Topic:Metal Artifact Reduction
What is Metal Artifact Reduction? Metal-artifact reduction is the process of reducing image artifacts caused by metal objects in medical images.
Papers and Code
Apr 28, 2025
Abstract:Low-dose CT (LDCT) is capable of reducing X-ray radiation exposure, but it will potentially degrade image quality, even yields metal artifacts at the case of metallic implants. For simultaneous LDCT reconstruction and metal artifact reduction (LDMAR), existing deep learning-based efforts face two main limitations: i) the network design neglects multi-scale and within-scale information; ii) training a distinct model for each dose necessitates significant storage space for multiple doses. To fill these gaps, we propose a prompt guiding multi-scale adaptive sparse representation-driven network, abbreviated as PMSRNet, for LDMAR task. Specifically, we construct PMSRNet inspired from multi-scale sparsifying frames, and it can simultaneously employ within-scale characteristics and cross-scale complementarity owing to an elaborated prompt guiding scale-adaptive threshold generator (PSATG) and a built multi-scale coefficient fusion module (MSFuM). The PSATG can adaptively capture multiple contextual information to generate more faithful thresholds, achieved by fusing features from local, regional, and global levels. Furthermore, we elaborate a model interpretable dual domain LDMAR framework called PDuMSRNet, and train single model with a prompt guiding strategy for multiple dose levels. We build a prompt guiding module, whose input contains dose level, metal mask and input instance, to provide various guiding information, allowing a single model to accommodate various CT dose settings. Extensive experiments at various dose levels demonstrate that the proposed methods outperform the state-of-the-art LDMAR methods.
Via
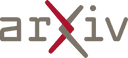
Jan 26, 2025
Abstract:Metal artifacts in computed tomography (CT) images can significantly degrade image quality and impede accurate diagnosis. Supervised metal artifact reduction (MAR) methods, trained using simulated datasets, often struggle to perform well on real clinical CT images due to a substantial domain gap. Although state-of-the-art semi-supervised methods use pseudo ground-truths generated by a prior network to mitigate this issue, their reliance on a fixed prior limits both the quality and quantity of these pseudo ground-truths, introducing confirmation bias and reducing clinical applicability. To address these limitations, we propose a novel Radiologist-In-the-loop SElf-training framework for MAR, termed RISE-MAR, which can integrate radiologists' feedback into the semi-supervised learning process, progressively improving the quality and quantity of pseudo ground-truths for enhanced generalization on real clinical CT images. For quality assurance, we introduce a clinical quality assessor model that emulates radiologist evaluations, effectively selecting high-quality pseudo ground-truths for semi-supervised training. For quantity assurance, our self-training framework iteratively generates additional high-quality pseudo ground-truths, expanding the clinical dataset and further improving model generalization. Extensive experimental results on multiple clinical datasets demonstrate the superior generalization performance of our RISE-MAR over state-of-the-art methods, advancing the development of MAR models for practical application. Code is available at https://github.com/Masaaki-75/rise-mar.
* IEEE TMI 2025
Via
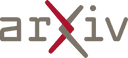
Jan 10, 2025
Abstract:Purpose: To propose a B1+ mapping technique for imaging of body parts containing metal hardware, based on magnitude images acquired with turbo spin echo (TSE) pulse sequences. Theory and Methods: To encode the underlying B1+, multiple (two to four) TSE image sets with various excitation and refocusing flip angles were acquired. To this end, the acquired signal intensities were matched to a database of simulated signals which was generated by solving the Bloch equations taking into account the exact sequence parameters. The retrieved B1+ values were validated against gradient-recalled and spin echo dual angle methods, as well as a vendor-provided turboFLASH-based mapping sequence, in gel phantoms and human subjects without and with metal implants. Results: In the absence of metal, phantom experiments demonstrated excellent agreement between the proposed technique using three or four flip angle sets and reference dual angle methods. In human subjects without metal implants, the proposed technique with three or four flip angle sets showed excellent correlation with the spin echo dual angle method. In the presence of metal, both phantoms and human subjects revealed a narrow range of B1+ estimation with the reference techniques, whereas the proposed technique successfully resolved B1+ near the metal. In select cases, the technique was implemented in conjunction with multispectral metal artifact reduction sequences and successfully applied for B1+ shimming. Conclusion: The proposed technique enables resolution of B1+ values in regions near metal hardware, overcoming susceptibility-related and narrow-range limitations of standard mapping techniques.
Via
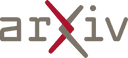
Oct 26, 2024
Abstract:Photon-counting computed tomography (PCCT) marks a significant advancement over conventional energy-integrating detector (EID) CT systems. This review highlights PCCT's superior spatial and contrast resolution, reduced radiation dose, and multi-energy imaging capabilities, which address key challenges in radiotherapy, such as accurate tumor delineation, precise dose calculation, and treatment response monitoring. PCCT's improved anatomical clarity enhances tumor targeting while minimizing damage to surrounding healthy tissues. Additionally, metal artifact reduction (MAR) and quantitative imaging capabilities optimize workflows, enabling adaptive radiotherapy and radiomics-driven personalized treatment. Emerging clinical applications in brachytherapy and radiopharmaceutical therapy (RPT) show promising outcomes, although challenges like high costs and limited software integration remain. With advancements in artificial intelligence (AI) and dedicated radiotherapy packages, PCCT is poised to transform precision, safety, and efficacy in cancer radiotherapy, marking it as a pivotal technology for future clinical practice.
Via
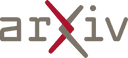
Jun 18, 2024
Abstract:In computed tomography (CT), the presence of metallic implants in patients often leads to disruptive artifacts in the reconstructed images, hindering accurate diagnosis. Recently, a large amount of supervised deep learning-based approaches have been proposed for metal artifact reduction (MAR). However, these methods neglect the influence of initial training weights. In this paper, we have discovered that the uncertainty image computed from the restoration result of initial training weights can effectively highlight high-frequency regions, including metal artifacts. This observation can be leveraged to assist the MAR network in removing metal artifacts. Therefore, we propose an uncertainty constraint (UC) loss that utilizes the uncertainty image as an adaptive weight to guide the MAR network to focus on the metal artifact region, leading to improved restoration. The proposed UC loss is designed to be a plug-and-play method, compatible with any MAR framework, and easily adoptable. To validate the effectiveness of the UC loss, we conduct extensive experiments on the public available Deeplesion and CLINIC-metal dataset. Experimental results demonstrate that the UC loss further optimizes the network training process and significantly improves the removal of metal artifacts.
Via
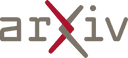
Jun 25, 2024
Abstract:X-ray computed tomography (CT) reconstructs the internal morphology of a three dimensional object from a collection of projection images, most commonly using a single rotation axis. However, for objects containing dense materials like metal, the use of a single rotation axis may leave some regions of the object obscured by the metal, even though projections from other rotation axes (or poses) might contain complementary information that would better resolve these obscured regions. In this paper, we propose pixel-weighted Multi-pose Fusion to reduce metal artifacts by fusing the information from complementary measurement poses into a single reconstruction. Our method uses Multi-Agent Consensus Equilibrium (MACE), an extension of Plug-and-Play, as a framework for integrating projection data from different poses. A primary novelty of the proposed method is that the output of different MACE agents are fused in a pixel-weighted manner to minimize the effects of metal throughout the reconstruction. Using real CT data on an object with and without metal inserts, we demonstrate that the proposed pixel-weighted Multi-pose Fusion method significantly reduces metal artifacts relative to single-pose reconstructions.
* Submitted to IEEE MMSP 2024. arXiv admin note: substantial text
overlap with arXiv:2209.07561
Via
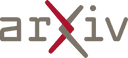
May 11, 2024
Abstract:Emerging unsupervised reconstruction techniques based on implicit neural representation (INR), such as NeRP, CoIL, and SCOPE, have shown unique capabilities in CT linear inverse imaging. In this work, we propose a novel unsupervised density neural representation (Diner) to tackle the challenging problem of CT metal artifacts when scanned objects contain metals. The drastic variation of linear attenuation coefficients (LACs) of metals over X-ray spectra leads to a nonlinear beam hardening effect (BHE) in CT measurements. Recovering CT images from metal-affected measurements therefore poses a complicated nonlinear inverse problem. Existing metal artifact reduction (MAR) techniques mostly formulate the MAR as an image inpainting task, which ignores the energy-induced BHE and produces suboptimal performance. Instead, our Diner introduces an energy-dependent polychromatic CT forward model to the INR framework, addressing the nonlinear nature of the MAR problem. Specifically, we decompose the energy-dependent LACs into energy-independent densities and energy-dependent mass attenuation coefficients (MACs) by fully considering the physical model of X-ray absorption. Using the densities as pivot variables and the MACs as known prior knowledge, the LACs can be accurately reconstructed from the raw measurements. Technically, we represent the unknown density map as an implicit function of coordinates. Combined with a novel differentiable forward model simulating the physical acquisition from the densities to the measurements, our Diner optimizes a multi-layer perception network to approximate the implicit function by minimizing predicted errors between the estimated and real measurements. Experimental results on simulated and real datasets confirm the superiority of our unsupervised Diner against popular supervised techniques in MAR performance and robustness.
* 13 pages
Via
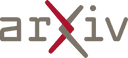
Nov 16, 2023
Abstract:Cone Beam Computed Tomography (CBCT) plays a key role in dental diagnosis and surgery. However, the metal teeth implants could bring annoying metal artifacts during the CBCT imaging process, interfering diagnosis and downstream processing such as tooth segmentation. In this paper, we develop an efficient Transformer to perform metal artifacts reduction (MAR) from dental CBCT images. The proposed MAR Transformer (MARformer) reduces computation complexity in the multihead self-attention by a new Dimension-Reduced Self-Attention (DRSA) module, based on that the CBCT images have globally similar structure. A Patch-wise Perceptive Feed Forward Network (P2FFN) is also proposed to perceive local image information for fine-grained restoration. Experimental results on CBCT images with synthetic and real-world metal artifacts show that our MARformer is efficient and outperforms previous MAR methods and two restoration Transformers.
Via
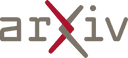
Mar 27, 2024
Abstract:Deep learning based approaches have been used to improve image quality in cone-beam computed tomography (CBCT), a medical imaging technique often used in applications such as image-guided radiation therapy, implant dentistry or orthopaedics. In particular, while deep learning methods have been applied to reduce various types of CBCT image artifacts arising from motion, metal objects, or low-dose acquisition, a comprehensive review summarizing the successes and shortcomings of these approaches, with a primary focus on the type of artifacts rather than the architecture of neural networks, is lacking in the literature. In this review, the data generation and simulation pipelines, and artifact reduction techniques are specifically investigated for each type of artifact. We provide an overview of deep learning techniques that have successfully been shown to reduce artifacts in 3D, as well as in time-resolved (4D) CBCT through the use of projection- and/or volume-domain optimizations, or by introducing neural networks directly within the CBCT reconstruction algorithms. Research gaps are identified to suggest avenues for future exploration. One of the key findings of this work is an observed trend towards the use of generative models including GANs and score-based or diffusion models, accompanied with the need for more diverse and open training datasets and simulations.
* IEEE Access, vol. 12, pp. 10281-10295, 2024
* 16 pages, 4 figures, 1 Table, published in IEEE Access Journal
Via
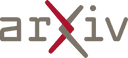
Jul 28, 2023
Abstract:CT images corrupted by metal artifacts have serious negative effects on clinical diagnosis. Considering the difficulty of collecting paired data with ground truth in clinical settings, unsupervised methods for metal artifact reduction are of high interest. However, it is difficult for previous unsupervised methods to retain structural information from CT images while handling the non-local characteristics of metal artifacts. To address these challenges, we proposed a novel Dense Transformer based Enhanced Coding Network (DTEC-Net) for unsupervised metal artifact reduction. Specifically, we introduce a Hierarchical Disentangling Encoder, supported by the high-order dense process, and transformer to obtain densely encoded sequences with long-range correspondence. Then, we present a second-order disentanglement method to improve the dense sequence's decoding process. Extensive experiments and model discussions illustrate DTEC-Net's effectiveness, which outperforms the previous state-of-the-art methods on a benchmark dataset, and greatly reduces metal artifacts while restoring richer texture details.
Via
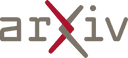