What is Recommendation? Recommendation is the task of providing personalized suggestions to users based on their preferences and behavior.
Papers and Code
Jun 07, 2025
Abstract:Sociotechnical systems, such as language technologies, frequently exhibit identity-based biases. These biases exacerbate the experiences of historically marginalized communities and remain understudied in low-resource contexts. While models and datasets specific to a language or with multilingual support are commonly recommended to address these biases, this paper empirically tests the effectiveness of such approaches in the context of gender, religion, and nationality-based identities in Bengali, a widely spoken but low-resourced language. We conducted an algorithmic audit of sentiment analysis models built on mBERT and BanglaBERT, which were fine-tuned using all Bengali sentiment analysis (BSA) datasets from Google Dataset Search. Our analyses showed that BSA models exhibit biases across different identity categories despite having similar semantic content and structure. We also examined the inconsistencies and uncertainties arising from combining pre-trained models and datasets created by individuals from diverse demographic backgrounds. We connected these findings to the broader discussions on epistemic injustice, AI alignment, and methodological decisions in algorithmic audits.
Via
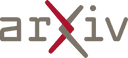
Jun 07, 2025
Abstract:Query suggestion plays a crucial role in enhancing user experience in e-commerce search systems by providing relevant query recommendations that align with users' initial input. This module helps users navigate towards personalized preference needs and reduces typing effort, thereby improving search experience. Traditional query suggestion modules usually adopt multi-stage cascading architectures, for making a well trade-off between system response time and business conversion. But they often suffer from inefficiencies and suboptimal performance due to inconsistent optimization objectives across stages. To address these, we propose OneSug, the first end-to-end generative framework for e-commerce query suggestion. OneSug incorporates a prefix2query representation enhancement module to enrich prefixes using semantically and interactively related queries to bridge content and business characteristics, an encoder-decoder generative model that unifies the query suggestion process, and a reward-weighted ranking strategy with behavior-level weights to capture fine-grained user preferences. Extensive evaluations on large-scale industry datasets demonstrate OneSug's ability for effective and efficient query suggestion. Furthermore, OneSug has been successfully deployed for the entire traffic on the e-commerce search engine in Kuaishou platform for over 1 month, with statistically significant improvements in user top click position (-9.33%), CTR (+2.01%), Order (+2.04%), and Revenue (+1.69%) over the online multi-stage strategy, showing great potential in e-commercial conversion.
* 11 pages, 8 figures, and 6 tables
Via
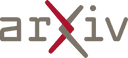
Jun 07, 2025
Abstract:Navigating healthcare systems can be complex and overwhelming, creating barriers for patients seeking timely and appropriate medical attention. In this paper, we introduce C-PATH (Conversational Patient Assistance and Triage in Healthcare), a novel conversational AI system powered by large language models (LLMs) designed to assist patients in recognizing symptoms and recommending appropriate medical departments through natural, multi-turn dialogues. C-PATH is fine-tuned on medical knowledge, dialogue data, and clinical summaries using a multi-stage pipeline built on the LLaMA3 architecture. A core contribution of this work is a GPT-based data augmentation framework that transforms structured clinical knowledge from DDXPlus into lay-person-friendly conversations, allowing alignment with patient communication norms. We also implement a scalable conversation history management strategy to ensure long-range coherence. Evaluation with GPTScore demonstrates strong performance across dimensions such as clarity, informativeness, and recommendation accuracy. Quantitative benchmarks show that C-PATH achieves superior performance in GPT-rewritten conversational datasets, significantly outperforming domain-specific baselines. C-PATH represents a step forward in the development of user-centric, accessible, and accurate AI tools for digital health assistance and triage.
* Accepted in IEEE ICDH 2025, 10 pages, 8 figures, 5 tables
Via
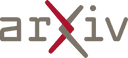
Jun 07, 2025
Abstract:The ACM Lifelog Search Challenge (LSC) is a venue that welcomes and compares systems that support the exploration of lifelog data, and in particular the retrieval of specific information, through an interactive competition format. This paper reviews the recent advances in interactive lifelog retrieval as demonstrated at the ACM LSC from 2022 to 2024. Through a detailed comparative analysis, we highlight key improvements across three main retrieval tasks: known-item search, question answering, and ad-hoc search. Our analysis identifies trends such as the widespread adoption of embedding-based retrieval methods (e.g., CLIP, BLIP), increased integration of large language models (LLMs) for conversational retrieval, and continued innovation in multimodal and collaborative search interfaces. We further discuss how specific retrieval techniques and user interface (UI) designs have impacted system performance, emphasizing the importance of balancing retrieval complexity with usability. Our findings indicate that embedding-driven approaches combined with LLMs show promise for lifelog retrieval systems. Likewise, improving UI design can enhance usability and efficiency. Additionally, we recommend reconsidering multi-instance system evaluations within the expert track to better manage variability in user familiarity and configuration effectiveness.
Via
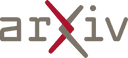
Jun 06, 2025
Abstract:With the rapid growth of fintech, personalized financial product recommendations have become increasingly important. Traditional methods like collaborative filtering or content-based models often fail to capture users' latent preferences and complex relationships. We propose a hybrid framework integrating large language models (LLMs) and graph neural networks (GNNs). A pre-trained LLM encodes text data (e.g., user reviews) into rich feature vectors, while a heterogeneous user-product graph models interactions and social ties. Through a tailored message-passing mechanism, text and graph information are fused within the GNN to jointly optimize embeddings. Experiments on public and real-world financial datasets show our model outperforms standalone LLM or GNN in accuracy, recall, and NDCG, with strong interpretability. This work offers new insights for personalized financial recommendations and cross-modal fusion in broader recommendation tasks.
Via
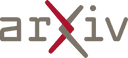
Jun 06, 2025
Abstract:Large language model (LLM) embeddings offer a promising new avenue for database query optimization. In this paper, we explore how pre-trained execution plan embeddings can guide SQL query execution without the need for additional model training. We introduce LLM-PM (LLM-based Plan Mapping), a framework that embeds the default execution plan of a query, finds its k nearest neighbors among previously executed plans, and recommends database hintsets based on neighborhood voting. A lightweight consistency check validates the selected hint, while a fallback mechanism searches the full hint space when needed. Evaluated on the JOB-CEB benchmark using OpenGauss, LLM-PM achieves an average speed-up of 21% query latency reduction. This work highlights the potential of LLM-powered embeddings to deliver practical improvements in query performance and opens new directions for training-free, embedding-based optimizer guidance systems.
* 18 pages, 5 figures
Via
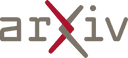
Jun 06, 2025
Abstract:Time-series forecasting is an essential task with wide real-world applications across domains. While recent advances in deep learning have enabled time-series forecasting models with accurate predictions, there remains considerable debate over which architectures and design components, such as series decomposition or normalization, are most effective under varying conditions. Existing benchmarks primarily evaluate models at a high level, offering limited insight into why certain designs work better. To mitigate this gap, we propose TimeRecipe, a unified benchmarking framework that systematically evaluates time-series forecasting methods at the module level. TimeRecipe conducts over 10,000 experiments to assess the effectiveness of individual components across a diverse range of datasets, forecasting horizons, and task settings. Our results reveal that exhaustive exploration of the design space can yield models that outperform existing state-of-the-art methods and uncover meaningful intuitions linking specific design choices to forecasting scenarios. Furthermore, we release a practical toolkit within TimeRecipe that recommends suitable model architectures based on these empirical insights. The benchmark is available at: https://github.com/AdityaLab/TimeRecipe.
* 46 pages, 1 figure, 28 tables
Via
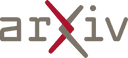
Jun 06, 2025
Abstract:Users of social media platforms based on recommendation systems (RecSys) (e.g. TikTok, X, YouTube) strategically interact with platform content to influence future recommendations. On some such platforms, users have been documented to form large-scale grassroots movements encouraging others to purposefully interact with algorithmically suppressed content in order to "boost" its recommendation; we term this behavior user altruism. To capture this behavior, we study a game between users and a RecSys, where users provide the RecSys (potentially manipulated) preferences over the contents available to them, and the RecSys -- limited by data and computation constraints -- creates a low-rank approximation preference matrix, and ultimately provides each user her (approximately) most-preferred item. We compare the users' social welfare under truthful preference reporting and under a class of strategies capturing user altruism. In our theoretical analysis, we provide sufficient conditions to ensure strict increases in user social welfare under user altruism, and provide an algorithm to find an effective altruistic strategy. Interestingly, we show that for commonly assumed recommender utility functions, effectively altruistic strategies also improve the utility of the RecSys! We show that our results are robust to several model misspecifications, thus strengthening our conclusions. Our theoretical analysis is complemented by empirical results of effective altruistic strategies on the GoodReads dataset, and an online survey on how real-world users behave altruistically in RecSys. Overall, our findings serve as a proof-of-concept of the reasons why traditional RecSys may incentivize users to form collectives and/or follow altruistic strategies when interacting with them.
Via
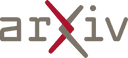
Jun 06, 2025
Abstract:Semantic ID-based recommendation models tokenize each item into a small number of discrete tokens that preserve specific semantics, leading to better performance, scalability, and memory efficiency. While recent models adopt a generative approach, they often suffer from inefficient inference due to the reliance on resource-intensive beam search and multiple forward passes through the neural sequence model. As a result, the length of semantic IDs is typically restricted (e.g. to just 4 tokens), limiting their expressiveness. To address these challenges, we propose RPG, a lightweight framework for semantic ID-based recommendation. The key idea is to produce unordered, long semantic IDs, allowing the model to predict all tokens in parallel. We train the model to predict each token independently using a multi-token prediction loss, directly integrating semantics into the learning objective. During inference, we construct a graph connecting similar semantic IDs and guide decoding to avoid generating invalid IDs. Experiments show that scaling up semantic ID length to 64 enables RPG to outperform generative baselines by an average of 12.6% on the NDCG@10, while also improving inference efficiency. Code is available at: https://github.com/facebookresearch/RPG_KDD2025.
* KDD 2025
Via
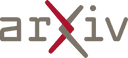
Jun 06, 2025
Abstract:Wheat management strategies play a critical role in determining yield. Traditional management decisions often rely on labour-intensive expert inspections, which are expensive, subjective and difficult to scale. Recently, Vision-Language Models (VLMs) have emerged as a promising solution to enable scalable, data-driven management support. However, due to a lack of domain-specific knowledge, directly applying VLMs to wheat management tasks results in poor quantification and reasoning capabilities, ultimately producing vague or even misleading management recommendations. In response, we propose WisWheat, a wheat-specific dataset with a three-layered design to enhance VLM performance on wheat management tasks: (1) a foundational pretraining dataset of 47,871 image-caption pairs for coarsely adapting VLMs to wheat morphology; (2) a quantitative dataset comprising 7,263 VQA-style image-question-answer triplets for quantitative trait measuring tasks; and (3) an Instruction Fine-tuning dataset with 4,888 samples targeting biotic and abiotic stress diagnosis and management plan for different phenological stages. Extensive experimental results demonstrate that fine-tuning open-source VLMs (e.g., Qwen2.5 7B) on our dataset leads to significant performance improvements. Specifically, the Qwen2.5 VL 7B fine-tuned on our wheat instruction dataset achieves accuracy scores of 79.2% and 84.6% on wheat stress and growth stage conversation tasks respectively, surpassing even general-purpose commercial models such as GPT-4o by a margin of 11.9% and 34.6%.
Via
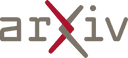