What is Topic Modeling? Topic modeling is a type of statistical modeling for discovering the abstract topics that occur in a collection of documents.
Papers and Code
May 29, 2025
Abstract:Conversational agents powered by large language models (LLMs) are rapidly becoming integral to our daily interactions, generating unprecedented amounts of conversational data. Such datasets offer a powerful lens into societal interests, trending topics, and collective concerns. Yet, existing approaches typically treat these interactions as independent and miss critical insights that could emerge from aggregating and reasoning across large-scale conversation logs. In this paper, we introduce Aggregative Question Answering, a novel task requiring models to reason explicitly over thousands of user-chatbot interactions to answer aggregative queries, such as identifying emerging concerns among specific demographics. To enable research in this direction, we construct a benchmark, WildChat-AQA, comprising 6,027 aggregative questions derived from 182,330 real-world chatbot conversations. Experiments show that existing methods either struggle to reason effectively or incur prohibitive computational costs, underscoring the need for new approaches capable of extracting collective insights from large-scale conversational data.
Via
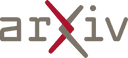
May 30, 2025
Abstract:The scientific literature is growing rapidly, making it hard to keep track of the state-of-the-art. Systematic literature reviews (SLRs) aim to identify and evaluate all relevant papers on a topic. After retrieving a set of candidate papers, the abstract screening phase determines initial relevance. To date, abstract screening methods using large language models (LLMs) focus on binary classification settings; existing question answering (QA) based ranking approaches suffer from error propagation. LLMs offer a unique opportunity to evaluate the SLR's inclusion and exclusion criteria, yet, existing benchmarks do not provide them exhaustively. We manually extract these criteria as well as research questions for 57 SLRs, mostly in the medical domain, enabling principled comparisons between approaches. Moreover, we propose LGAR, a zero-shot LLM Guided Abstract Ranker composed of an LLM based graded relevance scorer and a dense re-ranker. Our extensive experiments show that LGAR outperforms existing QA-based methods by 5-10 pp. in mean average precision. Our code and data is publicly available.
Via
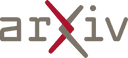
May 29, 2025
Abstract:Large Language Models (LLMs) excel in translation among other things, demonstrating competitive performance for many language pairs in zero- and few-shot settings. But unlike dedicated neural machine translation models, LLMs are not trained on any translation-related objective. What explains their remarkable translation abilities? Are these abilities grounded in "incidental bilingualism" (Briakou et al. 2023) in training data? Does instruction tuning contribute to it? Are LLMs capable of aligning and leveraging semantically identical or similar monolingual contents from different corners of the internet that are unlikely to fit in a single context window? I offer some reflections on this topic, informed by recent studies and growing user experience. My working hypothesis is that LLMs' translation abilities originate in two different types of pre-training data that may be internalized by the models in different ways. I discuss the prospects for testing the "duality" hypothesis empirically and its implications for reconceptualizing translation, human and machine, in the age of deep learning.
* 4 figures
Via
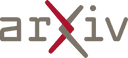
May 13, 2025
Abstract:Topic modeling in Italian legal research is hindered by the lack of public datasets, limiting the analysis of legal themes in Supreme Court judgments. To address this, we developed a document processing pipeline that produces an anonymized dataset optimized for topic modeling. The pipeline integrates document layout analysis (YOLOv8x), optical character recognition, and text anonymization. The DLA module achieved a mAP@50 of 0.964 and a mAP@50-95 of 0.800. The OCR detector reached a mAP@50-95 of 0.9022, and the text recognizer (TrOCR) obtained a character error rate of 0.0047 and a word error rate of 0.0248. Compared to OCR-only methods, our dataset improved topic modeling with a diversity score of 0.6198 and a coherence score of 0.6638. We applied BERTopic to extract topics and used large language models to generate labels and summaries. Outputs were evaluated against domain expert interpretations. Claude Sonnet 3.7 achieved a BERTScore F1 of 0.8119 for labeling and 0.9130 for summarization.
* 51 pages
Via
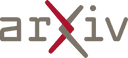
May 26, 2025
Abstract:Zero-shot streaming text-to-speech is an important research topic in human-computer interaction. Existing methods primarily use a lookahead mechanism, relying on future text to achieve natural streaming speech synthesis, which introduces high processing latency. To address this issue, we propose SMLLE, a streaming framework for generating high-quality speech frame-by-frame. SMLLE employs a Transducer to convert text into semantic tokens in real time while simultaneously obtaining duration alignment information. The combined outputs are then fed into a fully autoregressive (AR) streaming model to reconstruct mel-spectrograms. To further stabilize the generation process, we design a Delete < Bos > Mechanism that allows the AR model to access future text introducing as minimal delay as possible. Experimental results suggest that the SMLLE outperforms current streaming TTS methods and achieves comparable performance over sentence-level TTS systems. Samples are available on https://anonymous.4open.science/w/demo_page-48B7/.
Via
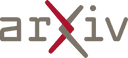
May 30, 2025
Abstract:Semantic Text Embedding is a fundamental NLP task that encodes textual content into vector representations, where proximity in the embedding space reflects semantic similarity. While existing embedding models excel at capturing general meaning, they often overlook ideological nuances, limiting their effectiveness in tasks that require an understanding of political bias. To address this gap, we introduce PRISM, the first framework designed to Produce inteRpretable polItical biaS eMbeddings. PRISM operates in two key stages: (1) Controversial Topic Bias Indicator Mining, which systematically extracts fine-grained political topics and their corresponding bias indicators from weakly labeled news data, and (2) Cross-Encoder Political Bias Embedding, which assigns structured bias scores to news articles based on their alignment with these indicators. This approach ensures that embeddings are explicitly tied to bias-revealing dimensions, enhancing both interpretability and predictive power. Through extensive experiments on two large-scale datasets, we demonstrate that PRISM outperforms state-of-the-art text embedding models in political bias classification while offering highly interpretable representations that facilitate diversified retrieval and ideological analysis. The source code is available at https://github.com/dukesun99/ACL-PRISM.
* Accepted to ACL 2025
Via
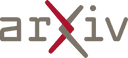
May 28, 2025
Abstract:Systematic reviews (SR), in which experts summarize and analyze evidence across individual studies to provide insights on a specialized topic, are a cornerstone for evidence-based clinical decision-making, research, and policy. Given the exponential growth of scientific articles, there is growing interest in using large language models (LLMs) to automate SR generation. However, the ability of LLMs to critically assess evidence and reason across multiple documents to provide recommendations at the same proficiency as domain experts remains poorly characterized. We therefore ask: Can LLMs match the conclusions of systematic reviews written by clinical experts when given access to the same studies? To explore this question, we present MedEvidence, a benchmark pairing findings from 100 SRs with the studies they are based on. We benchmark 24 LLMs on MedEvidence, including reasoning, non-reasoning, medical specialist, and models across varying sizes (from 7B-700B). Through our systematic evaluation, we find that reasoning does not necessarily improve performance, larger models do not consistently yield greater gains, and knowledge-based fine-tuning degrades accuracy on MedEvidence. Instead, most models exhibit similar behavior: performance tends to degrade as token length increases, their responses show overconfidence, and, contrary to human experts, all models show a lack of scientific skepticism toward low-quality findings. These results suggest that more work is still required before LLMs can reliably match the observations from expert-conducted SRs, even though these systems are already deployed and being used by clinicians. We release our codebase and benchmark to the broader research community to further investigate LLM-based SR systems.
Via
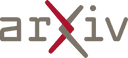
May 30, 2025
Abstract:Steering vectors are a lightweight method for controlling text properties by adding a learned bias to language model activations at inference time. So far, steering vectors have predominantly been evaluated in multiple-choice settings, while their effectiveness in free-form generation tasks remains understudied. Moving "Beyond Multiple Choice," we thoroughly evaluate the effectiveness of steering vectors in adaptively controlling topical focus, sentiment, toxicity, and readability in abstractive summaries of the NEWTS dataset. We find that steering effectively controls the targeted summary properties, but high steering strengths consistently degrade both intrinsic and extrinsic text quality. Compared to steering, prompting offers weaker control, while preserving text quality. Combining steering and prompting yields the strongest control over text properties and offers the most favorable efficacy-quality trade-off at moderate steering strengths. Our results underscore the practical trade-off between control strength and text quality preservation when applying steering vectors to free-form generation tasks.
* 29 pages, 21 figures, preprint
Via
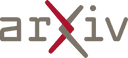
May 20, 2025
Abstract:EasyMath is a compact benchmark for practical math reasoning in small language models. It covers thirteen categories, from basic arithmetic and order of operations to word problems, algebraic expressions, edge cases, and omits specialist topics. We tested 23 models (14M to 4B parameters) using exact, numerical, and symbolic checks on free-form answers in a zero-shot setting. Accuracy rises with size and training, chain-of-thought adds modest gains, and consistency improves at scale.
* 17 pages, 9 figures, 8 tables
Via
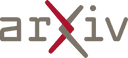
May 14, 2025
Abstract:Wildfires have become increasingly frequent, irregular, and severe in recent years. Understanding how affected populations perceive and respond during wildfire crises is critical for timely and empathetic disaster response. Social media platforms offer a crowd-sourced channel to capture evolving public discourse, providing hyperlocal information and insight into public sentiment. This study analyzes Reddit discourse during the 2025 Los Angeles wildfires, spanning from the onset of the disaster to full containment. We collect 385 posts and 114,879 comments related to the Palisades and Eaton fires. We adopt topic modeling methods to identify the latent topics, enhanced by large language models (LLMs) and human-in-the-loop (HITL) refinement. Furthermore, we develop a hierarchical framework to categorize latent topics, consisting of two main categories, Situational Awareness (SA) and Crisis Narratives (CN). The volume of SA category closely aligns with real-world fire progressions, peaking within the first 2-5 days as the fires reach the maximum extent. The most frequent co-occurring category set of public health and safety, loss and damage, and emergency resources expands on a wide range of health-related latent topics, including environmental health, occupational health, and one health. Grief signals and mental health risks consistently accounted for 60 percentage and 40 percentage of CN instances, respectively, with the highest total volume occurring at night. This study contributes the first annotated social media dataset on the 2025 LA fires, and introduces a scalable multi-layer framework that leverages topic modeling for crisis discourse analysis. By identifying persistent public health concerns, our results can inform more empathetic and adaptive strategies for disaster response, public health communication, and future research in comparable climate-related disaster events.
Via
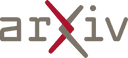