Mar 25, 2025
Abstract:Digital image spoofing has emerged as a significant security threat in biometric authentication systems, particularly those relying on facial recognition. This study evaluates the performance of three vision based models, MobileNetV2, ResNET50, and Vision Transformer, ViT, for spoof detection in image classification, utilizing a dataset of 150,986 images divided into training , 140,002, testing, 10,984, and validation ,39,574, sets. Spoof detection is critical for enhancing the security of image recognition systems, and this research compares the models effectiveness through accuracy, precision, recall, and F1 score metrics. Results reveal that MobileNetV2 outperforms other architectures on the test dataset, achieving an accuracy of 91.59%, precision of 91.72%, recall of 91.59%, and F1 score of 91.58%, compared to ViT 86.54%, 88.28%, 86.54%, and 86.39%, respectively. On the validation dataset, MobileNetV2, and ViT excel, with MobileNetV2 slightly ahead at 97.17% accuracy versus ViT 96.36%. MobileNetV2 demonstrates faster convergence during training and superior generalization to unseen data, despite both models showing signs of overfitting. These findings highlight MobileNetV2 balanced performance and robustness, making it the preferred choice for spoof detection applications where reliability on new data is essential. The study underscores the importance of model selection in security sensitive contexts and suggests MobileNetV2 as a practical solution for real world deployment.
* 26 pages, 9 figures,3 tables
Via
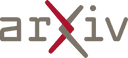