Mar 04, 2025
Abstract:"Magic" is referred to here and there in the robotics literature, from "magical moments" afforded by a mobile bubble machine, to "spells" intended to entertain and motivate children--but what exactly could this concept mean for designers? Here, we present (1) some theoretical discussion on how magic could inform interaction designs based on reviewing the literature, followed by (2) a practical description of using such ideas to develop a simplified prototype, which received an award in an international robot magic competition. Although this topic can be considered unusual and some negative connotations exist (e.g., unrealistic thinking can be referred to as magical), our results seem to suggest that magic, in the experiential, supernatural, and illusory senses of the term, could be useful to consider in various robot design contexts, also for artifacts like home assistants and autonomous vehicles--thus, inviting further discussion and exploration.
* Accepted Version of a Paper Published in IEEE, 10 pages, in the 34th
annual workshop of the Swedish Artificial Intelligence Society (SAIS 2022),
2022
Via
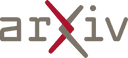