May 09, 2025
Abstract:Retouching is an essential task in post-manipulation of raw photographs. Generative editing, guided by text or strokes, provides a new tool accessible to users but can easily change the identity of the original objects in unacceptable and unpredictable ways. In contrast, although traditional procedural edits, as commonly supported by photoediting tools (e.g., Gimp, Lightroom), are conservative, they are still preferred by professionals. Unfortunately, professional quality retouching involves many individual procedural editing operations that is challenging to plan for most novices. In this paper, we ask if a multimodal large language model (MLLM) can be taught to critique raw photographs, suggest suitable remedies, and finally realize them with a given set of pre-authored procedural image operations. We demonstrate that MLLMs can be first made aware of the underlying image processing operations, by training them to solve specially designed visual puzzles. Subsequently, such an operation-aware MLLM can both plan and propose edit sequences. To facilitate training, given a set of expert-edited photos, we synthesize a reasoning dataset by procedurally manipulating the expert edits and then grounding a pretrained LLM on the visual adjustments, to synthesize reasoning for finetuning. The proposed retouching operations are, by construction, understandable by the users, preserve object details and resolution, and can be optionally overridden. We evaluate our setup on a variety of test examples and show advantages, in terms of explainability and identity preservation, over existing generative and other procedural alternatives. Code, data, models, and supplementary results can be found via our project website at https://monetgpt.github.io.
* Accepted at SIGGRAPH 2025 [ACM Transactions on Graphics]; Project
website: https://monetgpt.github.io
Via
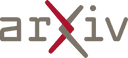