Jul 09, 2025
Abstract:Nowadays, speech emotion recognition (SER) plays a vital role in the field of human-computer interaction (HCI) and the evolution of artificial intelligence (AI). Our proposed DCRF-BiLSTM model is used to recognize seven emotions: neutral, happy, sad, angry, fear, disgust, and surprise, which are trained on five datasets: RAVDESS (R), TESS (T), SAVEE (S), EmoDB (E), and Crema-D (C). The model achieves high accuracy on individual datasets, including 97.83% on RAVDESS, 97.02% on SAVEE, 95.10% for CREMA-D, and a perfect 100% on both TESS and EMO-DB. For the combined (R+T+S) datasets, it achieves 98.82% accuracy, outperforming previously reported results. To our knowledge, no existing study has evaluated a single SER model across all five benchmark datasets (i.e., R+T+S+C+E) simultaneously. In our work, we introduce this comprehensive combination and achieve a remarkable overall accuracy of 93.76%. These results confirm the robustness and generalizability of our DCRF-BiLSTM framework across diverse datasets.
* 17 pages, 11 figures
Via
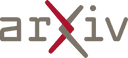