What is Imitation Learning? Imitation learning is a framework for learning a behavior policy from demonstrations. Usually, demonstrations are presented in the form of state-action trajectories, with each pair indicating the action to take at the state being visited. In order to learn the behavior policy, the demonstrated actions are usually utilized in two ways. The first, known as Behavior Cloning (BC), treats the action as the target label for each state, and then learns a generalized mapping from states to actions in a supervised manner. Another way, known as Inverse Reinforcement Learning (IRL), views the demonstrated actions as a sequence of decisions, and aims at finding a reward/cost function under which the demonstrated decisions are optimal.
Papers and Code
Jul 09, 2025
Abstract:Imitation Learning from Observation (IfO) offers a powerful way to learn behaviors at large-scale: Unlike behavior cloning or offline reinforcement learning, IfO can leverage action-free demonstrations and thus circumvents the need for costly action-labeled demonstrations or reward functions. However, current IfO research focuses on idealized scenarios with mostly bimodal-quality data distributions, restricting the meaningfulness of the results. In contrast, this paper investigates more nuanced distributions and introduces a method to learn from such data, moving closer to a paradigm in which imitation learning can be performed iteratively via self-improvement. Our method adapts RL-based imitation learning to action-free demonstrations, using a value function to transfer information between expert and non-expert data. Through comprehensive evaluation, we delineate the relation between different data distributions and the applicability of algorithms and highlight the limitations of established methods. Our findings provide valuable insights for developing more robust and practical IfO techniques on a path to scalable behaviour learning.
Via
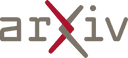
Jul 09, 2025
Abstract:This article introduces an imitation learning method for learning maximum entropy policies that comply with constraints demonstrated by expert trajectories executing a task. The formulation of the method takes advantage of results connecting performance to bounds for the KL-divergence between demonstrated and learned policies, and its objective is rigorously justified through a connection to a probabilistic inference framework for reinforcement learning, incorporating the reinforcement learning objective and the objective to abide by constraints in an entropy maximization setting. The proposed algorithm optimizes the learning objective with dual gradient descent, supporting effective and stable training. Experiments show that the proposed method can learn effective policy models for constraints-abiding behaviour, in settings with multiple constraints of different types, accommodating different modalities of demonstrated behaviour, and with abilities to generalize.
Via
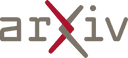
Jul 09, 2025
Abstract:Visual imitation learning is effective for robots to learn versatile tasks. However, many existing methods rely on behavior cloning with supervised historical trajectories, limiting their 3D spatial and 4D spatiotemporal awareness. Consequently, these methods struggle to capture the 3D structures and 4D spatiotemporal relationships necessary for real-world deployment. In this work, we propose 4D Diffusion Policy (DP4), a novel visual imitation learning method that incorporates spatiotemporal awareness into diffusion-based policies. Unlike traditional approaches that rely on trajectory cloning, DP4 leverages a dynamic Gaussian world model to guide the learning of 3D spatial and 4D spatiotemporal perceptions from interactive environments. Our method constructs the current 3D scene from a single-view RGB-D observation and predicts the future 3D scene, optimizing trajectory generation by explicitly modeling both spatial and temporal dependencies. Extensive experiments across 17 simulation tasks with 173 variants and 3 real-world robotic tasks demonstrate that the 4D Diffusion Policy (DP4) outperforms baseline methods, improving the average simulation task success rate by 16.4% (Adroit), 14% (DexArt), and 6.45% (RLBench), and the average real-world robotic task success rate by 8.6%.
Via
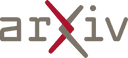
Jul 08, 2025
Abstract:In recent years, the advancement of imitation learning has led to increased interest in teleoperating low-cost manipulators to collect demonstration data. However, most existing systems rely on unilateral control, which only transmits target position values. While this approach is easy to implement and suitable for slow, non-contact tasks, it struggles with fast or contact-rich operations due to the absence of force feedback. This work demonstrates that fast teleoperation with force feedback is feasible even with force-sensorless, low-cost manipulators by leveraging 4-channel bilateral control. Based on accurately identified manipulator dynamics, our method integrates nonlinear terms compensation, velocity and external force estimation, and variable gain corresponding to inertial variation. Furthermore, using data collected by 4-channel bilateral control, we show that incorporating force information into both the input and output of learned policies improves performance in imitation learning. These results highlight the practical effectiveness of our system for high-fidelity teleoperation and data collection on affordable hardware.
* 19 pages, 8 figures, Submitted to CoRL 2025
Via
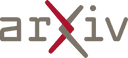
Jul 08, 2025
Abstract:Evaluating and comparing the performance of autonomous Humanoid Robots is challenging, as success rate metrics are difficult to reproduce and fail to capture the complexity of robot movement trajectories, critical in Human-Robot Interaction and Collaboration (HRIC). To address these challenges, we propose a general evaluation framework that measures the quality of Imitation Learning (IL) methods by focusing on trajectory performance. We devise the Neural Meta Evaluator (NeME), a deep learning model trained to classify actions from robot joint trajectories. NeME serves as a meta-evaluator to compare the performance of robot control policies, enabling policy evaluation without requiring human involvement in the loop. We validate our framework on ergoCub, a humanoid robot, using teleoperation data and comparing IL methods tailored to the available platform. The experimental results indicate that our method is more aligned with the success rate obtained on the robot than baselines, offering a reproducible, systematic, and insightful means for comparing the performance of multimodal imitation learning approaches in complex HRI tasks.
Via
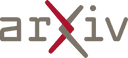
Jul 08, 2025
Abstract:Current language-guided robotic manipulation systems often require low-level action-labeled datasets for imitation learning. While object-centric flow prediction methods mitigate this issue, they remain limited to scenarios involving rigid objects with clear displacement and minimal occlusion. In this work, we present Embodiment-Centric Flow (EC-Flow), a framework that directly learns manipulation from action-unlabeled videos by predicting embodiment-centric flow. Our key insight is that incorporating the embodiment's inherent kinematics significantly enhances generalization to versatile manipulation scenarios, including deformable object handling, occlusions, and non-object-displacement tasks. To connect the EC-Flow with language instructions and object interactions, we further introduce a goal-alignment module by jointly optimizing movement consistency and goal-image prediction. Moreover, translating EC-Flow to executable robot actions only requires a standard robot URDF (Unified Robot Description Format) file to specify kinematic constraints across joints, which makes it easy to use in practice. We validate EC-Flow on both simulation (Meta-World) and real-world tasks, demonstrating its state-of-the-art performance in occluded object handling (62% improvement), deformable object manipulation (45% improvement), and non-object-displacement tasks (80% improvement) than prior state-of-the-art object-centric flow methods. For more information, see our project website at https://ec-flow1.github.io .
* Accepted at ICCV 2025
Via
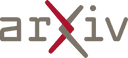
Jul 03, 2025
Abstract:Recent advancements in vision-language models (VLMs) for common-sense reasoning have led to the development of vision-language-action (VLA) models, enabling robots to perform generalized manipulation. Although existing autoregressive VLA methods design a specific architecture like dual-system to leverage large-scale pretrained knowledge, they tend to capture static information, often neglecting the dynamic aspects vital for embodied tasks. To this end, we propose TriVLA, a unified Vision-Language-Action model with a triple-system architecture for general robot control. The vision-language module (System 2) interprets the environment through vision and language instructions. The dynamics perception module (System 3) inherently produces visual representations that encompass both current static information and predicted future dynamics, thereby providing valuable guidance for policy learning. TriVLA utilizes pre-trained VLM model and fine-tunes pre-trained video foundation model on robot datasets along with internet human manipulation data. The subsequent policy learning module (System 1) generates fluid motor actions in real time. Experimental evaluation demonstrates that TriVLA operates at approximately 36 Hz and surpasses state-of-the-art imitation learning baselines on standard simulation benchmarks as well as challenging real-world manipulation tasks.
Via
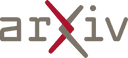
Jul 01, 2025
Abstract:This paper presents a novel satellite attitude control framework that integrates Soft Actor-Critic (SAC) reinforcement learning with Generative Adversarial Imitation Learning (GAIL) to achieve robust performance under various unknown perturbations. Traditional control techniques often rely on precise system models and are sensitive to parameter uncertainties and external perturbations. To overcome these limitations, we first develop a SAC-based expert controller that demonstrates improved resilience against actuator failures, sensor noise, and attitude misalignments, outperforming our previous results in several challenging scenarios. We then use GAIL to train a learner policy that imitates the expert's trajectories, thereby reducing training costs and improving generalization through expert demonstrations. Preliminary experiments under single and combined perturbations show that the SAC expert can rotate the antenna to a specified direction and keep the antenna orientation reliably stable in most of the listed perturbations. Additionally, the GAIL learner can imitate most of the features from the trajectories generated by the SAC expert. Comparative evaluations and ablation studies confirm the effectiveness of the SAC algorithm and reward shaping. The integration of GAIL further reduces sample complexity and demonstrates promising imitation capabilities, paving the way for more intelligent and autonomous spacecraft control systems.
* 2025 AAS/AIAA Astrodynamics Specialist Conference
Via
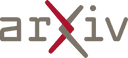
Jul 02, 2025
Abstract:Trajectory planning in robotics is understood as generating a sequence of joint configurations that will lead a robotic agent, or its manipulator, from an initial state to the desired final state, thus completing a manipulation task while considering constraints like robot kinematics and the environment. Typically, this is achieved via sampling-based planners, which are computationally intensive. Recent advances demonstrate that trajectory planning can also be performed by supervised sequence learning of trajectories, often requiring only a single or fixed number of passes through a neural architecture, thus ensuring a bounded computation time. Such fully supervised approaches, however, perform imitation learning; they do not learn based on whether the trajectories can successfully reach a goal, but try to reproduce observed trajectories. In our work, we build on this approach and propose a cognitively inspired self-supervised learning scheme based on a recurrent architecture for building a trajectory model. We evaluate the feasibility of the proposed method on a task of kinematic planning for a robotic arm. The results suggest that the model is able to learn to generate trajectories only using given paired forward and inverse kinematics models, and indicate that this novel method could facilitate planning for more complex manipulation tasks requiring adaptive solutions.
* 12 pages, 4 figures, 2 tables. To be published in 2025 International
Conference on Artificial Neural Networks (ICANN) proceedings. This research
was funded by the Horizon Europe project TERAIS, GA no. 101079338, and in
part by the Slovak Grant Agency for Science (VEGA), project 1/0373/23
Via
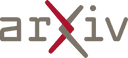
Jul 02, 2025
Abstract:Dexterous teleoperation plays a crucial role in robotic manipulation for real-world data collection and remote robot control. Previous dexterous teleoperation mostly relies on hand retargeting to closely mimic human hand postures. However, these approaches may fail to fully leverage the inherent dexterity of dexterous hands, which can execute unique actions through their structural advantages compared to human hands. To address this limitation, we propose TypeTele, a type-guided dexterous teleoperation system, which enables dexterous hands to perform actions that are not constrained by human motion patterns. This is achieved by introducing dexterous manipulation types into the teleoperation system, allowing operators to employ appropriate types to complete specific tasks. To support this system, we build an extensible dexterous manipulation type library to cover comprehensive dexterous postures used in manipulation tasks. During teleoperation, we employ a MLLM (Multi-modality Large Language Model)-assisted type retrieval module to identify the most suitable manipulation type based on the specific task and operator commands. Extensive experiments of real-world teleoperation and imitation learning demonstrate that the incorporation of manipulation types significantly takes full advantage of the dexterous robot's ability to perform diverse and complex tasks with higher success rates.
Via
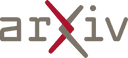