Topic:3D Scene Reconstruction
What is 3D Scene Reconstruction? 3D scene reconstruction is the process of creating 3D models of scenes or environments from images or videos.
Papers and Code
May 04, 2025
Abstract:Traditional 3D garment creation requires extensive manual operations, resulting in time and labor costs. Recently, 3D Gaussian Splatting has achieved breakthrough progress in 3D scene reconstruction and rendering, attracting widespread attention and opening new pathways for 3D garment reconstruction. However, due to the unstructured and irregular nature of Gaussian primitives, it is difficult to reconstruct high-fidelity, non-watertight 3D garments. In this paper, we present GarmentGS, a dense point cloud-guided method that can reconstruct high-fidelity garment surfaces with high geometric accuracy and generate non-watertight, single-layer meshes. Our method introduces a fast dense point cloud reconstruction module that can complete garment point cloud reconstruction in 10 minutes, compared to traditional methods that require several hours. Furthermore, we use dense point clouds to guide the movement, flattening, and rotation of Gaussian primitives, enabling better distribution on the garment surface to achieve superior rendering effects and geometric accuracy. Through numerical and visual comparisons, our method achieves fast training and real-time rendering while maintaining competitive quality.
Via
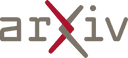
May 04, 2025
Abstract:Recovering 3D information from scenes via multi-view stereo reconstruction (MVS) and novel view synthesis (NVS) is inherently challenging, particularly in scenarios involving sparse-view setups. The advent of 3D Gaussian Splatting (3DGS) enabled real-time, photorealistic NVS. Following this, 2D Gaussian Splatting (2DGS) leveraged perspective accurate 2D Gaussian primitive rasterization to achieve accurate geometry representation during rendering, improving 3D scene reconstruction while maintaining real-time performance. Recent approaches have tackled the problem of sparse real-time NVS using 3DGS within a generalizable, MVS-based learning framework to regress 3D Gaussian parameters. Our work extends this line of research by addressing the challenge of generalizable sparse 3D reconstruction and NVS jointly, and manages to perform successfully at both tasks. We propose an MVS-based learning pipeline that regresses 2DGS surface element parameters in a feed-forward fashion to perform 3D shape reconstruction and NVS from sparse-view images. We further show that our generalizable pipeline can benefit from preexisting foundational multi-view deep visual features. The resulting model attains the state-of-the-art results on the DTU sparse 3D reconstruction benchmark in terms of Chamfer distance to ground-truth, as-well as state-of-the-art NVS. It also demonstrates strong generalization on the BlendedMVS and Tanks and Temples datasets. We note that our model outperforms the prior state-of-the-art in feed-forward sparse view reconstruction based on volume rendering of implicit representations, while offering an almost 2 orders of magnitude higher inference speed.
Via
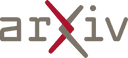
May 03, 2025
Abstract:Underwater visual enhancement (UVE) and underwater 3D reconstruction pose significant challenges in computer vision and AI-based tasks due to complex imaging conditions in aquatic environments. Despite the development of numerous enhancement algorithms, a comprehensive and systematic review covering both UVE and underwater 3D reconstruction remains absent. To advance research in these areas, we present an in-depth review from multiple perspectives. First, we introduce the fundamental physical models, highlighting the peculiarities that challenge conventional techniques. We survey advanced methods for visual enhancement and 3D reconstruction specifically designed for underwater scenarios. The paper assesses various approaches from non-learning methods to advanced data-driven techniques, including Neural Radiance Fields and 3D Gaussian Splatting, discussing their effectiveness in handling underwater distortions. Finally, we conduct both quantitative and qualitative evaluations of state-of-the-art UVE and underwater 3D reconstruction algorithms across multiple benchmark datasets. Finally, we highlight key research directions for future advancements in underwater vision.
Via
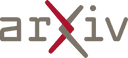
May 03, 2025
Abstract:Underwater scene reconstruction is a critical tech-nology for underwater operations, enabling the generation of 3D models from images captured by underwater platforms. However, the quality of underwater images is often degraded due to medium interference, which limits the effectiveness of Structure-from-Motion (SfM) pose estimation, leading to subsequent reconstruction failures. Additionally, SfM methods typically operate at slower speeds, further hindering their applicability in real-time scenarios. In this paper, we introduce AquaGS, an SfM-free underwater scene reconstruction model based on the SeaThru algorithm, which facilitates rapid and accurate separation of scene details and medium features. Our approach initializes Gaussians by integrating state-of-the-art multi-view stereo (MVS) technology, employs implicit Neural Radiance Fields (NeRF) for rendering translucent media and utilizes the latest explicit 3D Gaussian Splatting (3DGS) technique to render object surfaces, which effectively addresses the limitations of traditional methods and accurately simulates underwater optical phenomena. Experimental results on the data set and the robot platform show that our model can complete high-precision reconstruction in 30 seconds with only 3 image inputs, significantly enhancing the practical application of the algorithm in robotic platforms.
Via
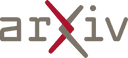
May 03, 2025
Abstract:Accurate and high-fidelity driving scene reconstruction relies on fully leveraging scene information as conditioning. However, existing approaches, which primarily use 3D bounding boxes and binary maps for foreground and background control, fall short in capturing the complexity of the scene and integrating multi-modal information. In this paper, we propose DualDiff, a dual-branch conditional diffusion model designed to enhance multi-view driving scene generation. We introduce Occupancy Ray Sampling (ORS), a semantic-rich 3D representation, alongside numerical driving scene representation, for comprehensive foreground and background control. To improve cross-modal information integration, we propose a Semantic Fusion Attention (SFA) mechanism that aligns and fuses features across modalities. Furthermore, we design a foreground-aware masked (FGM) loss to enhance the generation of tiny objects. DualDiff achieves state-of-the-art performance in FID score, as well as consistently better results in downstream BEV segmentation and 3D object detection tasks.
* 8 pages, 6 figures,
Via
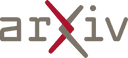
May 02, 2025
Abstract:We present the first scene-update aerial path planning algorithm specifically designed for detecting and updating change areas in urban environments. While existing methods for large-scale 3D urban scene reconstruction focus on achieving high accuracy and completeness, they are inefficient for scenarios requiring periodic updates, as they often re-explore and reconstruct entire scenes, wasting significant time and resources on unchanged areas. To address this limitation, our method leverages prior reconstructions and change probability statistics to guide UAVs in detecting and focusing on areas likely to have changed. Our approach introduces a novel changeability heuristic to evaluate the likelihood of changes, driving the planning of two flight paths: a prior path informed by static priors and a dynamic real-time path that adapts to newly detected changes. The framework integrates surface sampling and candidate view generation strategies, ensuring efficient coverage of change areas with minimal redundancy. Extensive experiments on real-world urban datasets demonstrate that our method significantly reduces flight time and computational overhead, while maintaining high-quality updates comparable to full-scene re-exploration and reconstruction. These contributions pave the way for efficient, scalable, and adaptive UAV-based scene updates in complex urban environments.
Via
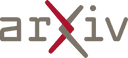
May 02, 2025
Abstract:Online reconstruction of dynamic scenes is significant as it enables learning scenes from live-streaming video inputs, while existing offline dynamic reconstruction methods rely on recorded video inputs. However, previous online reconstruction approaches have primarily focused on efficiency and rendering quality, overlooking the temporal consistency of their results, which often contain noticeable artifacts in static regions. This paper identifies that errors such as noise in real-world recordings affect temporal inconsistency in online reconstruction. We propose a method that enhances temporal consistency in online reconstruction from observations with temporal inconsistency which is inevitable in cameras. We show that our method restores the ideal observation by subtracting the learned error. We demonstrate that applying our method to various baselines significantly enhances both temporal consistency and rendering quality across datasets. Code, video results, and checkpoints are available at https://bbangsik13.github.io/OR2.
Via
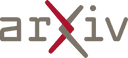
May 02, 2025
Abstract:Text-driven object insertion in 3D scenes is an emerging task that enables intuitive scene editing through natural language. However, existing 2D editing-based methods often rely on spatial priors such as 2D masks or 3D bounding boxes, and they struggle to ensure consistency of the inserted object. These limitations hinder flexibility and scalability in real-world applications. In this paper, we propose FreeInsert, a novel framework that leverages foundation models including MLLMs, LGMs, and diffusion models to disentangle object generation from spatial placement. This enables unsupervised and flexible object insertion in 3D scenes without spatial priors. FreeInsert starts with an MLLM-based parser that extracts structured semantics, including object types, spatial relationships, and attachment regions, from user instructions. These semantics guide both the reconstruction of the inserted object for 3D consistency and the learning of its degrees of freedom. We leverage the spatial reasoning capabilities of MLLMs to initialize object pose and scale. A hierarchical, spatially aware refinement stage further integrates spatial semantics and MLLM-inferred priors to enhance placement. Finally, the appearance of the object is improved using the inserted-object image to enhance visual fidelity. Experimental results demonstrate that FreeInsert achieves semantically coherent, spatially precise, and visually realistic 3D insertions without relying on spatial priors, offering a user-friendly and flexible editing experience.
Via
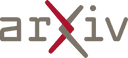
May 01, 2025
Abstract:We present RayZer, a self-supervised multi-view 3D Vision model trained without any 3D supervision, i.e., camera poses and scene geometry, while exhibiting emerging 3D awareness. Concretely, RayZer takes unposed and uncalibrated images as input, recovers camera parameters, reconstructs a scene representation, and synthesizes novel views. During training, RayZer relies solely on its self-predicted camera poses to render target views, eliminating the need for any ground-truth camera annotations and allowing RayZer to be trained with 2D image supervision. The emerging 3D awareness of RayZer is attributed to two key factors. First, we design a self-supervised framework, which achieves 3D-aware auto-encoding of input images by disentangling camera and scene representations. Second, we design a transformer-based model in which the only 3D prior is the ray structure, connecting camera, pixel, and scene simultaneously. RayZer demonstrates comparable or even superior novel view synthesis performance than ``oracle'' methods that rely on pose annotations in both training and testing. Project: https://hwjiang1510.github.io/RayZer/
Via
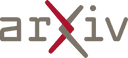
Apr 29, 2025
Abstract:Accurate and robust 3D scene reconstruction from casual, in-the-wild videos can significantly simplify robot deployment to new environments. However, reliable camera pose estimation and scene reconstruction from such unconstrained videos remains an open challenge. Existing visual-only SLAM methods perform well on benchmark datasets but struggle with real-world footage which often exhibits uncontrolled motion including rapid rotations and pure forward movements, textureless regions, and dynamic objects. We analyze the limitations of current methods and introduce a robust pipeline designed to improve 3D reconstruction from casual videos. We build upon recent deep visual odometry methods but increase robustness in several ways. Camera intrinsics are automatically recovered from the first few frames using structure-from-motion. Dynamic objects and less-constrained areas are masked with a predictive model. Additionally, we leverage monocular depth estimates to regularize bundle adjustment, mitigating errors in low-parallax situations. Finally, we integrate place recognition and loop closure to reduce long-term drift and refine both intrinsics and pose estimates through global bundle adjustment. We demonstrate large-scale contiguous 3D models from several online videos in various environments. In contrast, baseline methods typically produce locally inconsistent results at several points, producing separate segments or distorted maps. In lieu of ground-truth pose data, we evaluate map consistency, execution time and visual accuracy of re-rendered NeRF models. Our proposed system establishes a new baseline for visual reconstruction from casual uncontrolled videos found online, demonstrating more consistent reconstructions over longer sequences of in-the-wild videos than previously achieved.
* fix the overview figure
Via
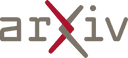