What is Recommendation? Recommendation is the task of providing personalized suggestions to users based on their preferences and behavior.
Papers and Code
Jun 04, 2025
Abstract:The NLP research community has made publicly available numerous instruments for measuring representational harms caused by large language model (LLM)-based systems. These instruments have taken the form of datasets, metrics, tools, and more. In this paper, we examine the extent to which such instruments meet the needs of practitioners tasked with evaluating LLM-based systems. Via semi-structured interviews with 12 such practitioners, we find that practitioners are often unable to use publicly available instruments for measuring representational harms. We identify two types of challenges. In some cases, instruments are not useful because they do not meaningfully measure what practitioners seek to measure or are otherwise misaligned with practitioner needs. In other cases, instruments - even useful instruments - are not used by practitioners due to practical and institutional barriers impeding their uptake. Drawing on measurement theory and pragmatic measurement, we provide recommendations for addressing these challenges to better meet practitioner needs.
* Findings of the Association for Computational Linguistics: ACL 2025
Via
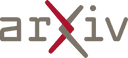
Jun 04, 2025
Abstract:Nearest neighbor (NN) methods have re-emerged as competitive tools for matrix completion, offering strong empirical performance and recent theoretical guarantees, including entry-wise error bounds, confidence intervals, and minimax optimality. Despite their simplicity, recent work has shown that NN approaches are robust to a range of missingness patterns and effective across diverse applications. This paper introduces N$^2$, a unified Python package and testbed that consolidates a broad class of NN-based methods through a modular, extensible interface. Built for both researchers and practitioners, N$^2$ supports rapid experimentation and benchmarking. Using this framework, we introduce a new NN variant that achieves state-of-the-art results in several settings. We also release a benchmark suite of real-world datasets, from healthcare and recommender systems to causal inference and LLM evaluation, designed to stress-test matrix completion methods beyond synthetic scenarios. Our experiments demonstrate that while classical methods excel on idealized data, NN-based techniques consistently outperform them in real-world settings.
* 21 pages, 6 figures
Via
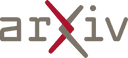
Jun 04, 2025
Abstract:Large Language Models (LLMs) have demonstrated remarkable performance across various Natural Language Processing (NLP) tasks, largely due to their generalisability and ability to perform tasks without additional training. However, their effectiveness for low-resource languages remains limited. In this study, we evaluate the performance of 55 publicly available LLMs on Maltese, a low-resource language, using a newly introduced benchmark covering 11 discriminative and generative tasks. Our experiments highlight that many models perform poorly, particularly on generative tasks, and that smaller fine-tuned models often perform better across all tasks. From our multidimensional analysis, we investigate various factors impacting performance. We conclude that prior exposure to Maltese during pre-training and instruction-tuning emerges as the most important factor. We also examine the trade-offs between fine-tuning and prompting, highlighting that while fine-tuning requires a higher initial cost, it yields better performance and lower inference costs. Through this work, we aim to highlight the need for more inclusive language technologies and recommend that researchers working with low-resource languages consider more "traditional" language modelling approaches.
* ACL 2025 Findings Camera-Ready
Via
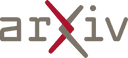
May 30, 2025
Abstract:Heterogeneous graph neural networks (HGNNs) have demonstrated their superiority in exploiting auxiliary information for recommendation tasks. However, graphs constructed using meta-paths in HGNNs are usually too dense and contain a large number of noise edges. The propagation mechanism of HGNNs propagates even small amounts of noise in a graph to distant neighboring nodes, thereby affecting numerous node embeddings. To address this limitation, we introduce a novel model, named Masked Contrastive Learning (MCL), to enhance recommendation robustness to noise. MCL employs a random masking strategy to augment the graph via meta-paths, reducing node sensitivity to specific neighbors and bolstering embedding robustness. Furthermore, MCL employs contrastive cross-view on a Heterogeneous Information Network (HIN) from two perspectives: one-hop neighbors and meta-path neighbors. This approach acquires embeddings capturing both local and high-order structures simultaneously for recommendation. Empirical evaluations on three real-world datasets confirm the superiority of our approach over existing recommendation methods.
* 12 pages, 7 figures
Via
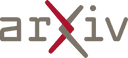
May 30, 2025
Abstract:This paper presents a comprehensive analysis of the linguistic diversity of LLM safety research, highlighting the English-centric nature of the field. Through a systematic review of nearly 300 publications from 2020--2024 across major NLP conferences and workshops at *ACL, we identify a significant and growing language gap in LLM safety research, with even high-resource non-English languages receiving minimal attention. We further observe that non-English languages are rarely studied as a standalone language and that English safety research exhibits poor language documentation practice. To motivate future research into multilingual safety, we make several recommendations based on our survey, and we then pose three concrete future directions on safety evaluation, training data generation, and crosslingual safety generalization. Based on our survey and proposed directions, the field can develop more robust, inclusive AI safety practices for diverse global populations.
Via
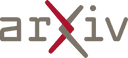
May 30, 2025
Abstract:The performance of large language models (LLMs) continues to improve, as reflected in rising scores on standard benchmarks. However, the lack of transparency around training data raises concerns about potential overlap with evaluation sets and the fairness of reported results. Although prior work has proposed methods for detecting data leakage, these approaches primarily focus on identifying outliers and have not been evaluated under controlled simulated leakage conditions. In this work, we compare existing leakage detection techniques, namely permutation and n-gram-based methods, under a continual pretraining setup that simulates real-world leakage scenarios, and additionally explore a lightweight method we call semi-half question. Although semi-half offers a low-cost alternative, our analysis shows that the n-gram method consistently achieves the highest F1-score. We also refine these techniques to support instance-level detection and reduce computational overhead. Leveraging the best-performing method, we create cleaned versions of MMLU and HellaSwag, and re-evaluate several LLMs. Our findings present a practical path toward more reliable and transparent evaluations, and we recommend contamination checks as a standard step before releasing benchmark results.
Via
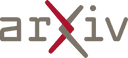
May 30, 2025
Abstract:In the era of foundation models, Out-of- Distribution (OOD) problems, i.e., the data discrepancy between the training environments and testing environments, hinder AI generalization. Further, relational data like graphs disobeying the Independent and Identically Distributed (IID) condition makes the problem more challenging, especially much harder when it is associated with time. Motivated by this, to realize the robust invariant learning over temporal graphs, we want to investigate what components in temporal graphs are most invariant and representative with respect to labels. With the Information Bottleneck (IB) method, we propose an error-bounded Invariant Link Selector that can distinguish invariant components and variant components during the training process to make the deep learning model generalizable for different testing scenarios. Besides deriving a series of rigorous generalizable optimization functions, we also equip the training with task-specific loss functions, e.g., temporal link prediction, to make pretrained models solve real-world application tasks like citation recommendation and merchandise recommendation, as demonstrated in our experiments with state-of-the-art (SOTA) methods. Our code is available at https://github.com/kthrn22/OOD-Linker.
* Accepted by AISTATS 2025. 22 pages, 2 figures, 6 tables
Via
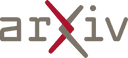
May 29, 2025
Abstract:This white paper discusses and explores the various points of intersection between quantum computing and artificial intelligence (AI). It describes how quantum computing could support the development of innovative AI solutions. It also examines use cases of classical AI that can empower research and development in quantum technologies, with a focus on quantum computing and quantum sensing. The purpose of this white paper is to provide a long-term research agenda aimed at addressing foundational questions about how AI and quantum computing interact and benefit one another. It concludes with a set of recommendations and challenges, including how to orchestrate the proposed theoretical work, align quantum AI developments with quantum hardware roadmaps, estimate both classical and quantum resources - especially with the goal of mitigating and optimizing energy consumption - advance this emerging hybrid software engineering discipline, and enhance European industrial competitiveness while considering societal implications.
* 32 pages, 3 figures
Via
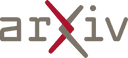
May 29, 2025
Abstract:A restaurant dinner or a hotel stay may lead to memorable experiences when guests encounter unexpected aspects that also match their interests. For example, an origami-making station in the waiting area of a restaurant may be both surprising and enjoyable for a customer who is passionate about paper crafts. Similarly, an exhibit of 18th century harpsichords would be atypical for a hotel lobby and likely pique the interest of a guest who has a passion for Baroque music. Motivated by this insight, in this paper we introduce the new task of engineering serendipity through recommendations of items with atypical aspects. We describe an LLM-based system pipeline that extracts atypical aspects from item reviews, then estimates and aggregates their user-specific utility in a measure of serendipity potential that is used to rerank a list of items recommended to the user. To facilitate system development and evaluation, we introduce a dataset of Yelp reviews that are manually annotated with atypical aspects and a dataset of artificially generated user profiles, together with crowdsourced annotations of user-aspect utility values. Furthermore, we introduce a custom procedure for dynamic selection of in-context learning examples, which is shown to improve LLM-based judgments of atypicality and utility. Experimental evaluations show that serendipity-based rankings generated by the system are highly correlated with ground truth rankings for which serendipity scores are computed from manual annotations of atypical aspects and their user-dependent utility. Overall, we hope that the new recommendation task and the associated system presented in this paper catalyze further research into recommendation approaches that go beyond accuracy in their pursuit of enhanced user satisfaction. The datasets and the code are made publicly available at https://github.com/ramituncc49er/ATARS .
* 25 pages of content + references and appendix. arXiv admin note: text
overlap with arXiv:2311.02702
Via
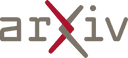
May 29, 2025
Abstract:Small Uncrewed Aerial Systems (sUAS) are increasingly deployed as autonomous swarms in search-and-rescue and other disaster-response scenarios. In these settings, they use computer vision (CV) to detect objects of interest and autonomously adapt their missions. However, traditional CV systems often struggle to recognize unfamiliar objects in open-world environments or to infer their relevance for mission planning. To address this, we incorporate large language models (LLMs) to reason about detected objects and their implications. While LLMs can offer valuable insights, they are also prone to hallucinations and may produce incorrect, misleading, or unsafe recommendations. To ensure safe and sensible decision-making under uncertainty, high-level decisions must be governed by cognitive guardrails. This article presents the design, simulation, and real-world integration of these guardrails for sUAS swarms in search-and-rescue missions.
* 16 pages, 8 figures
Via
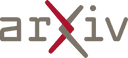