Jun 02, 2020
Abstract:With the increasing prevalence and more powerful camera systems of mobile devices, people can conveniently take photos in their daily life, which naturally brings the demand for more intelligent photo post-processing techniques, especially on those portrait photos. In this paper, we present a portrait recapture method enabling users to easily edit their portrait to desired posture/view, body figure and clothing style, which are very challenging to achieve since it requires to simultaneously perform non-rigid deformation of human body, invisible body-parts reasoning and semantic-aware editing. We decompose the editing procedure into semantic-aware geometric and appearance transformation. In geometric transformation, a semantic layout map is generated that meets user demands to represent part-level spatial constraints and further guides the semantic-aware appearance transformation. In appearance transformation, we design two novel modules, Semantic-aware Attentive Transfer (SAT) and Layout Graph Reasoning (LGR), to conduct intra-part transfer and inter-part reasoning, respectively. SAT module produces each human part by paying attention to the semantically consistent regions in the source portrait. It effectively addresses the non-rigid deformation issue and well preserves the intrinsic structure/appearance with rich texture details. LGR module utilizes body skeleton knowledge to construct a layout graph that connects all relevant part features, where graph reasoning mechanism is used to propagate information among part nodes to mine their relations. In this way, LGR module infers invisible body parts and guarantees global coherence among all the parts. Extensive experiments on DeepFashion, Market-1501 and in-the-wild photos demonstrate the effectiveness and superiority of our approach. Video demo is at: \url{https://youtu.be/vTyq9HL6jgw}.
* 14 pages
Via
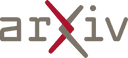