Topic:Image To Image Translation
What is Image To Image Translation? Image-to-image translation is the process of converting an image from one domain to another using deep learning techniques.
Papers and Code
May 03, 2025
Abstract:Collecting large-scale crop disease images in the field is labor-intensive and time-consuming. Generative models (GMs) offer an alternative by creating synthetic samples that resemble real-world images. However, existing research primarily relies on Generative Adversarial Networks (GANs)-based image-to-image translation and lack a comprehensive analysis of computational requirements in agriculture. Therefore, this research explores a multi-modal text-to-image approach for generating synthetic crop disease images and is the first to provide computational benchmarking in this context. We trained three Stable Diffusion (SD) variants-SDXL, SD3.5M (medium), and SD3.5L (large)-and fine-tuned them using Dreambooth and Low-Rank Adaptation (LoRA) fine-tuning techniques to enhance generalization. SD3.5M outperformed the others, with an average memory usage of 18 GB, power consumption of 180 W, and total energy use of 1.02 kWh/500 images (0.002 kWh per image) during inference task. Our results demonstrate SD3.5M's ability to generate 500 synthetic images from just 36 in-field samples in 1.5 hours. We recommend SD3.5M for efficient crop disease data generation.
Via
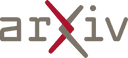
May 15, 2025
Abstract:Despite significant advances in modeling image priors via diffusion models, 3D-aware image editing remains challenging, in part because the object is only specified via a single image. To tackle this challenge, we propose 3D-Fixup, a new framework for editing 2D images guided by learned 3D priors. The framework supports difficult editing situations such as object translation and 3D rotation. To achieve this, we leverage a training-based approach that harnesses the generative power of diffusion models. As video data naturally encodes real-world physical dynamics, we turn to video data for generating training data pairs, i.e., a source and a target frame. Rather than relying solely on a single trained model to infer transformations between source and target frames, we incorporate 3D guidance from an Image-to-3D model, which bridges this challenging task by explicitly projecting 2D information into 3D space. We design a data generation pipeline to ensure high-quality 3D guidance throughout training. Results show that by integrating these 3D priors, 3D-Fixup effectively supports complex, identity coherent 3D-aware edits, achieving high-quality results and advancing the application of diffusion models in realistic image manipulation. The code is provided at https://3dfixup.github.io/
* SIGGRAPH 2025. Project page: https://3dfixup.github.io/
Via
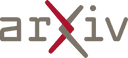
May 20, 2025
Abstract:Artificial intelligence (AI) holds strong potential for medical diagnostics, yet its clinical adoption is limited by a lack of interpretability and generalizability. This study introduces the Pathobiological Dictionary for Liver Cancer (LCP1.0), a practical framework designed to translate complex Pathomics and Radiomics Features (PF and RF) into clinically meaningful insights aligned with existing diagnostic workflows. QuPath and PyRadiomics, standardized according to IBSI guidelines, were used to extract 333 imaging features from hepatocellular carcinoma (HCC) tissue samples, including 240 PF-based-cell detection/intensity, 74 RF-based texture, and 19 RF-based first-order features. Expert-defined ROIs from the public dataset excluded artifact-prone areas, and features were aggregated at the case level. Their relevance to the WHO grading system was assessed using multiple classifiers linked with feature selectors. The resulting dictionary was validated by 8 experts in oncology and pathology. In collaboration with 10 domain experts, we developed a Pathobiological dictionary of imaging features such as PFs and RF. In our study, the Variable Threshold feature selection algorithm combined with the SVM model achieved the highest accuracy (0.80, P-value less than 0.05), selecting 20 key features, primarily clinical and pathomics traits such as Centroid, Cell Nucleus, and Cytoplasmic characteristics. These features, particularly nuclear and cytoplasmic, were strongly associated with tumor grading and prognosis, reflecting atypia indicators like pleomorphism, hyperchromasia, and cellular orientation.The LCP1.0 provides a clinically validated bridge between AI outputs and expert interpretation, enhancing model transparency and usability. Aligning AI-derived features with clinical semantics supports the development of interpretable, trustworthy diagnostic tools for liver cancer pathology.
* 29 pages, 4 figures and 1 table
Via
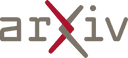
May 22, 2025
Abstract:The effective communication of procedural knowledge remains a significant challenge in natural language processing (NLP), as purely textual instructions often fail to convey complex physical actions and spatial relationships. We address this limitation by proposing a language-driven framework that translates procedural text into coherent visual instructions. Our approach models the linguistic structure of instructional content by decomposing it into goal statements and sequential steps, then conditioning visual generation on these linguistic elements. We introduce three key innovations: (1) a constituency parser-based text encoding mechanism that preserves semantic completeness even with lengthy instructions, (2) a pairwise discourse coherence model that maintains consistency across instruction sequences, and (3) a novel evaluation protocol specifically designed for procedural language-to-image alignment. Our experiments across three instructional datasets (HTStep, CaptainCook4D, and WikiAll) demonstrate that our method significantly outperforms existing baselines in generating visuals that accurately reflect the linguistic content and sequential nature of instructions. This work contributes to the growing body of research on grounding procedural language in visual content, with applications spanning education, task guidance, and multimodal language understanding.
* 13 pages, 5 figures, under review
Via
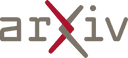
May 22, 2025
Abstract:Recent optical flow estimation methods often employ local cost sampling from a dense all-pairs correlation volume. This results in quadratic computational and memory complexity in the number of pixels. Although an alternative memory-efficient implementation with on-demand cost computation exists, this is slower in practice and therefore prior methods typically process images at reduced resolutions, missing fine-grained details. To address this, we propose a more efficient implementation of the all-pairs correlation volume sampling, still matching the exact mathematical operator as defined by RAFT. Our approach outperforms on-demand sampling by up to 90% while maintaining low memory usage, and performs on par with the default implementation with up to 95% lower memory usage. As cost sampling makes up a significant portion of the overall runtime, this can translate to up to 50% savings for the total end-to-end model inference in memory-constrained environments. Our evaluation of existing methods includes an 8K ultra-high-resolution dataset and an additional inference-time modification of the recent SEA-RAFT method. With this, we achieve state-of-the-art results at high resolutions both in accuracy and efficiency.
Via
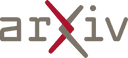
May 23, 2025
Abstract:This paper presents a novel method for monocular patient-to-image intraoperative registration, specifically designed to operate without any external hardware tracking equipment or fiducial point markers. Leveraging a synthetic microscopy surgical scene dataset with a wide range of transformations, our approach directly maps preoperative CT scans to 2D intraoperative surgical frames through a lightweight neural network for real-time cochlear implant surgery guidance via a zero-shot learning approach. Unlike traditional methods, our framework seamlessly integrates with monocular surgical microscopes, making it highly practical for clinical use without additional hardware dependencies and requirements. Our method estimates camera poses, which include a rotation matrix and a translation vector, by learning from the synthetic dataset, enabling accurate and efficient intraoperative registration. The proposed framework was evaluated on nine clinical cases using a patient-specific and cross-patient validation strategy. Our results suggest that our approach achieves clinically relevant accuracy in predicting 6D camera poses for registering 3D preoperative CT scans to 2D surgical scenes with an angular error within 10 degrees in most cases, while also addressing limitations of traditional methods, such as reliance on external tracking systems or fiducial markers.
Via
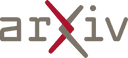
Apr 24, 2025
Abstract:This paper presents the technical solution proposed by Huawei Translation Service Center (HW-TSC) for the "End-to-End Document Image Machine Translation for Complex Layouts" competition at the 19th International Conference on Document Analysis and Recognition (DIMT25@ICDAR2025). Leveraging state-of-the-art open-source large vision-language model (LVLM), we introduce a training framework that combines multi-task learning with perceptual chain-of-thought to develop a comprehensive end-to-end document translation system. During the inference phase, we apply minimum Bayesian decoding and post-processing strategies to further enhance the system's translation capabilities. Our solution uniquely addresses both OCR-based and OCR-free document image translation tasks within a unified framework. This paper systematically details the training methods, inference strategies, LVLM base models, training data, experimental setups, and results, demonstrating an effective approach to document image machine translation.
* 7 pages, 1 figures, 2 tables
Via
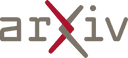
May 08, 2025
Abstract:Multimodal learning is an emerging research topic across multiple disciplines but has rarely been applied to planetary science. In this contribution, we identify that reflectance parameter estimation and image-based 3D reconstruction of lunar images can be formulated as a multimodal learning problem. We propose a single, unified transformer architecture trained to learn shared representations between multiple sources like grayscale images, digital elevation models, surface normals, and albedo maps. The architecture supports flexible translation from any input modality to any target modality. Predicting DEMs and albedo maps from grayscale images simultaneously solves the task of 3D reconstruction of planetary surfaces and disentangles photometric parameters and height information. Our results demonstrate that our foundation model learns physically plausible relations across these four modalities. Adding more input modalities in the future will enable tasks such as photometric normalization and co-registration.
* 14pages
Via
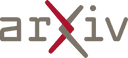
Apr 21, 2025
Abstract:Diffusion tensor imaging (DTI) provides crucial insights into the microstructure of the human brain, but it can be time-consuming to acquire compared to more readily available T1-weighted (T1w) magnetic resonance imaging (MRI). To address this challenge, we propose a diffusion bridge model for 3D brain image translation between T1w MRI and DTI modalities. Our model learns to generate high-quality DTI fractional anisotropy (FA) images from T1w images and vice versa, enabling cross-modality data augmentation and reducing the need for extensive DTI acquisition. We evaluate our approach using perceptual similarity, pixel-level agreement, and distributional consistency metrics, demonstrating strong performance in capturing anatomical structures and preserving information on white matter integrity. The practical utility of the synthetic data is validated through sex classification and Alzheimer's disease classification tasks, where the generated images achieve comparable performance to real data. Our diffusion bridge model offers a promising solution for improving neuroimaging datasets and supporting clinical decision-making, with the potential to significantly impact neuroimaging research and clinical practice.
Via
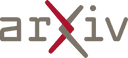
May 06, 2025
Abstract:Accurate MRI-to-CT translation promises the integration of complementary imaging information without the need for additional imaging sessions. Given the practical challenges associated with acquiring paired MRI and CT scans, the development of robust methods capable of leveraging unpaired datasets is essential for advancing the MRI-to-CT translation. Current unpaired MRI-to-CT translation methods, which predominantly rely on cycle consistency and contrastive learning frameworks, frequently encounter challenges in accurately translating anatomical features that are highly discernible on CT but less distinguishable on MRI, such as bone structures. This limitation renders these approaches less suitable for applications in radiation therapy, where precise bone representation is essential for accurate treatment planning. To address this challenge, we propose a path- and bone-contour regularized approach for unpaired MRI-to-CT translation. In our method, MRI and CT images are projected to a shared latent space, where the MRI-to-CT mapping is modeled as a continuous flow governed by neural ordinary differential equations. The optimal mapping is obtained by minimizing the transition path length of the flow. To enhance the accuracy of translated bone structures, we introduce a trainable neural network to generate bone contours from MRI and implement mechanisms to directly and indirectly encourage the model to focus on bone contours and their adjacent regions. Evaluations conducted on three datasets demonstrate that our method outperforms existing unpaired MRI-to-CT translation approaches, achieving lower overall error rates. Moreover, in a downstream bone segmentation task, our approach exhibits superior performance in preserving the fidelity of bone structures. Our code is available at: https://github.com/kennysyp/PaBoT.
Via
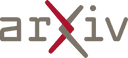