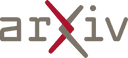
May 22, 2025
Abstract:In this paper, we combine two-step knowledge distillation, structured pruning, truncation, and vocabulary trimming for extremely compressing multilingual encoder-only language models for low-resource languages. Our novel approach systematically combines existing techniques and takes them to the extreme, reducing layer depth, feed-forward hidden size, and intermediate layer embedding size to create significantly smaller monolingual models while retaining essential language-specific knowledge. We achieve compression rates of up to 92% with only a marginal performance drop of 2-10% in four downstream tasks, including sentiment analysis, topic classification, named entity recognition, and part-of-speech tagging, across three low-resource languages. Notably, the performance degradation correlates with the amount of language-specific data in the teacher model, with larger datasets resulting in smaller performance losses. Additionally, we conduct extensive ablation studies to identify best practices for multilingual model compression using these techniques.
* Pre-print
Via
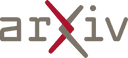