What is Object Detection? Object detection is a computer vision task in which the goal is to detect and locate objects of interest in an image or video. The task involves identifying the position and boundaries of objects in an image, and classifying the objects into different categories. It forms a crucial part of vision recognition, alongside image classification and retrieval.
Papers and Code
May 28, 2025
Abstract:Object counting has progressed from class-specific models, which count only known categories, to class-agnostic models that generalize to unseen categories. The next challenge is Referring Expression Counting (REC), where the goal is to count objects based on fine-grained attributes and contextual differences. Existing methods struggle with distinguishing visually similar objects that belong to the same category but correspond to different referring expressions. To address this, we propose C-REX, a novel contrastive learning framework, based on supervised contrastive learning, designed to enhance discriminative representation learning. Unlike prior works, C-REX operates entirely within the image space, avoiding the misalignment issues of image-text contrastive learning, thus providing a more stable contrastive signal. It also guarantees a significantly larger pool of negative samples, leading to improved robustness in the learned representations. Moreover, we showcase that our framework is versatile and generic enough to be applied to other similar tasks like class-agnostic counting. To support our approach, we analyze the key components of sota detection-based models and identify that detecting object centroids instead of bounding boxes is the key common factor behind their success in counting tasks. We use this insight to design a simple yet effective detection-based baseline to build upon. Our experiments show that C-REX achieves state-of-the-art results in REC, outperforming previous methods by more than 22\% in MAE and more than 10\% in RMSE, while also demonstrating strong performance in class-agnostic counting. Code is available at https://github.com/cvlab-stonybrook/c-rex.
* 9 pages, 4 figures
Via
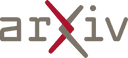
May 27, 2025
Abstract:Retrieving semantically similar but visually distinct contents has been a critical capability in visual search systems. In this work, we aim to tackle this problem with Visual Product Graph (VPG), leveraging high-performance infrastructure for storage and state-of-the-art computer vision models for image understanding. VPG is built to be an online real-time retrieval system that enables navigation from individual products to composite scenes containing those products, along with complementary recommendations. Our system not only offers contextual insights by showcasing how products can be styled in a context, but also provides recommendations for complementary products drawn from these inspirations. We discuss the essential components for building the Visual Product Graph, along with the core computer vision model improvements across object detection, foundational visual embeddings, and other visual signals. Our system achieves a 78.8% extremely similar@1 in end-to-end human relevance evaluations, and a 6% module engagement rate. The "Ways to Style It" module, powered by the Visual Product Graph technology, is deployed in production at Pinterest.
* 10 pages, 10 figures
Via
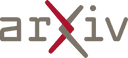
May 27, 2025
Abstract:Many state-of-the-art AI models deployed in cyber-physical systems (CPS), while highly accurate, are simply pattern-matchers.~With limited security guarantees, there are concerns for their reliability in safety-critical and contested domains. To advance assured AI, we advocate for a paradigm shift that imbues data-driven perception models with symbolic structure, inspired by a human's ability to reason over low-level features and high-level context. We propose a neuro-symbolic paradigm for perception (NeuSPaPer) and illustrate how joint object detection and scene graph generation (SGG) yields deep scene understanding.~Powered by foundation models for offline knowledge extraction and specialized SGG algorithms for real-time deployment, we design a framework leveraging structured relational graphs that ensures the integrity of situational awareness in autonomy. Using physics-based simulators and real-world datasets, we demonstrate how SGG bridges the gap between low-level sensor perception and high-level reasoning, establishing a foundation for resilient, context-aware AI and advancing trusted autonomy in CPS.
Via
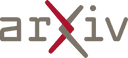
Jun 06, 2025
Abstract:Sensor fusion is crucial for a performant and robust Perception system in autonomous vehicles, but sensor staleness, where data from different sensors arrives with varying delays, poses significant challenges. Temporal misalignment between sensor modalities leads to inconsistent object state estimates, severely degrading the quality of trajectory predictions that are critical for safety. We present a novel and model-agnostic approach to address this problem via (1) a per-point timestamp offset feature (for LiDAR and radar both relative to camera) that enables fine-grained temporal awareness in sensor fusion, and (2) a data augmentation strategy that simulates realistic sensor staleness patterns observed in deployed vehicles. Our method is integrated into a perspective-view detection model that consumes sensor data from multiple LiDARs, radars and cameras. We demonstrate that while a conventional model shows significant regressions when one sensor modality is stale, our approach reaches consistently good performance across both synchronized and stale conditions.
* This paper has been accepted by CVPR 2025 Precognition Workshop
Via
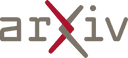
May 27, 2025
Abstract:From uncertainty quantification to real-world object detection, we recognize the importance of machine learning algorithms, particularly in safety-critical domains such as autonomous driving or medical diagnostics. In machine learning, ambiguous data plays an important role in various machine learning domains. Optical illusions present a compelling area of study in this context, as they offer insight into the limitations of both human and machine perception. Despite this relevance, optical illusion datasets remain scarce. In this work, we introduce a novel dataset of optical illusions featuring intermingled animal pairs designed to evoke perceptual ambiguity. We identify generalizable visual concepts, particularly gaze direction and eye cues, as subtle yet impactful features that significantly influence model accuracy. By confronting models with perceptual ambiguity, our findings underscore the importance of concepts in visual learning and provide a foundation for studying bias and alignment between human and machine vision. To make this dataset useful for general purposes, we generate optical illusions systematically with different concepts discussed in our bias mitigation section. The dataset is accessible in Kaggle via https://kaggle.com/datasets/693bf7c6dd2cb45c8a863f9177350c8f9849a9508e9d50526e2ffcc5559a8333. Our source code can be found at https://github.com/KDD-OpenSource/Ambivision.git.
* 19 pages, 18 figures
Via
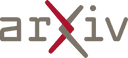
May 27, 2025
Abstract:Object concepts play a foundational role in human visual cognition, enabling perception, memory, and interaction in the physical world. Inspired by findings in developmental neuroscience - where infants are shown to acquire object understanding through observation of motion - we propose a biologically inspired framework for learning object-centric visual representations in an unsupervised manner. Our key insight is that motion boundary serves as a strong signal for object-level grouping, which can be used to derive pseudo instance supervision from raw videos. Concretely, we generate motion-based instance masks using off-the-shelf optical flow and clustering algorithms, and use them to train visual encoders via contrastive learning. Our framework is fully label-free and does not rely on camera calibration, making it scalable to large-scale unstructured video data. We evaluate our approach on three downstream tasks spanning both low-level (monocular depth estimation) and high-level (3D object detection and occupancy prediction) vision. Our models outperform previous supervised and self-supervised baselines and demonstrate strong generalization to unseen scenes. These results suggest that motion-induced object representations offer a compelling alternative to existing vision foundation models, capturing a crucial but overlooked level of abstraction: the visual instance. The corresponding code will be released upon paper acceptance.
Via
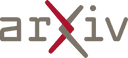
May 27, 2025
Abstract:Fire detection in dynamic environments faces continuous challenges, including the interference of illumination changes, many false detections or missed detections, and it is difficult to achieve both efficiency and accuracy. To address the problem of feature extraction limitation and information loss in the existing YOLO-based models, this study propose You Only Look Once for Fire Detection with Attention-guided Inverted Residual and Dual-pooling Downscale Fusion (YOLO-FireAD) with two core innovations: (1) Attention-guided Inverted Residual Block (AIR) integrates hybrid channel-spatial attention with inverted residuals to adaptively enhance fire features and suppress environmental noise; (2) Dual Pool Downscale Fusion Block (DPDF) preserves multi-scale fire patterns through learnable fusion of max-average pooling outputs, mitigating small-fire detection failures. Extensive evaluation on two public datasets shows the efficient performance of our model. Our proposed model keeps the sum amount of parameters (1.45M, 51.8% lower than YOLOv8n) (4.6G, 43.2% lower than YOLOv8n), and mAP75 is higher than the mainstream real-time object detection models YOLOv8n, YOL-Ov9t, YOLOv10n, YOLO11n, YOLOv12n and other YOLOv8 variants 1.3-5.5%.
Via
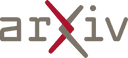
Jun 05, 2025
Abstract:Small, fast, and lightweight drones present significant challenges for traditional RGB cameras due to their limitations in capturing fast-moving objects, especially under challenging lighting conditions. Event cameras offer an ideal solution, providing high temporal definition and dynamic range, yet existing benchmarks often lack fine temporal resolution or drone-specific motion patterns, hindering progress in these areas. This paper introduces the Florence RGB-Event Drone dataset (FRED), a novel multimodal dataset specifically designed for drone detection, tracking, and trajectory forecasting, combining RGB video and event streams. FRED features more than 7 hours of densely annotated drone trajectories, using 5 different drone models and including challenging scenarios such as rain and adverse lighting conditions. We provide detailed evaluation protocols and standard metrics for each task, facilitating reproducible benchmarking. The authors hope FRED will advance research in high-speed drone perception and multimodal spatiotemporal understanding.
Via
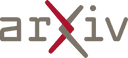
Jun 04, 2025
Abstract:Finding reliable matches is essential in multi-object tracking to ensure the accuracy and reliability of perception systems in safety-critical applications such as autonomous vehicles. Effective matching mitigates perception errors, enhancing object identification and tracking for improved performance and safety. However, traditional metrics such as Intersection over Union (IoU) and Center Point Distances (CPDs), which are effective in 2D image planes, often fail to find critical matches in complex 3D scenes. To address this limitation, we introduce Contour Errors (CEs), an ego or object-centric metric for identifying matches of interest in tracking scenarios from a functional perspective. By comparing bounding boxes in the ego vehicle's frame, contour errors provide a more functionally relevant assessment of object matches. Extensive experiments on the nuScenes dataset demonstrate that contour errors improve the reliability of matches over the state-of-the-art 2D IoU and CPD metrics in tracking-by-detection methods. In 3D car tracking, our results show that Contour Errors reduce functional failures (FPs/FNs) by 80% at close ranges and 60% at far ranges compared to IoU in the evaluation stage.
Via
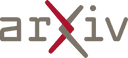
Jun 04, 2025
Abstract:Public health experts need scalable approaches to monitor large volumes of health data (e.g., cases, hospitalizations, deaths) for outbreaks or data quality issues. Traditional alert-based monitoring systems struggle with modern public health data monitoring systems for several reasons, including that alerting thresholds need to be constantly reset and the data volumes may cause application lag. Instead, we propose a ranking-based monitoring paradigm that leverages new AI anomaly detection methods. Through a multi-year interdisciplinary collaboration, the resulting system has been deployed at a national organization to monitor up to 5,000,000 data points daily. A three-month longitudinal deployed evaluation revealed a significant improvement in monitoring objectives, with a 54x increase in reviewer speed efficiency compared to traditional alert-based methods. This work highlights the potential of human-centered AI to transform public health decision-making.
Via
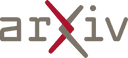