What is cancer detection? Cancer detection using Artificial Intelligence (AI) involves leveraging advanced machine learning algorithms and techniques to identify and diagnose cancer from various medical data sources. The goal is to enhance early detection, improve diagnostic accuracy, and potentially reduce the need for invasive procedures.
Papers and Code
May 28, 2025
Abstract:Gastrointestinal (GI) diseases represent a clinically significant burden, necessitating precise diagnostic approaches to optimize patient outcomes. Conventional histopathological diagnosis, heavily reliant on the subjective interpretation of pathologists, suffers from limited reproducibility and diagnostic variability. To overcome these limitations and address the lack of pathology-specific foundation models for GI diseases, we develop Digepath, a specialized foundation model for GI pathology. Our framework introduces a dual-phase iterative optimization strategy combining pretraining with fine-screening, specifically designed to address the detection of sparsely distributed lesion areas in whole-slide images. Digepath is pretrained on more than 353 million image patches from over 200,000 hematoxylin and eosin-stained slides of GI diseases. It attains state-of-the-art performance on 33 out of 34 tasks related to GI pathology, including pathological diagnosis, molecular prediction, gene mutation prediction, and prognosis evaluation, particularly in diagnostically ambiguous cases and resolution-agnostic tissue classification.We further translate the intelligent screening module for early GI cancer and achieve near-perfect 99.6% sensitivity across 9 independent medical institutions nationwide. The outstanding performance of Digepath highlights its potential to bridge critical gaps in histopathological practice. This work not only advances AI-driven precision pathology for GI diseases but also establishes a transferable paradigm for other pathology subspecialties.
Via
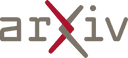
May 23, 2025
Abstract:Lung cancer has been one of the major threats across the world with the highest mortalities. Computer-aided detection (CAD) can help in early detection and thus can help increase the survival rate. Accurate lung parenchyma segmentation (to include the juxta-pleural nodules) and lung nodule segmentation, the primary symptom of lung cancer, play a crucial role in the overall accuracy of the Lung CAD pipeline. Lung nodule segmentation is quite challenging because of the diverse nodule types and other inhibit structures present within the lung lobes. Traditional machine/deep learning methods suffer from generalization and robustness. Recent Vision Language Models/Foundation Models perform well on the anatomical level, but they suffer on fine-grained segmentation tasks, and their semi-automatic nature limits their effectiveness in real-time clinical scenarios. In this paper, we propose a novel method for accurate 3D segmentation of lung parenchyma and lung nodules. The proposed architecture is an attention-based network with residual blocks at each encoder-decoder state. Max pooling is replaced by strided convolutions at the encoder, and trilinear interpolation is replaced by transposed convolutions at the decoder to maximize the number of learnable parameters. Dilated convolutions at each encoder-decoder stage allow the model to capture the larger context without increasing computational costs. The proposed method has been evaluated extensively on one of the largest publicly available datasets, namely LUNA16, and is compared with recent notable work in the domain using standard performance metrics like Dice score, IOU, etc. It can be seen from the results that the proposed method achieves better performance than state-of-the-art methods. The source code, datasets, and pre-processed data can be accessed using the link: https://github.com/EMeRALDsNRPU/Attention-Based-3D-ResUNet.
Via
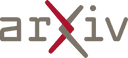
May 14, 2025
Abstract:Lung cancer is a leading cause of cancer-related deaths globally, where early detection and accurate diagnosis are critical for improving survival rates. While deep learning, particularly convolutional neural networks (CNNs), has revolutionized medical image analysis by detecting subtle patterns indicative of early-stage lung cancer, its adoption faces challenges. These models are often computationally expensive and require significant resources, making them unsuitable for resource constrained environments. Additionally, their lack of transparency hinders trust and broader adoption in sensitive fields like healthcare. Knowledge distillation addresses these challenges by transferring knowledge from large, complex models (teachers) to smaller, lightweight models (students). We propose a knowledge distillation-based approach for lung cancer detection, incorporating explainable AI (XAI) techniques to enhance model transparency. Eight CNNs, including ResNet50, EfficientNetB0, EfficientNetB3, and VGG16, are evaluated as teacher models. We developed and trained a lightweight student model, Distilled Custom Student Network (DCSNet) using ResNet50 as the teacher. This approach not only ensures high diagnostic performance in resource-constrained settings but also addresses transparency concerns, facilitating the adoption of AI-driven diagnostic tools in healthcare.
Via
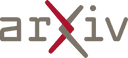
May 22, 2025
Abstract:Total Body Photography (TBP) is becoming a useful screening tool for patients at high risk for skin cancer. While much progress has been made, existing TBP systems can be further improved for automatic detection and analysis of suspicious skin lesions, which is in part related to the resolution and sharpness of acquired images. This paper proposes a novel shape-aware TBP system automatically capturing full-body images while optimizing image quality in terms of resolution and sharpness over the body surface. The system uses depth and RGB cameras mounted on a 360-degree rotary beam, along with 3D body shape estimation and an in-focus surface optimization method to select the optimal focus distance for each camera pose. This allows for optimizing the focused coverage over the complex 3D geometry of the human body given the calibrated camera poses. We evaluate the effectiveness of the system in capturing high-fidelity body images. The proposed system achieves an average resolution of 0.068 mm/pixel and 0.0566 mm/pixel with approximately 85% and 95% of surface area in-focus, evaluated on simulation data of diverse body shapes and poses as well as a real scan of a mannequin respectively. Furthermore, the proposed shape-aware focus method outperforms existing focus protocols (e.g. auto-focus). We believe the high-fidelity imaging enabled by the proposed system will improve automated skin lesion analysis for skin cancer screening.
* Accepted to JBHI
Via
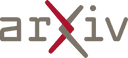
May 18, 2025
Abstract:Prostate cancer is one of the most common and lethal cancers among men, making its early detection critically important. Although ultrasound imaging offers greater accessibility and cost-effectiveness compared to MRI, traditional transrectal ultrasound methods suffer from low sensitivity, especially in detecting anteriorly located tumors. Ultrasound computed tomography provides quantitative tissue characterization, but its clinical implementation faces significant challenges, particularly under anatomically constrained limited-angle acquisition conditions specific to prostate imaging. To address these unmet needs, we introduce OpenPros, the first large-scale benchmark dataset explicitly developed for limited-view prostate USCT. Our dataset includes over 280,000 paired samples of realistic 2D speed-of-sound (SOS) phantoms and corresponding ultrasound full-waveform data, generated from anatomically accurate 3D digital prostate models derived from real clinical MRI/CT scans and ex vivo ultrasound measurements, annotated by medical experts. Simulations are conducted under clinically realistic configurations using advanced finite-difference time-domain and Runge-Kutta acoustic wave solvers, both provided as open-source components. Through comprehensive baseline experiments, we demonstrate that state-of-the-art deep learning methods surpass traditional physics-based approaches in both inference efficiency and reconstruction accuracy. Nevertheless, current deep learning models still fall short of delivering clinically acceptable high-resolution images with sufficient accuracy. By publicly releasing OpenPros, we aim to encourage the development of advanced machine learning algorithms capable of bridging this performance gap and producing clinically usable, high-resolution, and highly accurate prostate ultrasound images. The dataset is publicly accessible at https://open-pros.github.io/.
Via
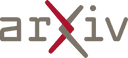
May 14, 2025
Abstract:Research projects, including those focused on cancer, rely on the manual extraction of information from clinical reports. This process is time-consuming and prone to errors, limiting the efficiency of data-driven approaches in healthcare. To address these challenges, Natural Language Processing (NLP) offers an alternative for automating the extraction of relevant data from electronic health records (EHRs). In this study, we focus on lung and breast cancer due to their high incidence and the significant impact they have on public health. Early detection and effective data management in both types of cancer are crucial for improving patient outcomes. To enhance the accuracy and efficiency of data extraction, we utilized GMV's NLP tool uQuery, which excels at identifying relevant entities in clinical texts and converting them into standardized formats such as SNOMED and OMOP. uQuery not only detects and classifies entities but also associates them with contextual information, including negated entities, temporal aspects, and patient-related details. In this work, we explore the use of NLP techniques, specifically Named Entity Recognition (NER), to automatically identify and extract key clinical information from EHRs related to these two cancers. A dataset from Health Research Institute Hospital La Fe (IIS La Fe), comprising 200 annotated breast cancer and 400 lung cancer reports, was used, with eight clinical entities manually labeled using the Doccano platform. To perform NER, we fine-tuned the bsc-bio-ehr-en3 model, a RoBERTa-based biomedical linguistic model pre-trained in Spanish. Fine-tuning was performed using the Transformers architecture, enabling accurate recognition of clinical entities in these cancer types. Our results demonstrate strong overall performance, particularly in identifying entities like MET and PAT, although challenges remain with less frequent entities like EVOL.
Via
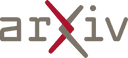
May 23, 2025
Abstract:Background: Accurate MRI-based identification of extramural vascular invasion (EVI) and mesorectal fascia invasion (MFI) is pivotal for risk-stratified management of rectal cancer, yet visual assessment is subjective and vulnerable to inter-institutional variability. Purpose: To develop and externally evaluate a multicenter, foundation-model-driven framework that automatically classifies EVI and MFI on axial and sagittal T2-weighted MRI. Methods: This retrospective study used 331 pre-treatment rectal cancer MRI examinations from three European hospitals. After TotalSegmentator-guided rectal patch extraction, a self-supervised frequency-domain harmonization pipeline was trained to minimize scanner-related contrast shifts. Four classifiers were compared: ResNet50, SeResNet, the universal biomedical pretrained transformer (UMedPT) with a lightweight MLP head, and a logistic-regression variant using frozen UMedPT features (UMedPT_LR). Results: UMedPT_LR achieved the best EVI detection when axial and sagittal features were fused (AUC = 0.82; sensitivity = 0.75; F1 score = 0.73), surpassing the Chaimeleon Grand-Challenge winner (AUC = 0.74). The highest MFI performance was attained by UMedPT on axial harmonized images (AUC = 0.77), surpassing the Chaimeleon Grand-Challenge winner (AUC = 0.75). Frequency-domain harmonization improved MFI classification but variably affected EVI performance. Conventional CNNs (ResNet50, SeResNet) underperformed, especially in F1 score and balanced accuracy. Conclusion: These findings demonstrate that combining foundation model features, harmonization, and multi-view fusion significantly enhances diagnostic performance in rectal MRI.
* 22 pages, 8 figures
Via
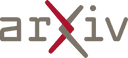
May 08, 2025
Abstract:Colorectal cancer is one of the deadliest cancers today, but it can be prevented through early detection of malignant polyps in the colon, primarily via colonoscopies. While this method has saved many lives, human error remains a significant challenge, as missing a polyp could have fatal consequences for the patient. Deep learning (DL) polyp detectors offer a promising solution. However, existing DL polyp detectors often mistake white light reflections from the endoscope for polyps, which can lead to false positives.To address this challenge, in this paper, we propose a novel data augmentation approach that artificially adds more white light reflections to create harder training scenarios. Specifically, we first generate a bank of artificial lights using the training dataset. Then we find the regions of the training images that we should not add these artificial lights on. Finally, we propose a sliding window method to add the artificial light to the areas that fit of the training images, resulting in augmented images. By providing the model with more opportunities to make mistakes, we hypothesize that it will also have more chances to learn from those mistakes, ultimately improving its performance in polyp detection. Experimental results demonstrate the effectiveness of our new data augmentation method.
* 5 pages, 4 Figures, paper accepted by the ISBI (International
Symposium on Biomedical Imaging) 2025 Conference
Via
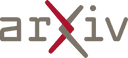
May 13, 2025
Abstract:The rising incidence of skin cancer, coupled with limited public awareness and a shortfall in clinical expertise, underscores an urgent need for advanced diagnostic aids. Artificial Intelligence (AI) has emerged as a promising tool in this domain, particularly for distinguishing malignant from benign skin lesions. Leveraging publicly available datasets of skin lesions, researchers have been developing AI-based diagnostic solutions. However, the integration of such computer systems in clinical settings is still nascent. This study aims to bridge this gap by employing a fusion of image processing techniques and machine learning algorithms, specifically neuro-fuzzy and colonial competition approaches. Applied to dermoscopic images from the ISIC database, our method achieved a notable accuracy of 94% on a dataset of 560 images. These results underscore the potential of our approach in aiding clinicians in the early detection of melanoma, thereby contributing significantly to skin cancer diagnostics.
* Proceedings of the 2024 7th International Conference on
Information and Computer Technologies, pages 166-172, IEEE, March 2024
* 7 pages, 10 figures. Accepted at the 2nd Asia Pacific Computer
Systems Conference (APCS 2024), March 15-17, 2024
Via
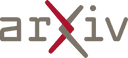
May 14, 2025
Abstract:The assessment of imaging biomarkers is critical for advancing precision medicine and improving disease characterization. Despite the availability of methods to derive disease heterogeneity metrics in imaging studies, a robust framework for evaluating measurement uncertainty remains underdeveloped. To address this gap, we propose a novel Bayesian framework to assess the precision of disease heterogeneity measures in biomarker studies. Our approach extends traditional methods for evaluating biomarker precision by providing greater flexibility in statistical assumptions and enabling the analysis of biomarkers beyond univariate or multivariate normally-distributed variables. Using Hamiltonian Monte Carlo sampling, the framework supports both, for example, normally-distributed and Dirichlet-Multinomial distributed variables, enabling the derivation of posterior distributions for biomarker parameters under diverse model assumptions. Designed to be broadly applicable across various imaging modalities and biomarker types, the framework builds a foundation for generalizing reproducible and objective biomarker evaluation. To demonstrate utility, we apply the framework to whole-body diffusion-weighted MRI (WBDWI) to assess heterogeneous therapeutic responses in metastatic bone disease. Specifically, we analyze data from two patient studies investigating treatments for metastatic castrate-resistant prostate cancer (mCRPC). Our results reveal an approximately 70% response rate among individual tumors across both studies, objectively characterizing differential responses to systemic therapies and validating the clinical relevance of the proposed methodology. This Bayesian framework provides a powerful tool for advancing biomarker research across diverse imaging-based studies while offering valuable insights into specific clinical applications, such as mCRPC treatment response.
Via
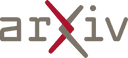