Topic:Story Visualization
What is Story Visualization? Story visualization is the task of generating coherent and aligned sequence of images given a sequence of textual captions representing description of a story. It mainly consists of two tasks: story generation and story continuation, where story continuation uses additional ground-truth information in the form of the first frame.
Papers and Code
Apr 24, 2025
Abstract:A data story typically integrates data facts from multiple perspectives and stances to construct a comprehensive and objective narrative. However, retrieving these facts demands time for data search and challenges the creator's analytical skills. In this work, we introduce DataScout, an interactive system that automatically performs reasoning and stance-based data facts retrieval to augment the user's statement. Particularly, DataScout leverages an LLM-based agent to construct a retrieval tree, enabling collaborative control of its expansion between users and the agent. The interface visualizes the retrieval tree as a mind map that eases users to intuitively steer the retrieval direction and effectively engage in reasoning and analysis. We evaluate the proposed system through case studies and in-depth expert interviews. Our evaluation demonstrates that DataScout can effectively retrieve multifaceted data facts from different stances, helping users verify their statements and enhance the credibility of their stories.
Via
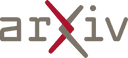
Apr 21, 2025
Abstract:We introduce Eagle 2.5, a family of frontier vision-language models (VLMs) for long-context multimodal learning. Our work addresses the challenges in long video comprehension and high-resolution image understanding, introducing a generalist framework for both tasks. The proposed training framework incorporates Automatic Degrade Sampling and Image Area Preservation, two techniques that preserve contextual integrity and visual details. The framework also includes numerous efficiency optimizations in the pipeline for long-context data training. Finally, we propose Eagle-Video-110K, a novel dataset that integrates both story-level and clip-level annotations, facilitating long-video understanding. Eagle 2.5 demonstrates substantial improvements on long-context multimodal benchmarks, providing a robust solution to the limitations of existing VLMs. Notably, our best model Eagle 2.5-8B achieves 72.4% on Video-MME with 512 input frames, matching the results of top-tier commercial model such as GPT-4o and large-scale open-source models like Qwen2.5-VL-72B and InternVL2.5-78B.
Via
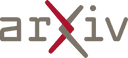
Mar 30, 2025
Abstract:Open-ended story visualization is a challenging task that involves generating coherent image sequences from a given storyline. One of the main difficulties is maintaining character consistency while creating natural and contextually fitting scenes--an area where many existing methods struggle. In this paper, we propose an enhanced Transformer module that uses separate self attention and cross attention mechanisms, leveraging prior knowledge from pre-trained diffusion models to ensure logical scene creation. The isolated self attention mechanism improves character consistency by refining attention maps to reduce focus on irrelevant areas and highlight key features of the same character. Meanwhile, the isolated cross attention mechanism independently processes each character's features, avoiding feature fusion and further strengthening consistency. Notably, our method is training-free, allowing the continuous generation of new characters and storylines without re-tuning. Both qualitative and quantitative evaluations show that our approach outperforms current methods, demonstrating its effectiveness.
* 6 pages, 4 figures
Via
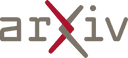
Apr 08, 2025
Abstract:Training-free consistent text-to-image generation depicting the same subjects across different images is a topic of widespread recent interest. Existing works in this direction predominantly rely on cross-frame self-attention; which improves subject-consistency by allowing tokens in each frame to pay attention to tokens in other frames during self-attention computation. While useful for single subjects, we find that it struggles when scaling to multiple characters. In this work, we first analyze the reason for these limitations. Our exploration reveals that the primary-issue stems from self-attention-leakage, which is exacerbated when trying to ensure consistency across multiple-characters. This happens when tokens from one subject pay attention to other characters, causing them to appear like each other (e.g., a dog appearing like a duck). Motivated by these findings, we propose StoryBooth: a training-free approach for improving multi-character consistency. In particular, we first leverage multi-modal chain-of-thought reasoning and region-based generation to apriori localize the different subjects across the desired story outputs. The final outputs are then generated using a modified diffusion model which consists of two novel layers: 1) a bounded cross-frame self-attention layer for reducing inter-character attention leakage, and 2) token-merging layer for improving consistency of fine-grain subject details. Through both qualitative and quantitative results we find that the proposed approach surpasses prior state-of-the-art, exhibiting improved consistency across both multiple-characters and fine-grain subject details.
Via
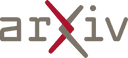
Apr 03, 2025
Abstract:Interactive storytelling benefits from planning and exploring multiple 'what if' scenarios. Modern LLMs are useful tools for ideation and exploration, but current chat-based user interfaces restrict users to a single linear flow. To address this limitation, we propose Narrative Studio -- a novel in-browser narrative exploration environment featuring a tree-like interface that allows branching exploration from user-defined points in a story. Each branch is extended via iterative LLM inference guided by system and user-defined prompts. Additionally, we employ Monte Carlo Tree Search (MCTS) to automatically expand promising narrative paths based on user-specified criteria, enabling more diverse and robust story development. We also allow users to enhance narrative coherence by grounding the generated text in an entity graph that represents the actors and environment of the story.
Via
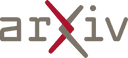
Mar 29, 2025
Abstract:Data-driven storytelling has gained prominence in journalism and other data reporting fields. However, the process of creating these stories remains challenging, often requiring the integration of effective visualizations with compelling narratives to form a cohesive, interactive presentation. To help streamline this process, we present an integrated authoring framework and system, DataWeaver, that supports both visualization-to-text and text-to-visualization composition. DataWeaver enables users to create data narratives anchored to data facts derived from "call-out" interactions, i.e., user-initiated highlights of visualization elements that prompt relevant narrative content. In addition to this "vis-to-text" composition, DataWeaver also supports a "text-initiated" approach, generating relevant interactive visualizations from existing narratives. Key findings from an evaluation with 13 participants highlighted the utility and usability of DataWeaver and the effectiveness of its integrated authoring framework. The evaluation also revealed opportunities to enhance the framework by refining filtering mechanisms and visualization recommendations and better support authoring creativity by introducing advanced customization options.
* Accepted to EuroVis 2025. Published in Computer Graphics Forum. DOI:
10.1111/cgf.70098
Via
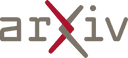
Mar 30, 2025
Abstract:Comics have long been a popular form of storytelling, offering visually engaging narratives that captivate audiences worldwide. However, the visual nature of comics presents a significant barrier for visually impaired readers, limiting their access to these engaging stories. In this work, we provide a pragmatic solution to this accessibility challenge by developing an automated system that generates text-based literary narratives from manga comics. Our approach aims to create an evocative and immersive prose that not only conveys the original narrative but also captures the depth and complexity of characters, their interactions, and the vivid settings in which they reside. To this end we make the following contributions: (1) We present a unified model, Magiv3, that excels at various functional tasks pertaining to comic understanding, such as localising panels, characters, texts, and speech-bubble tails, performing OCR, grounding characters etc. (2) We release human-annotated captions for over 3300 Japanese comic panels, along with character grounding annotations, and benchmark large vision-language models in their ability to understand comic images. (3) Finally, we demonstrate how integrating large vision-language models with Magiv3, can generate seamless literary narratives that allows visually impaired audiences to engage with the depth and richness of comic storytelling.
Via
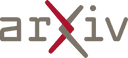
Mar 26, 2025
Abstract:Visual narrative generation transforms textual narratives into sequences of images illustrating the content of the text. However, generating visual narratives that are faithful to the input text and self-consistent across generated images remains an open challenge, due to the lack of knowledge constraints used for planning the stories. In this work, we propose a new benchmark, VinaBench, to address this challenge. Our benchmark annotates the underlying commonsense and discourse constraints in visual narrative samples, offering systematic scaffolds for learning the implicit strategies of visual storytelling. Based on the incorporated narrative constraints, we further propose novel metrics to closely evaluate the consistency of generated narrative images and the alignment of generations with the input textual narrative. Our results across three generative vision models demonstrate that learning with VinaBench's knowledge constraints effectively improves the faithfulness and cohesion of generated visual narratives.
* Proceedings of the IEEE/CVF Conference on Computer Vision and Pattern
Recognition (CVPR 2025)
Via
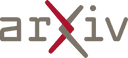
Mar 04, 2025
Abstract:Story visualization is the transformation of narrative elements into image sequences. While existing research has primarily focused on visual contextual coherence, the deeper narrative essence of stories often remains overlooked. This limitation hinders the practical application of these approaches, as generated images frequently fail to capture the intended meaning and nuances of the narrative fully. To address these challenges, we propose VisAgent, a training-free multi-agent framework designed to comprehend and visualize pivotal scenes within a given story. By considering story distillation, semantic consistency, and contextual coherence, VisAgent employs an agentic workflow. In this workflow, multiple specialized agents collaborate to: (i) refine layered prompts based on the narrative structure and (ii) seamlessly integrate \gt{generated} elements, including refined prompts, scene elements, and subject placement, into the final image. The empirically validated effectiveness confirms the framework's suitability for practical story visualization applications.
* Accepted to ICASSP 2025. Equal contribution from first two authors
Via
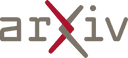
Mar 07, 2025
Abstract:The rapid advancement of large language models (LLMs) and artificial intelligence-generated content (AIGC) has accelerated AI-native applications, such as AI-based storybooks that automate engaging story production for children. However, challenges remain in improving story attractiveness, enriching storytelling expressiveness, and developing open-source evaluation benchmarks and frameworks. Therefore, we propose and opensource MM-StoryAgent, which creates immersive narrated video storybooks with refined plots, role-consistent images, and multi-channel audio. MM-StoryAgent designs a multi-agent framework that employs LLMs and diverse expert tools (generative models and APIs) across several modalities to produce expressive storytelling videos. The framework enhances story attractiveness through a multi-stage writing pipeline. In addition, it improves the immersive storytelling experience by integrating sound effects with visual, music and narrative assets. MM-StoryAgent offers a flexible, open-source platform for further development, where generative modules can be substituted. Both objective and subjective evaluation regarding textual story quality and alignment between modalities validate the effectiveness of our proposed MM-StoryAgent system. The demo and source code are available.
Via
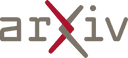