Topic:Text Classification
What is Text Classification? Text classification is the process of categorizing text documents into predefined categories or labels.
Papers and Code
May 29, 2025
Abstract:Process mining aims to discover, monitor and optimize the actual behaviors of real processes. While prior work has mainly focused on extracting procedural action flows from instructional texts, rule flows embedded in business documents remain underexplored. To this end, we introduce a novel annotated Chinese dataset, BPRF, which contains 50 business process documents with 326 explicitly labeled business rules across multiple domains. Each rule is represented as a <Condition, Action> pair, and we annotate logical dependencies between rules (sequential, conditional, or parallel). We also propose ExIde, a framework for automatic business rule extraction and dependency relationship identification using large language models (LLMs). We evaluate ExIde using 12 state-of-the-art (SOTA) LLMs on the BPRF dataset, benchmarking performance on both rule extraction and dependency classification tasks of current LLMs. Our results demonstrate the effectiveness of ExIde in extracting structured business rules and analyzing their interdependencies for current SOTA LLMs, paving the way for more automated and interpretable business process automation.
Via
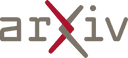
May 27, 2025
Abstract:The growing population of L2 English speakers has increased the demand for developing automatic graders for spoken language assessment (SLA). Historically, statistical models, text encoders, and self-supervised speech models have been utilised for this task. However, cascaded systems suffer from the loss of information, while E2E graders also have limitations. With the recent advancements of multi-modal large language models (LLMs), we aim to explore their potential as L2 oral proficiency graders and overcome these issues. In this work, we compare various training strategies using regression and classification targets. Our results show that speech LLMs outperform all previous competitive baselines, achieving superior performance on two datasets. Furthermore, the trained grader demonstrates strong generalisation capabilities in the cross-part or cross-task evaluation, facilitated by the audio understanding knowledge acquired during LLM pre-training.
* submitted to Interspeech
Via
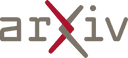
May 27, 2025
Abstract:While graph neural networks (GNNs) have shown remarkable performance across diverse graph-related tasks, their high-dimensional hidden representations render them black boxes. In this work, we propose Graph Lingual Network (GLN), a GNN built on large language models (LLMs), with hidden representations in the form of human-readable text. Through careful prompt design, GLN incorporates not only the message passing module of GNNs but also advanced GNN techniques, including graph attention and initial residual connection. The comprehensibility of GLN's hidden representations enables an intuitive analysis of how node representations change (1) across layers and (2) under advanced GNN techniques, shedding light on the inner workings of GNNs. Furthermore, we demonstrate that GLN achieves strong zero-shot performance on node classification and link prediction, outperforming existing LLM-based baseline methods.
Via
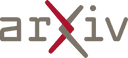
May 15, 2025
Abstract:Large Language Models (LLMs) have demonstrated remarkable capabilities in generating text that closely resembles human writing across a wide range of styles and genres. However, such capabilities are prone to potential misuse, such as fake news generation, spam email creation, and misuse in academic assignments. As a result, accurate detection of AI-generated text and identification of the model that generated it are crucial for maintaining the responsible use of LLMs. In this work, we addressed two sub-tasks put forward by the Defactify workshop under AI-Generated Text Detection shared task at the Association for the Advancement of Artificial Intelligence (AAAI 2025): Task A involved distinguishing between human-authored or AI-generated text, while Task B focused on attributing text to its originating language model. For each task, we proposed two neural architectures: an optimized model and a simpler variant. For Task A, the optimized neural architecture achieved fifth place with $F1$ score of 0.994, and for Task B, the simpler neural architecture also ranked fifth place with $F1$ score of 0.627.
Via
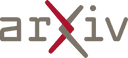
May 23, 2025
Abstract:Spiking Neural Networks (SNNs) provide an energy-efficient way to extract 3D spatio-temporal features. However, existing SNNs still exhibit a significant performance gap compared to Artificial Neural Networks (ANNs) due to inadequate pre-training strategies. These limitations manifest as restricted generalization ability, task specificity, and a lack of multimodal understanding, particularly in challenging tasks such as multimodal question answering and zero-shot 3D classification. To overcome these challenges, we propose a Spike-based Vision-Language (SVL) pretraining framework that empowers SNNs with open-world 3D understanding while maintaining spike-driven efficiency. SVL introduces two key components: (i) Multi-scale Triple Alignment (MTA) for label-free triplet-based contrastive learning across 3D, image, and text modalities, and (ii) Re-parameterizable Vision-Language Integration (Rep-VLI) to enable lightweight inference without relying on large text encoders. Extensive experiments show that SVL achieves a top-1 accuracy of 85.4% in zero-shot 3D classification, surpassing advanced ANN models, and consistently outperforms prior SNNs on downstream tasks, including 3D classification (+6.1%), DVS action recognition (+2.1%), 3D detection (+1.1%), and 3D segmentation (+2.1%) with remarkable efficiency. Moreover, SVL enables SNNs to perform open-world 3D question answering, sometimes outperforming ANNs. To the best of our knowledge, SVL represents the first scalable, generalizable, and hardware-friendly paradigm for 3D open-world understanding, effectively bridging the gap between SNNs and ANNs in complex open-world understanding tasks. Code is available https://github.com/bollossom/SVL.
Via
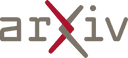
May 10, 2025
Abstract:Robustness to label noise within data is a significant challenge in federated learning (FL). From the data-centric perspective, the data quality of distributed datasets can not be guaranteed since annotations of different clients contain complicated label noise of varying degrees, which causes the performance degradation. There have been some early attempts to tackle noisy labels in FL. However, there exists a lack of benchmark studies on comprehensively evaluating their practical performance under unified settings. To this end, we propose the first benchmark study FNBench to provide an experimental investigation which considers three diverse label noise patterns covering synthetic label noise, imperfect human-annotation errors and systematic errors. Our evaluation incorporates eighteen state-of-the-art methods over five image recognition datasets and one text classification dataset. Meanwhile, we provide observations to understand why noisy labels impair FL, and additionally exploit a representation-aware regularization method to enhance the robustness of existing methods against noisy labels based on our observations. Finally, we discuss the limitations of this work and propose three-fold future directions. To facilitate related communities, our source code is open-sourced at https://github.com/Sprinter1999/FNBench.
* Submitted to IEEE TDSC, currently under major revision
Via
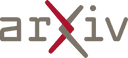
May 28, 2025
Abstract:The development of large-scale image-text pair datasets has significantly advanced self-supervised learning in Vision-Language Processing (VLP). However, directly applying general-domain architectures such as CLIP to medical data presents challenges, particularly in handling negations and addressing the inherent data imbalance of medical datasets. To address these issues, we propose a novel approach that integrates clinically-enhanced dynamic soft labels and medical graphical alignment, thereby improving clinical comprehension and the applicability of contrastive loss in medical contexts. Furthermore, we introduce negation-based hard negatives to deepen the model's understanding of the complexities of clinical language. Our approach is easily integrated into the medical CLIP training pipeline and achieves state-of-the-art performance across multiple tasks, including zero-shot, fine-tuned classification, and report retrieval. To comprehensively evaluate our model's capacity for understanding clinical language, we introduce CXR-Align, a benchmark uniquely designed to evaluate the understanding of negation and clinical information within chest X-ray (CXR) datasets. Experimental results demonstrate that our proposed methods are straightforward to implement and generalize effectively across contrastive learning frameworks, enhancing medical VLP capabilities and advancing clinical language understanding in medical imaging.
* 16 pages (8 main, 2 references, 6 appendix), 13 figures. Accepted to
CVPR 2025. This author-accepted manuscript includes an expanded ethics/data
user agreement section. The final version will appear in the Proceedings of
CVPR 2025
Via
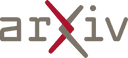
May 14, 2025
Abstract:I develop Ornithologist, a weakly-supervised textual classification system and measure the hawkishness and dovishness of central bank text. Ornithologist uses ``taxonomy-guided reasoning'', guiding a large language model with human-authored decision trees. This increases the transparency and explainability of the system and makes it accessible to non-experts. It also reduces hallucination risk. Since it requires less supervision than traditional classification systems, it can more easily be applied to other problems or sources of text (e.g. news) without much modification. Ornithologist measurements of hawkishness and dovishness of RBA communication carry information about the future of the cash rate path and of market expectations.
* 16 pages, 6 figures
Via
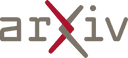
May 23, 2025
Abstract:Ground deformation is regarded in volcanology as a key precursor signal preceding volcanic eruptions. Satellite-based Interferometric Synthetic Aperture Radar (InSAR) enables consistent, global-scale deformation tracking; however, deep learning methods remain largely unexplored in this domain, mainly due to the lack of a curated machine learning dataset. In this work, we build on the existing Hephaestus dataset, and introduce Hephaestus Minicubes, a global collection of 38 spatiotemporal datacubes offering high resolution, multi-source and multi-temporal information, covering 44 of the world's most active volcanoes over a 7-year period. Each spatiotemporal datacube integrates InSAR products, topographic data, as well as atmospheric variables which are known to introduce signal delays that can mimic ground deformation in InSAR imagery. Furthermore, we provide expert annotations detailing the type, intensity and spatial extent of deformation events, along with rich text descriptions of the observed scenes. Finally, we present a comprehensive benchmark, demonstrating Hephaestus Minicubes' ability to support volcanic unrest monitoring as a multi-modal, multi-temporal classification and semantic segmentation task, establishing strong baselines with state-of-the-art architectures. This work aims to advance machine learning research in volcanic monitoring, contributing to the growing integration of data-driven methods within Earth science applications.
Via
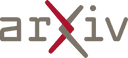
May 21, 2025
Abstract:Despite the wide-scale usage and development of emotion classification datasets in NLP, the field lacks a standardized, large-scale resource that follows a psychologically grounded taxonomy. Existing datasets either use inconsistent emotion categories, suffer from limited sample size, or focus on specific domains. The Super Emotion Dataset addresses this gap by harmonizing diverse text sources into a unified framework based on Shaver's empirically validated emotion taxonomy, enabling more consistent cross-domain emotion recognition research.
Via
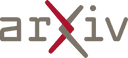