What is Object Detection? Object detection is a computer vision task in which the goal is to detect and locate objects of interest in an image or video. The task involves identifying the position and boundaries of objects in an image, and classifying the objects into different categories. It forms a crucial part of vision recognition, alongside image classification and retrieval.
Papers and Code
Jun 25, 2025
Abstract:The rapid advancement of generative artificial intelligence is producing fake remote sensing imagery (RSI) that is increasingly difficult to detect, potentially leading to erroneous intelligence, fake news, and even conspiracy theories. Existing forgery detection methods typically rely on single visual features to capture predefined artifacts, such as spatial-domain cues to detect forged objects like roads or buildings in RSI, or frequency-domain features to identify artifacts from up-sampling operations in adversarial generative networks (GANs). However, the nature of artifacts can significantly differ depending on geographic terrain, land cover types, or specific features within the RSI. Moreover, these complex artifacts evolve as generative models become more sophisticated. In short, over-reliance on a single visual cue makes existing forgery detectors struggle to generalize across diverse remote sensing data. This paper proposed a novel forgery detection framework called SFNet, designed to identify fake images in diverse remote sensing data by leveraging spatial and frequency domain features. Specifically, to obtain rich and comprehensive visual information, SFNet employs two independent feature extractors to capture spatial and frequency domain features from input RSIs. To fully utilize the complementary domain features, the domain feature mapping module and the hybrid domain feature refinement module(CBAM attention) of SFNet are designed to successively align and fuse the multi-domain features while suppressing redundant information. Experiments on three datasets show that SFNet achieves an accuracy improvement of 4%-15.18% over the state-of-the-art RS forgery detection methods and exhibits robust generalization capabilities. The code is available at https://github.com/GeoX-Lab/RSTI/tree/main/SFNet.
Via
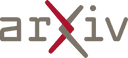
Jun 26, 2025
Abstract:Enforcing helmet regulations among motorcyclists is essential for enhancing road safety and ensuring the effectiveness of traffic management systems. However, automatic detection of helmet violations faces significant challenges due to environmental variability, camera angles, and inconsistencies in the data. These factors hinder reliable detection of motorcycles and riders and disrupt consistent object classification. To address these challenges, we propose VisionGuard, a synergistic multi-stage framework designed to overcome the limitations of frame-wise detectors, especially in scenarios with class imbalance and inconsistent annotations. VisionGuard integrates two key components: Adaptive Labeling and Contextual Expander modules. The Adaptive Labeling module is a tracking-based refinement technique that enhances classification consistency by leveraging a tracking algorithm to assign persistent labels across frames and correct misclassifications. The Contextual Expander module improves recall for underrepresented classes by generating virtual bounding boxes with appropriate confidence scores, effectively addressing the impact of data imbalance. Experimental results show that VisionGuard improves overall mAP by 3.1% compared to baseline detectors, demonstrating its effectiveness and potential for real-world deployment in traffic surveillance systems, ultimately promoting safety and regulatory compliance.
Via
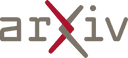
Jun 25, 2025
Abstract:Drones can inspect overhead power lines while they remain energized, significantly simplifying the inspection process. However, localizing a drone relative to all conductors using an onboard LiDAR sensor presents several challenges: (1) conductors provide minimal surface for LiDAR beams limiting the number of conductor points in a scan, (2) not all conductors are consistently detected, and (3) distinguishing LiDAR points corresponding to conductors from other objects, such as trees and pylons, is difficult. This paper proposes an estimation approach that minimizes the error between LiDAR measurements and a single geometric model representing the entire conductor array, rather than tracking individual conductors separately. Experimental results, using data from a power line drone inspection, demonstrate that this method achieves accurate tracking, with a solver converging under 50 ms per frame, even in the presence of partial observations, noise, and outliers. A sensitivity analysis shows that the estimation approach can tolerate up to twice as many outlier points as valid conductors measurements.
* Submitted to IEEE case 2025
Via
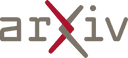
Jun 26, 2025
Abstract:Detectors often suffer from performance drop due to domain gap between training and testing data. Recent methods explore diffusion models applied to domain generalization (DG) and adaptation (DA) tasks, but still struggle with large inference costs and have not yet fully leveraged the capabilities of diffusion models. We propose to tackle these problems by extracting intermediate features from a single-step diffusion process, improving feature collection and fusion to reduce inference time by 75% while enhancing performance on source domains (i.e., Fitness). Then, we construct an object-centered auxiliary branch by applying box-masked images with class prompts to extract robust and domain-invariant features that focus on object. We also apply consistency loss to align the auxiliary and ordinary branch, balancing fitness and generalization while preventing overfitting and improving performance on target domains (i.e., Generalization). Furthermore, within a unified framework, standard detectors are guided by diffusion detectors through feature-level and object-level alignment on source domains (for DG) and unlabeled target domains (for DA), thereby improving cross-domain detection performance (i.e., Transferability). Our method achieves competitive results on 3 DA benchmarks and 5 DG benchmarks. Additionally, experiments on COCO generalization benchmark demonstrate that our method maintains significant advantages and show remarkable efficiency in large domain shifts and low-data scenarios. Our work shows the superiority of applying diffusion models to domain generalized and adaptive detection tasks and offers valuable insights for visual perception tasks across diverse domains. The code is available at \href{https://github.com/heboyong/Fitness-Generalization-Transferability}{Fitness-Generalization-Transferability}.
* Accepted by ICCV2025. arXiv admin note: text overlap with
arXiv:2503.02101
Via
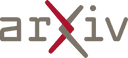
Jun 24, 2025
Abstract:Automated waterway environment perception is crucial for enabling unmanned surface vessels (USVs) to understand their surroundings and make informed decisions. Most existing waterway perception models primarily focus on instance-level object perception paradigms (e.g., detection, segmentation). However, due to the complexity of waterway environments, current perception datasets and models fail to achieve global semantic understanding of waterways, limiting large-scale monitoring and structured log generation. With the advancement of vision-language models (VLMs), we leverage image captioning to introduce WaterCaption, the first captioning dataset specifically designed for waterway environments. WaterCaption focuses on fine-grained, multi-region long-text descriptions, providing a new research direction for visual geo-understanding and spatial scene cognition. Exactly, it includes 20.2k image-text pair data with 1.8 million vocabulary size. Additionally, we propose Da Yu, an edge-deployable multi-modal large language model for USVs, where we propose a novel vision-to-language projector called Nano Transformer Adaptor (NTA). NTA effectively balances computational efficiency with the capacity for both global and fine-grained local modeling of visual features, thereby significantly enhancing the model's ability to generate long-form textual outputs. Da Yu achieves an optimal balance between performance and efficiency, surpassing state-of-the-art models on WaterCaption and several other captioning benchmarks.
* 14 pages, 13 figures
Via
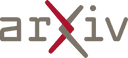
Jun 23, 2025
Abstract:Autonomous driving perception faces significant challenges due to occlusions and incomplete scene data in the environment. To overcome these issues, the task of semantic occupancy prediction (SOP) is proposed, which aims to jointly infer both the geometry and semantic labels of a scene from images. However, conventional camera-based methods typically treat all categories equally and primarily rely on local features, leading to suboptimal predictions, especially for dynamic foreground objects. To address this, we propose Object-Centric SOP (OC-SOP), a framework that integrates high-level object-centric cues extracted via a detection branch into the semantic occupancy prediction pipeline. This object-centric integration significantly enhances the prediction accuracy for foreground objects and achieves state-of-the-art performance among all categories on SemanticKITTI.
* under review
Via
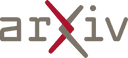
Jun 25, 2025
Abstract:Obtaining a better knowledge of the current state and behavior of objects orbiting Earth has proven to be essential for a range of applications such as active debris removal, in-orbit maintenance, or anomaly detection. 3D models represent a valuable source of information in the field of Space Situational Awareness (SSA). In this work, we leveraged Neural Radiance Fields (NeRF) to perform 3D reconstruction of non-cooperative space objects from simulated images. This scenario is challenging for NeRF models due to unusual camera characteristics and environmental conditions : mono-chromatic images, unknown object orientation, limited viewing angles, absence of diffuse lighting etc. In this work we focus primarly on the joint optimization of camera poses alongside the NeRF. Our experimental results show that the most accurate 3D reconstruction is achieved when training with successive images one-by-one. We estimate camera poses by optimizing an uniform rotation and use regularization to prevent successive poses from being too far apart.
* accepted for CVPR 2025 NFBCC workshop
Via
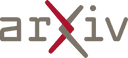
Jun 26, 2025
Abstract:Machine learning-assisted diagnosis is gaining traction in skin disease detection, but training effective models requires large amounts of high-quality data. Skin disease datasets often suffer from class imbalance, privacy concerns, and object bias, making data augmentation essential. While classical generative models are widely used, they demand extensive computational resources and lengthy training time. Quantum computing offers a promising alternative, but existing quantum-based image generation methods can only yield grayscale low-quality images. Through a novel classical-quantum latent space fusion technique, our work overcomes this limitation and introduces the first classical-quantum generative adversarial network (GAN) capable of generating color medical images. Our model outperforms classical deep convolutional GANs and existing hybrid classical-quantum GANs in both image generation quality and classification performance boost when used as data augmentation. Moreover, the performance boost is comparable with that achieved using state-of-the-art classical generative models, yet with over 25 times fewer parameters and 10 times fewer training epochs. Such results suggest a promising future for quantum image generation as quantum hardware advances. Finally, we demonstrate the robust performance of our model on real IBM quantum machine with hardware noise.
Via
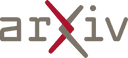
Jun 18, 2025
Abstract:Multispectral object detection, which integrates information from multiple bands, can enhance detection accuracy and environmental adaptability, holding great application potential across various fields. Although existing methods have made progress in cross-modal interaction, low-light conditions, and model lightweight, there are still challenges like the lack of a unified single-stage framework, difficulty in balancing performance and fusion strategy, and unreasonable modality weight allocation. To address these, based on the YOLOv11 framework, we present YOLOv11-RGBT, a new comprehensive multimodal object detection framework. We designed six multispectral fusion modes and successfully applied them to models from YOLOv3 to YOLOv12 and RT-DETR. After reevaluating the importance of the two modalities, we proposed a P3 mid-fusion strategy and multispectral controllable fine-tuning (MCF) strategy for multispectral models. These improvements optimize feature fusion, reduce redundancy and mismatches, and boost overall model performance. Experiments show our framework excels on three major open-source multispectral object detection datasets, like LLVIP and FLIR. Particularly, the multispectral controllable fine-tuning strategy significantly enhanced model adaptability and robustness. On the FLIR dataset, it consistently improved YOLOv11 models' mAP by 3.41%-5.65%, reaching a maximum of 47.61%, verifying the framework and strategies' effectiveness. The code is available at: https://github.com/wandahangFY/YOLOv11-RGBT.
* 29 pages, 8 figures . The errors in the first version have been
corrected, and no new version will be submitted in the near future. The next
version will include more experiments
Via
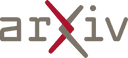
Jun 18, 2025
Abstract:Open-vocabulary 3D object detection has gained significant interest due to its critical applications in autonomous driving and embodied AI. Existing detection methods, whether offline or online, typically rely on dense point cloud reconstruction, which imposes substantial computational overhead and memory constraints, hindering real-time deployment in downstream tasks. To address this, we propose a novel reconstruction-free online framework tailored for memory-efficient and real-time 3D detection. Specifically, given streaming posed RGB-D video input, we leverage Cubify Anything as a pre-trained visual foundation model (VFM) for single-view 3D object detection by bounding boxes, coupled with CLIP to capture open-vocabulary semantics of detected objects. To fuse all detected bounding boxes across different views into a unified one, we employ an association module for correspondences of multi-views and an optimization module to fuse the 3D bounding boxes of the same instance predicted in multi-views. The association module utilizes 3D Non-Maximum Suppression (NMS) and a box correspondence matching module, while the optimization module uses an IoU-guided efficient random optimization technique based on particle filtering to enforce multi-view consistency of the 3D bounding boxes while minimizing computational complexity. Extensive experiments on ScanNetV2 and CA-1M datasets demonstrate that our method achieves state-of-the-art performance among online methods. Benefiting from this novel reconstruction-free paradigm for 3D object detection, our method exhibits great generalization abilities in various scenarios, enabling real-time perception even in environments exceeding 1000 square meters.
* 11 pages, 6 figures
Via
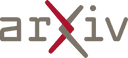