What is Recommendation? Recommendation is the task of providing personalized suggestions to users based on their preferences and behavior.
Papers and Code
Jul 02, 2025
Abstract:Deep Recommender Models (DLRMs) inference is a fundamental AI workload accounting for more than 79% of the total AI workload in Meta's data centers. DLRMs' performance bottleneck is found in the embedding layers, which perform many random memory accesses to retrieve small embedding vectors from tables of various sizes. We propose the design of tailored data flows to speedup embedding look-ups. Namely, we propose four strategies to look up an embedding table effectively on one core, and a framework to automatically map the tables asymmetrically to the multiple cores of a SoC. We assess the effectiveness of our method using the Huawei Ascend AI accelerators, comparing it with the default Ascend compiler, and we perform high-level comparisons with Nvidia A100. Results show a speed-up varying from 1.5x up to 6.5x for real workload distributions, and more than 20x for extremely unbalanced distributions. Furthermore, the method proves to be much more independent of the query distribution than the baseline.
* 2024 IEEE 42nd International Conference on Computer Design (ICCD),
Milan, Italy, 2024, pp. 517-520
* 5 pages, 4 figures, conference: IEEE ICCD24
Via
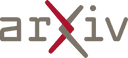
Jul 02, 2025
Abstract:Group recommendation over social media streams has attracted significant attention due to its wide applications in domains such as e-commerce, entertainment, and online news broadcasting. By leveraging social connections and group behaviours, group recommendation (GR) aims to provide more accurate and engaging content to a set of users rather than individuals. Recently, influence-aware GR has emerged as a promising direction, as it considers the impact of social influence on group decision-making. In earlier work, we proposed Influence-aware Group Recommendation (IGR) to solve this task. However, this task remains challenging due to three key factors: the large and ever-growing scale of social graphs, the inherently dynamic nature of influence propagation within user groups, and the high computational overhead of real-time group-item matching. To tackle these issues, we propose an Enhanced Influence-aware Group Recommendation (EIGR) framework. First, we introduce a Graph Extraction-based Sampling (GES) strategy to minimise redundancy across multiple temporal social graphs and effectively capture the evolving dynamics of both groups and items. Second, we design a novel DYnamic Independent Cascade (DYIC) model to predict how influence propagates over time across social items and user groups. Finally, we develop a two-level hash-based User Group Index (UG-Index) to efficiently organise user groups and enable real-time recommendation generation. Extensive experiments on real-world datasets demonstrate that our proposed framework, EIGR, consistently outperforms state-of-the-art baselines in both effectiveness and efficiency.
Via
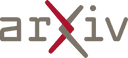
Jul 02, 2025
Abstract:We introduce ManifoldMind, a probabilistic geometric recommender system for exploratory reasoning over semantic hierarchies in hyperbolic space. Unlike prior methods with fixed curvature and rigid embeddings, ManifoldMind represents users, items, and tags as adaptive-curvature probabilistic spheres, enabling personalised uncertainty modeling and geometry-aware semantic exploration. A curvature-aware semantic kernel supports soft, multi-hop inference, allowing the model to explore diverse conceptual paths instead of overfitting to shallow or direct interactions. Experiments on four public benchmarks show superior NDCG, calibration, and diversity compared to strong baselines. ManifoldMind produces explicit reasoning traces, enabling transparent, trustworthy, and exploration-driven recommendations in sparse or abstract domains.
Via
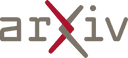
Jul 02, 2025
Abstract:Graph convolutional networks (GCNs) are fundamental in various scientific applications, ranging from biomedical protein-protein interactions (PPI) to large-scale recommendation systems. An essential component for modeling graph structures in GCNs is sparse general matrix-matrix multiplication (SpGEMM). As the size of graph data continues to scale up, SpGEMMs are often conducted in an out-of-core fashion due to limited GPU memory space in resource-constrained systems. Albeit recent efforts that aim to alleviate the memory constraints of out-of-core SpGEMM through either GPU feature caching, hybrid CPU-GPU memory layout, or performing the computation in sparse format, current systems suffer from both high I/O latency and GPU under-utilization issues. In this paper, we first identify the problems of existing systems, where sparse format data alignment and memory allocation are the main performance bottlenecks, and propose AIRES, a novel algorithm-system co-design solution to accelerate out-of-core SpGEMM computation for GCNs. Specifically, from the algorithm angle, AIRES proposes to alleviate the data alignment issues on the block level for matrices in sparse formats and develops a tiling algorithm to facilitate row block-wise alignment. On the system level, AIRES employs a three-phase dynamic scheduling that features a dual-way data transfer strategy utilizing a tiered memory system: integrating GPU memory, GPU Direct Storage (GDS), and host memory to reduce I/O latency and improve throughput. Evaluations show that AIRES significantly outperforms the state-of-the-art methods, achieving up to 1.8x lower latency in real-world graph processing benchmarks.
* 36th IEEE International Conference on Application-Specific Systems,
Architectures and Processors. (Accepted)
Via
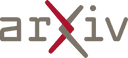
Jul 02, 2025
Abstract:Federated recommendation (FedRec) preserves user privacy by enabling decentralized training of personalized models, but this architecture is inherently vulnerable to adversarial attacks. Significant research has been conducted on targeted attacks in FedRec systems, motivated by commercial and social influence considerations. However, much of this work has largely overlooked the differential robustness of recommendation models. Moreover, our empirical findings indicate that existing targeted attack methods achieve only limited effectiveness in Federated Sequential Recommendation(FSR) tasks. Driven by these observations, we focus on investigating targeted attacks in FSR and propose a novel dualview attack framework, named DV-FSR. This attack method uniquely combines a sampling-based explicit strategy with a contrastive learning-based implicit gradient strategy to orchestrate a coordinated attack. Additionally, we introduce a specific defense mechanism tailored for targeted attacks in FSR, aiming to evaluate the mitigation effects of the attack method we proposed. Extensive experiments validate the effectiveness of our proposed approach on representative sequential models. Our codes are publicly available.
* 10 pages. arXiv admin note: substantial text overlap with
arXiv:2409.07500; text overlap with arXiv:2212.05399 by other authors
Via
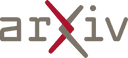
Jul 02, 2025
Abstract:As AI integrates in various types of human writing, calls for transparency around AI assistance are growing. However, if transparency operates on uneven ground and certain identity groups bear a heavier cost for being honest, then the burden of openness becomes asymmetrical. This study investigates how AI disclosure statement affects perceptions of writing quality, and whether these effects vary by the author's race and gender. Through a large-scale controlled experiment, both human raters (n = 1,970) and LLM raters (n = 2,520) evaluated a single human-written news article while disclosure statements and author demographics were systematically varied. This approach reflects how both human and algorithmic decisions now influence access to opportunities (e.g., hiring, promotion) and social recognition (e.g., content recommendation algorithms). We find that both human and LLM raters consistently penalize disclosed AI use. However, only LLM raters exhibit demographic interaction effects: they favor articles attributed to women or Black authors when no disclosure is present. But these advantages disappear when AI assistance is revealed. These findings illuminate the complex relationships between AI disclosure and author identity, highlighting disparities between machine and human evaluation patterns.
* Presented at CHIWORK 2025 Workshop on Generative AI Disclosure,
Ownership, and Accountability in Co-Creative Domains
Via
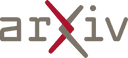
Jul 02, 2025
Abstract:Graph federated recommendation systems offer a privacy-preserving alternative to traditional centralized recommendation architectures, which often raise concerns about data security. While federated learning enables personalized recommendations without exposing raw user data, existing aggregation methods overlook the unique properties of user embeddings in this setting. Indeed, traditional aggregation methods fail to account for their complexity and the critical role of user similarity in recommendation effectiveness. Moreover, evolving user interactions require adaptive aggregation while preserving the influence of high-relevance anchor users (the primary users before expansion in graph-based frameworks). To address these limitations, we introduce Dist-FedAvg, a novel distance-based aggregation method designed to enhance personalization and aggregation efficiency in graph federated learning. Our method assigns higher aggregation weights to users with similar embeddings, while ensuring that anchor users retain significant influence in local updates. Empirical evaluations on multiple datasets demonstrate that Dist-FedAvg consistently outperforms baseline aggregation techniques, improving recommendation accuracy while maintaining seamless integration into existing federated learning frameworks.
* 17 pages, 5 figures
Via
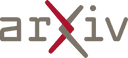
Jul 02, 2025
Abstract:Large language models (LLMs) are rapidly evolving from passive engines of text generation into agentic entities that can plan, remember, invoke external tools, and co-operate with one another. This perspective paper investigates how such LLM agents (and societies thereof) can transform the design space of recommender systems. We introduce a unified formalism that (i) models an individual agent as a tuple comprising its language core, tool set, and hierarchical memory, and (ii) captures a multi-agent recommender as a triple of agents, shared environment, and communication protocol. Within this framework, we present four end-to-end use cases-interactive party planning, synthetic user-simulation for offline evaluation, multi-modal furniture recommendation, and brand-aligned explanation generation-each illustrating a distinct capability unlocked by agentic orchestration. We then surface five cross-cutting challenge families: protocol complexity, scalability, hallucination and error propagation, emergent misalignment (including covert collusion), and brand compliance. For each, we formalize the problem, review nascent mitigation strategies, and outline open research questions. The result is both a blueprint and an agenda: a blueprint that shows how memory-augmented, tool-using LLM agents can be composed into robust recommendation pipelines, and an agenda inviting the RecSys community to develop benchmarks, theoretical guarantees, and governance tools that keep pace with this new degree of autonomy. By unifying agentic abstractions with recommender objectives, the paper lays the groundwork for the next generation of personalized, trustworthy, and context-rich recommendation services.
Via
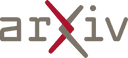
Jul 02, 2025
Abstract:Automatic medical coding has the potential to ease documentation and billing processes. For this task, transparency plays an important role for medical coders and regulatory bodies, which can be achieved using explainability methods. However, the evaluation of these approaches has been mostly limited to short text and binary settings due to a scarcity of annotated data. Recent efforts by Cheng et al. (2023) have introduced the MDACE dataset, which provides a valuable resource containing code evidence in clinical records. In this work, we conduct an in-depth analysis of the MDACE dataset and perform plausibility evaluation of current explainable medical coding systems from an applied perspective. With this, we contribute to a deeper understanding of automatic medical coding and evidence extraction. Our findings reveal that ground truth evidence aligns with code descriptions to a certain degree. An investigation into state-of-the-art approaches shows a high overlap with ground truth evidence. We propose match measures and highlight success and failure cases. Based on our findings, we provide recommendations for developing and evaluating explainable medical coding systems.
* Accepted to ACL 2025 Findings
Via
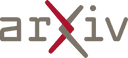
Jul 01, 2025
Abstract:There is growing interest in explainable recommender systems that provide recommendations along with explanations for the reasoning behind them. When evaluating recommender systems, most studies focus on overall recommendation performance. Only a few assess the quality of the explanations. Explanation quality is often evaluated through user studies that subjectively gather users' opinions on representative explanatory factors that shape end-users' perspective towards the results, not about the explanation contents itself. We aim to fill this gap by developing an objective metric to evaluate Veracity: the information quality of explanations. Specifically, we decompose Veracity into two dimensions: Fidelity and Attunement. Fidelity refers to whether the explanation includes accurate information about the recommended item. Attunement evaluates whether the explanation reflects the target user's preferences. By applying signal detection theory, we first determine decision outcomes for each dimension and then combine them to calculate a sensitivity, which serves as the final Veracity value. To assess the effectiveness of the proposed metric, we set up four cases with varying levels of information quality to validate whether our metric can accurately capture differences in quality. The results provided meaningful insights into the effectiveness of our proposed metric.
* Accepted to IEEE CAI 2025
Via
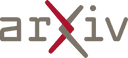