Topic:Unsupervised Anomaly Detection
What is Unsupervised Anomaly Detection? Unsupervised anomaly detection is the process of identifying unusual patterns or outliers in data without using labeled examples.
Papers and Code
Apr 22, 2025
Abstract:We describe the use of an unsupervised Random Forest for similarity learning and improved unsupervised anomaly detection. By training a Random Forest to discriminate between real data and synthetic data sampled from a uniform distribution over the real data bounds, a distance measure is obtained that anisometrically transforms the data, expanding distances at the boundary of the data manifold. We show that using distances recovered from this transformation improves the accuracy of unsupervised anomaly detection, compared to other commonly used detectors, demonstrated over a large number of benchmark datasets. As well as improved performance, this method has advantages over other unsupervised anomaly detection methods, including minimal requirements for data preprocessing, native handling of missing data, and potential for visualizations. By relating outlier scores to partitions of the Random Forest, we develop a method for locally explainable anomaly predictions in terms of feature importance.
* 14 pages, 5 figures
Via
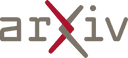
Apr 21, 2025
Abstract:Recent advances in unsupervised anomaly detection (UAD) have shifted from single-class to multi-class scenarios. In such complex contexts, the increasing pattern diversity has brought two challenges to reconstruction-based approaches: (1) over-generalization: anomalies that are subtle or share compositional similarities with normal patterns may be reconstructed with high fidelity, making them difficult to distinguish from normal instances; and (2) insufficient normality reconstruction: complex normal features, such as intricate textures or fine-grained structures, may not be faithfully reconstructed due to the model's limited representational capacity, resulting in false positives. Existing methods typically focus on addressing the former, which unintentionally exacerbate the latter, resulting in inadequate representation of intricate normal patterns. To concurrently address these two challenges, we propose a Memory-augmented Dual-Decoder Networks (MDD-Net). This network includes two critical components: a Dual-Decoder Reverse Distillation Network (DRD-Net) and a Class-aware Memory Module (CMM). Specifically, the DRD-Net incorporates a restoration decoder designed to recover normal features from synthetic abnormal inputs and an identity decoder to reconstruct features that maintain the anomalous semantics. By exploiting the discrepancy between features produced by two decoders, our approach refines anomaly scores beyond the conventional encoder-decoder comparison paradigm, effectively reducing false positives and enhancing localization accuracy. Furthermore, the CMM explicitly encodes and preserves class-specific normal prototypes, actively steering the network away from anomaly reconstruction. Comprehensive experimental results across several benchmarks demonstrate the superior performance of our MDD-Net framework over current SoTA approaches in multi-class UAD tasks.
Via
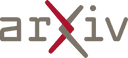
Apr 23, 2025
Abstract:The rapid growth of unlabeled time-series data in domains such as wireless communications, radar, biomedical engineering, and the Internet of Things (IoT) has driven advancements in unsupervised learning. This review synthesizes recent progress in applying autoencoders and vision transformers for unsupervised signal analysis, focusing on their architectures, applications, and emerging trends. We explore how these models enable feature extraction, anomaly detection, and classification across diverse signal types, including electrocardiograms, radar waveforms, and IoT sensor data. The review highlights the strengths of hybrid architectures and self-supervised learning, while identifying challenges in interpretability, scalability, and domain generalization. By bridging methodological innovations and practical applications, this work offers a roadmap for developing robust, adaptive models for signal intelligence.
Via
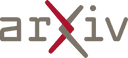
Apr 21, 2025
Abstract:With the widespread availability of sensor data across industrial and operational systems, we frequently encounter heterogeneous time series from multiple systems. Anomaly detection is crucial for such systems to facilitate predictive maintenance. However, most existing anomaly detection methods are designed for either univariate or single-system multivariate data, making them insufficient for these complex scenarios. To address this, we introduce M$^2$AD, a framework for unsupervised anomaly detection in multivariate time series data from multiple systems. M$^2$AD employs deep models to capture expected behavior under normal conditions, using the residuals as indicators of potential anomalies. These residuals are then aggregated into a global anomaly score through a Gaussian Mixture Model and Gamma calibration. We theoretically demonstrate that this framework can effectively address heterogeneity and dependencies across sensors and systems. Empirically, M$^2$AD outperforms existing methods in extensive evaluations by 21% on average, and its effectiveness is demonstrated on a large-scale real-world case study on 130 assets in Amazon Fulfillment Centers. Our code and results are available at https://github.com/sarahmish/M2AD.
* Accepted at AISTATS 2025
Via
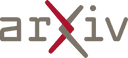
Apr 17, 2025
Abstract:Detecting mission-critical anomalous events and data is a crucial challenge across various industries, including finance, healthcare, and energy. Quantum computing has recently emerged as a powerful tool for tackling several machine learning tasks, but training quantum machine learning models remains challenging, particularly due to the difficulty of gradient calculation. The challenge is even greater for anomaly detection, where unsupervised learning methods are essential to ensure practical applicability. To address these issues, we propose Quorum, the first quantum anomaly detection framework designed for unsupervised learning that operates without requiring any training.
Via
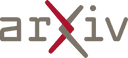
Apr 17, 2025
Abstract:Multi-class Unsupervised Anomaly Detection algorithms (MUAD) are receiving increasing attention due to their relatively low deployment costs and improved training efficiency. However, the real-world effectiveness of MUAD methods is questioned due to limitations in current Industrial Anomaly Detection (IAD) datasets. These datasets contain numerous classes that are unlikely to be produced by the same factory and fail to cover multiple structures or appearances. Additionally, the defects do not reflect real-world characteristics. Therefore, we introduce the Heterogeneous Same-Sort Industrial Anomaly Detection (HSS-IAD) dataset, which contains 8,580 images of metallic-like industrial parts and precise anomaly annotations. These parts exhibit variations in structure and appearance, with subtle defects that closely resemble the base materials. We also provide foreground images for synthetic anomaly generation. Finally, we evaluate popular IAD methods on this dataset under multi-class and class-separated settings, demonstrating its potential to bridge the gap between existing datasets and real factory conditions. The dataset is available at https://github.com/Qiqigeww/HSS-IAD-Dataset.
* Accepted to IEEE ICME 2025
Via
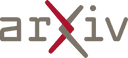
Apr 16, 2025
Abstract:Unsupervised anomaly detection in hyperspectral images (HSI), aiming to detect unknown targets from backgrounds, is challenging for earth surface monitoring. However, current studies are hindered by steep computational costs due to the high-dimensional property of HSI and dense sampling-based training paradigm, constraining their rapid deployment. Our key observation is that, during training, not all samples within the same homogeneous area are indispensable, whereas ingenious sampling can provide a powerful substitute for reducing costs. Motivated by this, we propose an Asymmetrical Consensus State Space Model (ACMamba) to significantly reduce computational costs without compromising accuracy. Specifically, we design an asymmetrical anomaly detection paradigm that utilizes region-level instances as an efficient alternative to dense pixel-level samples. In this paradigm, a low-cost Mamba-based module is introduced to discover global contextual attributes of regions that are essential for HSI reconstruction. Additionally, we develop a consensus learning strategy from the optimization perspective to simultaneously facilitate background reconstruction and anomaly compression, further alleviating the negative impact of anomaly reconstruction. Theoretical analysis and extensive experiments across eight benchmarks verify the superiority of ACMamba, demonstrating a faster speed and stronger performance over the state-of-the-art.
* 15 pages, 9 figures
Via
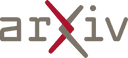
Apr 17, 2025
Abstract:We propose a new unsupervised anomaly detection method based on the sliced-Wasserstein distance for training data selection in machine learning approaches. Our filtering technique is interesting for decision-making pipelines deploying machine learning models in critical sectors, e.g., power systems, as it offers a conservative data selection and an optimal transport interpretation. To ensure the scalability of our method, we provide two efficient approximations. The first approximation processes reduced-cardinality representations of the datasets concurrently. The second makes use of a computationally light Euclidian distance approximation. Additionally, we open the first dataset showcasing localized critical peak rebate demand response in a northern climate. We present the filtering patterns of our method on synthetic datasets and numerically benchmark our method for training data selection. Finally, we employ our method as part of a first forecasting benchmark for our open-source dataset.
* arXiv admin note: substantial text overlap with arXiv:2410.21712
Via
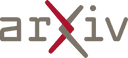
Apr 19, 2025
Abstract:Time series anomaly detection holds notable importance for risk identification and fault detection across diverse application domains. Unsupervised learning methods have become popular because they have no requirement for labels. However, due to the challenges posed by the multiplicity of abnormal patterns, the sparsity of anomalies, and the growth of data scale and complexity, these methods often fail to capture robust and representative dependencies within the time series for identifying anomalies. To enhance the ability of models to capture normal patterns of time series and avoid the retrogression of modeling ability triggered by the dependencies on high-quality prior knowledge, we propose a differencing-based contrastive representation learning framework for time series anomaly detection (DConAD). Specifically, DConAD generates differential data to provide additional information about time series and utilizes transformer-based architecture to capture spatiotemporal dependencies, which enhances the robustness of unbiased representation learning ability. Furthermore, DConAD implements a novel KL divergence-based contrastive learning paradigm that only uses positive samples to avoid deviation from reconstruction and deploys the stop-gradient strategy to compel convergence. Extensive experiments on five public datasets show the superiority and effectiveness of DConAD compared with nine baselines. The code is available at https://github.com/shaieesss/DConAD.
Via
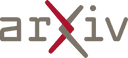
Apr 15, 2025
Abstract:We present a refined version of the Anomaly Awareness framework for enhancing unsupervised anomaly detection. Our approach introduces minimal supervision into Variational Autoencoders (VAEs) through a two-stage training strategy: the model is first trained in an unsupervised manner on background data, and then fine-tuned using a small sample of labeled anomalies to encourage larger reconstruction errors for anomalous samples. We validate the method across diverse domains, including the MNIST dataset with synthetic anomalies, network intrusion data from the CICIDS benchmark, collider physics data from the LHCO2020 dataset, and simulated events from the Standard Model Effective Field Theory (SMEFT). The latter provides a realistic example of subtle kinematic deviations in Higgs boson production. In all cases, the model demonstrates improved sensitivity to unseen anomalies, achieving better separation between normal and anomalous samples. These results indicate that even limited anomaly information, when incorporated through targeted fine-tuning, can substantially improve the generalization and performance of unsupervised models for anomaly detection.
* 16 pages, 5 figures
Via
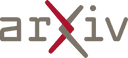