What is autonomous cars? Autonomous cars are self-driving vehicles that use artificial intelligence (AI) and sensors to navigate and operate without human intervention, using high-resolution cameras and lidars that detect what happens in the car's immediate surroundings. They have the potential to revolutionize transportation by improving safety, efficiency, and accessibility.
Papers and Code
Jun 12, 2024
Abstract:A fundamental task in mobile robotics is keeping an intelligent agent under surveillance with an autonomous robot as it travels in the environment. This work studies a version of that problem involving one of the most popular vehicle platforms in robotics. In particular, we consider two identical Dubins cars moving on a plane without obstacles. One of them plays as the pursuer, and it is equipped with a limited field-of-view detection region modeled as a semi-infinite cone with its apex at the pursuer's position. The pursuer aims to maintain the other Dubins car, which plays as the evader, as much time as possible inside its detection region. On the contrary, the evader wants to escape as soon as possible. In this work, employing differential game theory, we find the time-optimal motion strategies near the game's end. The analysis of those trajectories reveals the existence of at least two singular surfaces: a Transition Surface and an Evader's Universal Surface. We also found that the barrier's standard construction produces a surface that partially lies outside the playing space and fails to define a closed region, implying that an additional procedure is required to determine all configurations where the evader escapes.
* 8 pages, 9 figures
Via
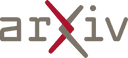
Aug 22, 2024
Abstract:Social norm is defined as a shared standard of acceptable behavior in a society. The emergence of social norms fosters coordination among agents without any hard-coded rules, which is crucial for the large-scale deployment of AVs in an intelligent transportation system. This paper explores the application of LLMs in understanding and modeling social norms in autonomous driving games. We introduce LLMs into autonomous driving games as intelligent agents who make decisions according to text prompts. These agents are referred to as LLM-based agents. Our framework involves LLM-based agents playing Markov games in a multi-agent system (MAS), allowing us to investigate the emergence of social norms among individual agents. We aim to identify social norms by designing prompts and utilizing LLMs on textual information related to the environment setup and the observations of LLM-based agents. Using the OpenAI Chat API powered by GPT-4.0, we conduct experiments to simulate interactions and evaluate the performance of LLM-based agents in two driving scenarios: unsignalized intersection and highway platoon. The results show that LLM-based agents can handle dynamically changing environments in Markov games, and social norms evolve among LLM-based agents in both scenarios. In the intersection game, LLM-based agents tend to adopt a conservative driving policy when facing a potential car crash. The advantage of LLM-based agents in games lies in their strong operability and analyzability, which facilitate experimental design.
Via
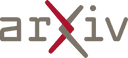
May 28, 2024
Abstract:Unveiling an empty street from crowded observations captured by in-car cameras is crucial for autonomous driving. However, removing all temporary static objects, such as stopped vehicles and standing pedestrians, presents a significant challenge. Unlike object-centric 3D inpainting, which relies on thorough observation in a small scene, street scenes involve long trajectories that differ from previous 3D inpainting tasks. The camera-centric moving environment of captured videos further complicates the task due to the limited degree and time duration of object observation. To address these obstacles, we introduce StreetUnveiler to reconstruct an empty street. StreetUnveiler learns a 3D representation of the empty street from crowded observations. Our representation is based on the hard-label semantic 2D Gaussian Splatting (2DGS) for its scalability and ability to identify Gaussians to be removed. We inpaint rendered image after removing unwanted Gaussians to provide pseudo-labels and subsequently re-optimize the 2DGS. Given its temporal continuous movement, we divide the empty street scene into observed, partial-observed, and unobserved regions, which we propose to locate through a rendered alpha map. This decomposition helps us to minimize the regions that need to be inpainted. To enhance the temporal consistency of the inpainting, we introduce a novel time-reversal framework to inpaint frames in reverse order and use later frames as references for earlier frames to fully utilize the long-trajectory observations. Our experiments conducted on the street scene dataset successfully reconstructed a 3D representation of the empty street. The mesh representation of the empty street can be extracted for further applications. Project page and more visualizations can be found at: https://streetunveiler.github.io
Via
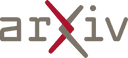
Jun 08, 2024
Abstract:This paper summarizes an autonomous driving project by musculoskeletal humanoids. The musculoskeletal humanoid, which mimics the human body in detail, has redundant sensors and a flexible body structure. These characteristics are suitable for motions with complex environmental contact, and the robot is expected to sit down on the car seat, step on the acceleration and brake pedals, and operate the steering wheel by both arms. We reconsider the developed hardware and software of the musculoskeletal humanoid Musashi in the context of autonomous driving. The respective components of autonomous driving are conducted using the benefits of the hardware and software. Finally, Musashi succeeded in the pedal and steering wheel operations with recognition.
* Accepted at IEEE Robotics and Automation Magazine
Via
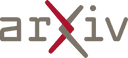
May 30, 2024
Abstract:Autonomous systems, such as self-driving cars, rely on reliable semantic environment perception for decision making. Despite great advances in video semantic segmentation, existing approaches ignore important inductive biases and lack structured and interpretable internal representations. In this work, we propose MCDS-VSS, a structured filter model that learns in a self-supervised manner to estimate scene geometry and ego-motion of the camera, while also estimating the motion of external objects. Our model leverages these representations to improve the temporal consistency of semantic segmentation without sacrificing segmentation accuracy. MCDS-VSS follows a prediction-fusion approach in which scene geometry and camera motion are first used to compensate for ego-motion, then residual flow is used to compensate motion of dynamic objects, and finally the predicted scene features are fused with the current features to obtain a temporally consistent scene segmentation. Our model parses automotive scenes into multiple decoupled interpretable representations such as scene geometry, ego-motion, and object motion. Quantitative evaluation shows that MCDS-VSS achieves superior temporal consistency on video sequences while retaining competitive segmentation performance.
Via
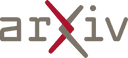
Jul 25, 2024
Abstract:Accurately and promptly predicting accidents among surrounding traffic agents from camera footage is crucial for the safety of autonomous vehicles (AVs). This task presents substantial challenges stemming from the unpredictable nature of traffic accidents, their long-tail distribution, the intricacies of traffic scene dynamics, and the inherently constrained field of vision of onboard cameras. To address these challenges, this study introduces a novel accident anticipation framework for AVs, termed CRASH. It seamlessly integrates five components: object detector, feature extractor, object-aware module, context-aware module, and multi-layer fusion. Specifically, we develop the object-aware module to prioritize high-risk objects in complex and ambiguous environments by calculating the spatial-temporal relationships between traffic agents. In parallel, the context-aware is also devised to extend global visual information from the temporal to the frequency domain using the Fast Fourier Transform (FFT) and capture fine-grained visual features of potential objects and broader context cues within traffic scenes. To capture a wider range of visual cues, we further propose a multi-layer fusion that dynamically computes the temporal dependencies between different scenes and iteratively updates the correlations between different visual features for accurate and timely accident prediction. Evaluated on real-world datasets--Dashcam Accident Dataset (DAD), Car Crash Dataset (CCD), and AnAn Accident Detection (A3D) datasets--our model surpasses existing top baselines in critical evaluation metrics like Average Precision (AP) and mean Time-To-Accident (mTTA). Importantly, its robustness and adaptability are particularly evident in challenging driving scenarios with missing or limited training data, demonstrating significant potential for application in real-world autonomous driving systems.
Via
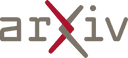
Jul 22, 2024
Abstract:To alleviate energy shortages and environmental impacts caused by transportation, this study introduces EcoFollower, a novel eco-car-following model developed using reinforcement learning (RL) to optimize fuel consumption in car-following scenarios. Employing the NGSIM datasets, the performance of EcoFollower was assessed in comparison with the well-established Intelligent Driver Model (IDM). The findings demonstrate that EcoFollower excels in simulating realistic driving behaviors, maintaining smooth vehicle operations, and closely matching the ground truth metrics of time-to-collision (TTC), headway, and comfort. Notably, the model achieved a significant reduction in fuel consumption, lowering it by 10.42\% compared to actual driving scenarios. These results underscore the capability of RL-based models like EcoFollower to enhance autonomous vehicle algorithms, promoting safer and more energy-efficient driving strategies.
Via
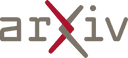
Jun 17, 2024
Abstract:Trajectory prediction forecasts nearby agents' moves based on their historical trajectories. Accurate trajectory prediction is crucial for autonomous vehicles. Existing attacks compromise the prediction model of a victim AV by directly manipulating the historical trajectory of an attacker AV, which has limited real-world applicability. This paper, for the first time, explores an indirect attack approach that induces prediction errors via attacks against the perception module of a victim AV. Although it has been shown that physically realizable attacks against LiDAR-based perception are possible by placing a few objects at strategic locations, it is still an open challenge to find an object location from the vast search space in order to launch effective attacks against prediction under varying victim AV velocities. Through analysis, we observe that a prediction model is prone to an attack focusing on a single point in the scene. Consequently, we propose a novel two-stage attack framework to realize the single-point attack. The first stage of prediction-side attack efficiently identifies, guided by the distribution of detection results under object-based attacks against perception, the state perturbations for the prediction model that are effective and velocity-insensitive. In the second stage of location matching, we match the feasible object locations with the found state perturbations. Our evaluation using a public autonomous driving dataset shows that our attack causes a collision rate of up to 63% and various hazardous responses of the victim AV. The effectiveness of our attack is also demonstrated on a real testbed car. To the best of our knowledge, this study is the first security analysis spanning from LiDAR-based perception to prediction in autonomous driving, leading to a realistic attack on prediction. To counteract the proposed attack, potential defenses are discussed.
* In Proceedings of the 33rd USENIX Security Symposium 2024
Via
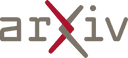
Jul 24, 2024
Abstract:Adversarial attacks against monocular depth estimation (MDE) systems pose significant challenges, particularly in safety-critical applications such as autonomous driving. Existing patch-based adversarial attacks for MDE are confined to the vicinity of the patch, making it difficult to affect the entire target. To address this limitation, we propose a physics-based adversarial attack on monocular depth estimation, employing a framework called Attack with Shape-Varying Patches (ASP), aiming to optimize patch content, shape, and position to maximize effectiveness. We introduce various mask shapes, including quadrilateral, rectangular, and circular masks, to enhance the flexibility and efficiency of the attack. Furthermore, we propose a new loss function to extend the influence of the patch beyond the overlapping regions. Experimental results demonstrate that our attack method generates an average depth error of 18 meters on the target car with a patch area of 1/9, affecting over 98\% of the target area.
Via
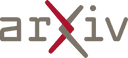
Jul 02, 2024
Abstract:In pursuit of autonomous vehicles, achieving human-like driving behavior is vital. This study introduces adaptive autopilot (AA), a unique framework utilizing constrained-deep reinforcement learning (C-DRL). AA aims to safely emulate human driving to reduce the necessity for driver intervention. Focusing on the car-following scenario, the process involves (i) extracting data from the highD natural driving study and categorizing it into three driving styles using a rule-based classifier; (ii) employing deep neural network (DNN) regressors to predict human-like acceleration across styles; and (iii) using C-DRL, specifically the soft actor-critic Lagrangian technique, to learn human-like safe driving policies. Results indicate effectiveness in each step, with the rule-based classifier distinguishing driving styles, the regressor model accurately predicting acceleration, outperforming traditional car-following models, and C-DRL agents learning optimal policies for humanlike driving across styles.
* 8 pages, 9 figures
Via
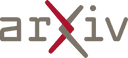