What is autonomous cars? Autonomous cars are self-driving vehicles that use artificial intelligence (AI) and sensors to navigate and operate without human intervention, using high-resolution cameras and lidars that detect what happens in the car's immediate surroundings. They have the potential to revolutionize transportation by improving safety, efficiency, and accessibility.
Papers and Code
Jan 29, 2025
Abstract:New challenges on transport systems are emerging due to the advances that the current paradigm is experiencing. The breakthrough of the autonomous car brings concerns about ride comfort, while the pollution concerns have arisen in recent years. In the model of automated automobiles, drivers are expected to become passengers, so, they will be more prone to suffer from ride discomfort or motion sickness. Conversely, the eco-driving implications should not be set aside because of the influence of pollution on climate and people's health. For that reason, a joint assessment of the aforementioned points would have a positive impact. Thus, this work presents a self-organised map-based solution to assess ride comfort features of individuals considering their driving style from the viewpoint of eco-driving. For this purpose, a previously acquired dataset from an instrumented car was used to classify drivers regarding the causes of their lack of ride comfort and eco-friendliness. Once drivers are classified regarding their driving style, natural-language-based recommendations are proposed to increase the engagement with the system. Hence, potential improvements of up to the 57.7% for ride comfort evaluation parameters, as well as up to the 47.1% in greenhouse-gasses emissions are expected to be reached.
Via
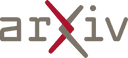
Jan 29, 2025
Abstract:Advanced driving assistance systems (ADAS) are primarily designed to increase driving safety and reduce traffic congestion without paying too much attention to passenger comfort or motion sickness. However, in view of autonomous cars, and taking into account that the lack of comfort and motion sickness increase in passengers, analysis from a comfort perspective is essential in the future car investigation. The aim of this work is to study in detail how passenger's comfort evaluation parameters vary depending on the driving style, car or road. The database used has been developed by compiling the accelerations suffered by passengers when three drivers cruise two different vehicles on different types of routes. In order to evaluate both comfort and motion sickness, first, the numerical values of the main comfort evaluation variables reported in the literature have been analyzed. Moreover, a complementary statistical analysis of probability density and a power spectral analysis are performed. Finally, quantitative results are compared with passenger qualitative feedback. The results show the high dependence of comfort evaluation variables' value with the road type. In addition, it has been demonstrated that the driving style and vehicle dynamics amplify or attenuate those values. Additionally, it has been demonstrated that contributions from longitudinal and lateral accelerations have a much greater effect in the lack of comfort than vertical ones. Finally, based on the concrete results obtained, a new experimental campaign is proposed.
Via
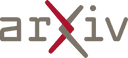
Feb 23, 2025
Abstract:As automated vehicles enter public roads, safety in a near-infinite number of driving scenarios becomes one of the major concerns for the widespread adoption of fully autonomous driving. The ability to detect anomalous situations outside of the operational design domain is a key component in self-driving cars, enabling us to mitigate the impact of abnormal ego behaviors and to realize trustworthy driving systems. On-road anomaly detection in egocentric videos remains a challenging problem due to the difficulties introduced by complex and interactive scenarios. We conduct a holistic analysis of common on-road anomaly patterns, from which we propose three unsupervised anomaly detection experts: a scene expert that focuses on frame-level appearances to detect abnormal scenes and unexpected scene motions; an interaction expert that models normal relative motions between two road participants and raises alarms whenever anomalous interactions emerge; and a behavior expert which monitors abnormal behaviors of individual objects by future trajectory prediction. To combine the strengths of all the modules, we propose an expert ensemble (Xen) using a Kalman filter, in which the final anomaly score is absorbed as one of the states and the observations are generated by the experts. Our experiments employ a novel evaluation protocol for realistic model performance, demonstrate superior anomaly detection performance than previous methods, and show that our framework has potential in classifying anomaly types using unsupervised learning on a large-scale on-road anomaly dataset.
* Accepted by International Journal of Robotics Research (IJRR)
Via
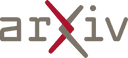
Feb 10, 2025
Abstract:Self-driving cars relying solely on ego-centric perception face limitations in sensing, often failing to detect occluded, faraway objects. Collaborative autonomous driving (CAV) seems like a promising direction, but collecting data for development is non-trivial. It requires placing multiple sensor-equipped agents in a real-world driving scene, simultaneously! As such, existing datasets are limited in locations and agents. We introduce a novel surrogate to the rescue, which is to generate realistic perception from different viewpoints in a driving scene, conditioned on a real-world sample - the ego-car's sensory data. This surrogate has huge potential: it could potentially turn any ego-car dataset into a collaborative driving one to scale up the development of CAV. We present the very first solution, using a combination of simulated collaborative data and real ego-car data. Our method, Transfer Your Perspective (TYP), learns a conditioned diffusion model whose output samples are not only realistic but also consistent in both semantics and layouts with the given ego-car data. Empirical results demonstrate TYP's effectiveness in aiding in a CAV setting. In particular, TYP enables us to (pre-)train collaborative perception algorithms like early and late fusion with little or no real-world collaborative data, greatly facilitating downstream CAV applications.
Via
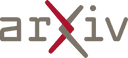
Jan 19, 2025
Abstract:3D car modeling is crucial for applications in autonomous driving systems, virtual and augmented reality, and gaming. However, due to the distinctive properties of cars, such as highly reflective and transparent surface materials, existing methods often struggle to achieve accurate 3D car reconstruction.To address these limitations, we propose Car-GS, a novel approach designed to mitigate the effects of specular highlights and the coupling of RGB and geometry in 3D geometric and shading reconstruction (3DGS). Our method incorporates three key innovations: First, we introduce view-dependent Gaussian primitives to effectively model surface reflections. Second, we identify the limitations of using a shared opacity parameter for both image rendering and geometric attributes when modeling transparent objects. To overcome this, we assign a learnable geometry-specific opacity to each 2D Gaussian primitive, dedicated solely to rendering depth and normals. Third, we observe that reconstruction errors are most prominent when the camera view is nearly orthogonal to glass surfaces. To address this issue, we develop a quality-aware supervision module that adaptively leverages normal priors from a pre-trained large-scale normal model.Experimental results demonstrate that Car-GS achieves precise reconstruction of car surfaces and significantly outperforms prior methods. The project page is available at https://lcc815.github.io/Car-GS.
Via
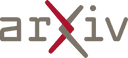
Feb 21, 2025
Abstract:Vehicle make and model recognition (VMMR) is a crucial component of the Intelligent Transport System, garnering significant attention in recent years. VMMR has been widely utilized for detecting suspicious vehicles, monitoring urban traffic, and autonomous driving systems. The complexity of VMMR arises from the subtle visual distinctions among vehicle models and the wide variety of classes produced by manufacturers. Convolutional Neural Networks (CNNs), a prominent type of deep learning model, have been extensively employed in various computer vision tasks, including VMMR, yielding remarkable results. As VMMR is a fine-grained classification problem, it primarily faces inter-class similarity and intra-class variation challenges. In this study, we implement an attention module to address these challenges and enhance the model's focus on critical areas containing distinguishing features. This module, which does not increase the parameters of the original model, generates three-dimensional (3-D) attention weights to refine the feature map. Our proposed model integrates the attention module into two different locations within the middle section of a convolutional model, where the feature maps from these sections offer sufficient information about the input frames without being overly detailed or overly coarse. The performance of our proposed model, along with state-of-the-art (SOTA) convolutional and transformer-based models, was evaluated using the Stanford Cars dataset. Our proposed model achieved the highest accuracy, 90.69\%, among the compared models.
Via
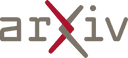
Dec 23, 2024
Abstract:Validating the behavior of autonomous Cyber-Physical Systems (CPS) and Artificial Intelligence (AI) agents, which rely on automated controllers, is an objective of great importance. In recent years, Neural-Network (NN) controllers have been demonstrating great promise. Unfortunately, such learned controllers are often not certified and can cause the system to suffer from unpredictable or unsafe behavior. To mitigate this issue, a great effort has been dedicated to automated verification of systems. Specifically, works in the category of ``black-box testing'' rely on repeated system simulations to find a falsifying counterexample of a system run that violates a specification. As running high-fidelity simulations is computationally demanding, the goal of falsification approaches is to minimize the simulation effort (NN inference queries) needed to return a falsifying example. This often proves to be a great challenge, especially when the tested controller is well-trained. This work contributes a novel falsification approach for autonomous systems under formal specification operating in uncertain environments. We are especially interested in CPS operating in rich, semantically-defined, open environments, which yield high-dimensional, simulation-dependent sensor observations. Our approach introduces a novel reformulation of the falsification problem as the problem of planning a trajectory for a ``meta-system,'' which wraps and encapsulates the examined system; we call this approach: meta-planning. This formulation can be solved with standard sampling-based motion-planning techniques (like RRT) and can gradually integrate domain knowledge to improve the search. We support the suggested approach with an experimental study on falsification of an obstacle-avoiding autonomous car with a NN controller, where meta-planning demonstrates superior performance over alternative approaches.
Via
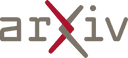
Dec 09, 2024
Abstract:Machine Learning (ML) models, such as deep neural networks, are widely applied in autonomous systems to perform complex perception tasks. New dependability challenges arise when ML predictions are used in safety-critical applications, like autonomous cars and surgical robots. Thus, the use of fault tolerance mechanisms, such as safety monitors, is essential to ensure the safe behavior of the system despite the occurrence of faults. This paper presents an extensive literature review on safety monitoring of perception functions using ML in a safety-critical context. In this review, we structure the existing literature to highlight key factors to consider when designing such monitors: threat identification, requirements elicitation, detection of failure, reaction, and evaluation. We also highlight the ongoing challenges associated with safety monitoring and suggest directions for future research.
* 25 pages, 2 figures
Via
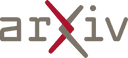
Dec 12, 2024
Abstract:Autonomous racing extends beyond the challenge of controlling a racecar at its physical limits. Professional racers employ strategic maneuvers to outwit other competing opponents to secure victory. While modern control algorithms can achieve human-level performance by computing offline racing lines for single-car scenarios, research on real-time algorithms for multi-car autonomous racing is limited. To bridge this gap, we develop game-theoretic modeling framework that incorporates the competitive aspect of autonomous racing like overtaking and blocking through a novel policy parametrization, while operating the car at its limit. Furthermore, we propose an algorithmic approach to compute the (approximate) Nash equilibrium strategy, which represents the optimal approach in the presence of competing agents. Specifically, we introduce an algorithm inspired by recently introduced framework of dynamic near-potential function, enabling real-time computation of the Nash equilibrium. Our approach comprises two phases: offline and online. During the offline phase, we use simulated racing data to learn a near-potential function that approximates utility changes for agents. This function facilitates the online computation of approximate Nash equilibria by maximizing its value. We evaluate our method in a head-to-head 3-car racing scenario, demonstrating superior performance compared to several existing baselines.
Via
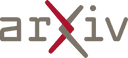
Dec 02, 2024
Abstract:Spatial understanding of the semantics of the surroundings is a key capability needed by autonomous cars to enable safe driving decisions. Recently, purely vision-based solutions have gained increasing research interest. In particular, approaches extracting a bird's eye view (BEV) from multiple cameras have demonstrated great performance for spatial understanding. This paper addresses the dependency on learned positional encodings to correlate image and BEV feature map elements for transformer-based methods. We propose leveraging epipolar geometric constraints to model the relationship between cameras and the BEV by Epipolar Attention Fields. They are incorporated into the attention mechanism as a novel attribution term, serving as an alternative to learned positional encodings. Experiments show that our method EAFormer outperforms previous BEV approaches by 2% mIoU for map semantic segmentation and exhibits superior generalization capabilities compared to implicitly learning the camera configuration.
* Accepted at WACV 2025
Via
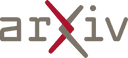