What is facial recognition? Facial recognition is an AI-based technique for identifying or confirming an individual's identity using their face. It maps facial features from an image or video and then compares the information with a collection of known faces to find a match.
Papers and Code
Apr 24, 2025
Abstract:Vision Large Language Models (VLLMs) exhibit promising potential for multi-modal understanding, yet their application to video-based emotion recognition remains limited by insufficient spatial and contextual awareness. Traditional approaches, which prioritize isolated facial features, often neglect critical non-verbal cues such as body language, environmental context, and social interactions, leading to reduced robustness in real-world scenarios. To address this gap, we propose Set-of-Vision-Text Prompting (SoVTP), a novel framework that enhances zero-shot emotion recognition by integrating spatial annotations (e.g., bounding boxes, facial landmarks), physiological signals (facial action units), and contextual cues (body posture, scene dynamics, others' emotions) into a unified prompting strategy. SoVTP preserves holistic scene information while enabling fine-grained analysis of facial muscle movements and interpersonal dynamics. Extensive experiments show that SoVTP achieves substantial improvements over existing visual prompting methods, demonstrating its effectiveness in enhancing VLLMs' video emotion recognition capabilities.
* 12 pages, 10 figures
Via
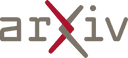
May 11, 2025
Abstract:Micro-expressions (MEs) are crucial psychological responses with significant potential for affective computing. However, current automatic micro-expression recognition (MER) research primarily focuses on discrete emotion classification, neglecting a convincing analysis of the subtle dynamic movements and inherent emotional cues. The rapid progress in multimodal large language models (MLLMs), known for their strong multimodal comprehension and language generation abilities, offers new possibilities. MLLMs have shown success in various vision-language tasks, indicating their potential to understand MEs comprehensively, including both fine-grained motion patterns and underlying emotional semantics. Nevertheless, challenges remain due to the subtle intensity and short duration of MEs, as existing MLLMs are not designed to capture such delicate frame-level facial dynamics. In this paper, we propose a novel Micro-Expression Large Language Model (MELLM), which incorporates a subtle facial motion perception strategy with the strong inference capabilities of MLLMs, representing the first exploration of MLLMs in the domain of ME analysis. Specifically, to explicitly guide the MLLM toward motion-sensitive regions, we construct an interpretable motion-enhanced color map by fusing onset-apex optical flow dynamics with the corresponding grayscale onset frame as the model input. Additionally, specialized fine-tuning strategies are incorporated to further enhance the model's visual perception of MEs. Furthermore, we construct an instruction-description dataset based on Facial Action Coding System (FACS) annotations and emotion labels to train our MELLM. Comprehensive evaluations across multiple benchmark datasets demonstrate that our model exhibits superior robustness and generalization capabilities in ME understanding (MEU). Code is available at https://github.com/zyzhangUstc/MELLM.
Via
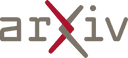
Apr 24, 2025
Abstract:Electroencephalography (EEG) signals provide a promising and involuntary reflection of brain activity related to emotional states, offering significant advantages over behavioral cues like facial expressions. However, EEG signals are often noisy, affected by artifacts, and vary across individuals, complicating emotion recognition. While multimodal approaches have used Peripheral Physiological Signals (PPS) like GSR to complement EEG, they often overlook the dynamic synchronization and consistent semantics between the modalities. Additionally, the temporal dynamics of emotional fluctuations across different time resolutions in PPS remain underexplored. To address these challenges, we propose PhysioSync, a novel pre-training framework leveraging temporal and cross-modal contrastive learning, inspired by physiological synchronization phenomena. PhysioSync incorporates Cross-Modal Consistency Alignment (CM-CA) to model dynamic relationships between EEG and complementary PPS, enabling emotion-related synchronizations across modalities. Besides, it introduces Long- and Short-Term Temporal Contrastive Learning (LS-TCL) to capture emotional synchronization at different temporal resolutions within modalities. After pre-training, cross-resolution and cross-modal features are hierarchically fused and fine-tuned to enhance emotion recognition. Experiments on DEAP and DREAMER datasets demonstrate PhysioSync's advanced performance under uni-modal and cross-modal conditions, highlighting its effectiveness for EEG-centered emotion recognition.
* The source code will be publicly available at
https://github.com/MSA-LMC/PhysioSync
Via
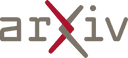
Apr 01, 2025
Abstract:This study used machine learning algorithms to identify actors and extract the age of actors from images taken randomly from movies. The use of images taken from Arab movies includes challenges such as non-uniform lighting, different and multiple poses for the actors and multiple elements with the actor or a group of actors. Additionally, the use of make-up, wigs, beards, and wearing different accessories and costumes made it difficult for the system to identify the personality of the same actor. The Arab Actors Dataset-AAD comprises 574 images sourced from various movies, encompassing both black and white as well as color compositions. The images depict complete scenes or fragments thereof. Multiple models were employed for feature extraction, and diverse machine learning algorithms were utilized during the classification and prediction stages to determine the most effective algorithm for handling such image types. The study demonstrated the effectiveness of the Logistic Regression model exhibited the best performance compared to other models in the training phase, as evidenced by its AUC, precision, CA and F1score values of 99%, 86%, 85.5% and 84.2% respectively. The findings of this study can be used to improve the precision and reliability of facial recognition technology for various uses as with movies search services, movie suggestion algorithms, and genre classification of movies.
Via
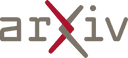
Apr 05, 2025
Abstract:Personalized facial expression recognition (FER) involves adapting a machine learning model using samples from labeled sources and unlabeled target domains. Given the challenges of recognizing subtle expressions with considerable interpersonal variability, state-of-the-art unsupervised domain adaptation (UDA) methods focus on the multi-source UDA (MSDA) setting, where each domain corresponds to a specific subject, and improve model accuracy and robustness. However, when adapting to a specific target, the diverse nature of multiple source domains translates to a large shift between source and target data. State-of-the-art MSDA methods for FER address this domain shift by considering all the sources to adapt to the target representations. Nevertheless, adapting to a target subject presents significant challenges due to large distributional differences between source and target domains, often resulting in negative transfer. In addition, integrating all sources simultaneously increases computational costs and causes misalignment with the target. To address these issues, we propose a progressive MSDA approach that gradually introduces information from subjects based on their similarity to the target subject. This will ensure that only the most relevant sources from the target are selected, which helps avoid the negative transfer caused by dissimilar sources. We first exploit the closest sources to reduce the distribution shift with the target and then move towards the furthest while only considering the most relevant sources based on the predetermined threshold. Furthermore, to mitigate catastrophic forgetting caused by the incremental introduction of source subjects, we implemented a density-based memory mechanism that preserves the most relevant historical source samples for adaptation. Our experiments show the effectiveness of our proposed method on pain datasets: Biovid and UNBC-McMaster.
Via
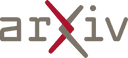
Apr 09, 2025
Abstract:The human face plays a central role in social communication, necessitating the use of performant computer vision tools for human-centered applications. We propose Face-LLaVA, a multimodal large language model for face-centered, in-context learning, including facial expression and attribute recognition. Additionally, Face-LLaVA is able to generate natural language descriptions that can be used for reasoning. Leveraging existing visual databases, we first developed FaceInstruct-1M, a face-centered database for instruction tuning MLLMs for face processing. We then developed a novel face-specific visual encoder powered by Face-Region Guided Cross-Attention that integrates face geometry with local visual features. We evaluated the proposed method across nine different datasets and five different face processing tasks, including facial expression recognition, action unit detection, facial attribute detection, age estimation and deepfake detection. Face-LLaVA achieves superior results compared to existing open-source MLLMs and competitive performance compared to commercial solutions. Our model output also receives a higher reasoning rating by GPT under a zero-shot setting across all the tasks. Both our dataset and model wil be released at https://face-llava.github.io to support future advancements in social AI and foundational vision-language research.
Via
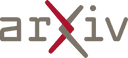
Apr 10, 2025
Abstract:Facial expression detection involves two interrelated tasks: spotting, which identifies the onset and offset of expressions, and recognition, which classifies them into emotional categories. Most existing methods treat these tasks separately using a two-step training pipelines. A spotting model first detects expression intervals. A recognition model then classifies the detected segments. However, this sequential approach leads to error propagation, inefficient feature learning, and suboptimal performance due to the lack of joint optimization of the two tasks. We propose FEDN, an end-to-end Facial Expression Detection Network that jointly optimizes spotting and recognition. Our model introduces a novel attention-based feature extraction module, incorporating segment attention and sliding window attention to improve facial feature learning. By unifying two tasks within a single network, we greatly reduce error propagation and enhance overall performance. Experiments on CASME}^2 and CASME^3 demonstrate state-of-the-art accuracy for both spotting and detection, underscoring the benefits of joint optimization for robust facial expression detection in long videos.
Via
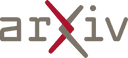
Mar 31, 2025
Abstract:Recent advances in generative AI have been driven by alignment techniques such as reinforcement learning from human feedback (RLHF). RLHF and related techniques typically involve constructing a dataset of binary or ranked choice human preferences and subsequently fine-tuning models to align with these preferences. This paper shifts the focus to understanding the preferences encoded in such datasets and identifying common human preferences. We find that a small subset of 21 preference categories (selected from a set of nearly 5,000 distinct preferences) captures >89% of preference variation across individuals. This small set of preferences is analogous to a canonical basis of human preferences, similar to established findings that characterize human variation in psychology or facial recognition studies. Through both synthetic and empirical evaluations, we confirm that our low-rank, canonical set of human preferences generalizes across the entire dataset and within specific topics. We further demonstrate our preference basis' utility in model evaluation, where our preference categories offer deeper insights into model alignment, and in model training, where we show that fine-tuning on preference-defined subsets successfully aligns the model accordingly.
* 25 pages, 11 figures
Via
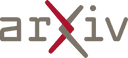
Mar 26, 2025
Abstract:This study investigates the key characteristics and suitability of widely used Facial Expression Recognition (FER) datasets for training deep learning models. In the field of affective computing, FER is essential for interpreting human emotions, yet the performance of FER systems is highly contingent on the quality and diversity of the underlying datasets. To address this issue, we compiled and analyzed 24 FER datasets, including those targeting specific age groups such as children, adults, and the elderly, and processed them through a comprehensive normalization pipeline. In addition, we enriched the datasets with automatic annotations for age and gender, enabling a more nuanced evaluation of their demographic properties. To further assess dataset efficacy, we introduce three novel metricsLocal, Global, and Paired Similarity, which quantitatively measure dataset difficulty, generalization capability, and cross-dataset transferability. Benchmark experiments using state-of-the-art neural networks reveal that large-scale, automatically collected datasets (e.g., AffectNet, FER2013) tend to generalize better, despite issues with labeling noise and demographic biases, whereas controlled datasets offer higher annotation quality but limited variability. Our findings provide actionable recommendations for dataset selection and design, advancing the development of more robust, fair, and effective FER systems.
Via
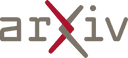
Apr 01, 2025
Abstract:Facial recognition systems in real-world scenarios are susceptible to both digital and physical attacks. Previous methods have attempted to achieve classification by learning a comprehensive feature space. However, these methods have not adequately accounted for the inherent characteristics of physical and digital attack data, particularly the large intra class variation in attacks and the small inter-class variation between live and fake faces. To address these limitations, we propose the Fine-Grained MoE with Class-Aware Regularization CLIP framework (FG-MoE-CLIP-CAR), incorporating key improvements at both the feature and loss levels. At the feature level, we employ a Soft Mixture of Experts (Soft MoE) architecture to leverage different experts for specialized feature processing. Additionally, we refine the Soft MoE to capture more subtle differences among various types of fake faces. At the loss level, we introduce two constraint modules: the Disentanglement Module (DM) and the Cluster Distillation Module (CDM). The DM enhances class separability by increasing the distance between the centers of live and fake face classes. However, center-to-center constraints alone are insufficient to ensure distinctive representations for individual features. Thus, we propose the CDM to further cluster features around their respective class centers while maintaining separation from other classes. Moreover, specific attacks that significantly deviate from common attack patterns are often overlooked. To address this issue, our distance calculation prioritizes more distant features. Experimental results on two unified physical-digital attack datasets demonstrate that the proposed method achieves state-of-the-art (SOTA) performance.
* 9 pages, 5 figures, accepted by AAAI-2025 (Oral)
Via
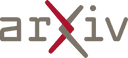