Jun 07, 2025
Abstract:Whispered speech is produced when the vocal folds are not used, either intentionally, or due to a temporary or permanent voice condition. The essential difference between natural speech and whispered speech is that periodic signal components that exist in certain regions of the former, called voiced regions, as a consequence of the vibration of the vocal folds, are missing in the latter. The restoration of natural speech from whispered speech requires delicate signal processing procedures that are especially useful if they can be implemented on low-resourced portable devices, in real-time, and on-the-fly, taking advantage of the established source-filter paradigm of voice production and related models. This paper addresses two challenges that are intertwined and are key in informing and making viable this envisioned technological realization. The first challenge involves characterizing and modeling the evolution of the harmonic phase/magnitude structure of a sequence of individual pitch periods in a voiced region of natural speech comprising sustained or co-articulated vowels. This paper proposes a novel algorithm segmenting individual pitch pulses, which is then used to obtain illustrative results highlighting important differences between sustained and co-articulated vowels, and suggesting practical synthetic voicing approaches. The second challenge involves model-based synthetic voicing. Three implementation alternatives are described that differ in their signal reconstruction approaches: frequency-domain, combined frequency and time-domain, and physiologically-inspired separate filtering of glottal excitation pulses individually generated. The three alternatives are compared objectively using illustrative examples, and subjectively using the results of listening tests involving synthetic voicing of sustained and co-articulated vowels in word context.
* 58 pages, 17 figures, 8 tables
Via
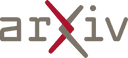