Jun 08, 2025
Abstract:Metaphors are pervasive in communication, making them crucial for natural language processing (NLP). Previous research on automatic metaphor processing predominantly relies on training data consisting of English samples, which often reflect Western European or North American biases. This cultural skew can lead to an overestimation of model performance and contributions to NLP progress. However, the impact of cultural bias on metaphor processing, particularly in multimodal contexts, remains largely unexplored. To address this gap, we introduce MultiMM, a Multicultural Multimodal Metaphor dataset designed for cross-cultural studies of metaphor in Chinese and English. MultiMM consists of 8,461 text-image advertisement pairs, each accompanied by fine-grained annotations, providing a deeper understanding of multimodal metaphors beyond a single cultural domain. Additionally, we propose Sentiment-Enriched Metaphor Detection (SEMD), a baseline model that integrates sentiment embeddings to enhance metaphor comprehension across cultural backgrounds. Experimental results validate the effectiveness of SEMD on metaphor detection and sentiment analysis tasks. We hope this work increases awareness of cultural bias in NLP research and contributes to the development of fairer and more inclusive language models. Our dataset and code are available at https://github.com/DUTIR-YSQ/MultiMM.
* This paper has been accepted to the 63rd Annual Meeting of the
Association for Computational Linguistics (ACL 2025), Main Conference
Via
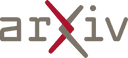