Mar 10, 2025
Abstract:Vision-Language-Action (VLA) models excel at robotic tasks by leveraging large-scale 2D vision-language pretraining, but their reliance on RGB images limits spatial reasoning critical for real-world interaction. Retraining these models with 3D data is computationally prohibitive, while discarding existing 2D datasets wastes valuable resources. To bridge this gap, we propose PointVLA, a framework that enhances pre-trained VLAs with point cloud inputs without requiring retraining. Our method freezes the vanilla action expert and injects 3D features via a lightweight modular block. To identify the most effective way of integrating point cloud representations, we conduct a skip-block analysis to pinpoint less useful blocks in the vanilla action expert, ensuring that 3D features are injected only into these blocks--minimizing disruption to pre-trained representations. Extensive experiments demonstrate that PointVLA outperforms state-of-the-art 2D imitation learning methods, such as OpenVLA, Diffusion Policy and DexVLA, across both simulated and real-world robotic tasks. Specifically, we highlight several key advantages of PointVLA enabled by point cloud integration: (1) Few-shot multi-tasking, where PointVLA successfully performs four different tasks using only 20 demonstrations each; (2) Real-vs-photo discrimination, where PointVLA distinguishes real objects from their images, leveraging 3D world knowledge to improve safety and reliability; (3) Height adaptability, Unlike conventional 2D imitation learning methods, PointVLA enables robots to adapt to objects at varying table height that unseen in train data. Furthermore, PointVLA achieves strong performance in long-horizon tasks, such as picking and packing objects from a moving conveyor belt, showcasing its ability to generalize across complex, dynamic environments.
Via
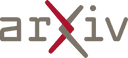