Apr 24, 2025
Abstract:Locomotion plays a crucial role in shaping the user experience within virtual reality environments. In particular, hands-free locomotion offers a valuable alternative by supporting accessibility and freeing users from reliance on handheld controllers. To this end, traditional speech-based methods often depend on rigid command sets, limiting the naturalness and flexibility of interaction. In this study, we propose a novel locomotion technique powered by large language models (LLMs), which allows users to navigate virtual environments using natural language with contextual awareness. We evaluate three locomotion methods: controller-based teleportation, voice-based steering, and our language model-driven approach. Our evaluation measures include eye-tracking data analysis, including explainable machine learning through SHAP analysis as well as standardized questionnaires for usability, presence, cybersickness, and cognitive load to examine user attention and engagement. Our findings indicate that the LLM-driven locomotion possesses comparable usability, presence, and cybersickness scores to established methods like teleportation, demonstrating its novel potential as a comfortable, natural language-based, hands-free alternative. In addition, it enhances user attention within the virtual environment, suggesting greater engagement. Complementary to these findings, SHAP analysis revealed that fixation, saccade, and pupil-related features vary across techniques, indicating distinct patterns of visual attention and cognitive processing. Overall, we state that our method can facilitate hands-free locomotion in virtual spaces, especially in supporting accessibility.
* This work has been submitted to the IEEE for possible publication
Via
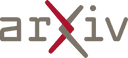