Jun 10, 2025
Abstract:In this article, we present a novel multimodal feedback framework called MOSAIC-F, an acronym for a data-driven Framework that integrates Multimodal Learning Analytics (MMLA), Observations, Sensors, Artificial Intelligence (AI), and Collaborative assessments for generating personalized feedback on student learning activities. This framework consists of four key steps. First, peers and professors' assessments are conducted through standardized rubrics (that include both quantitative and qualitative evaluations). Second, multimodal data are collected during learning activities, including video recordings, audio capture, gaze tracking, physiological signals (heart rate, motion data), and behavioral interactions. Third, personalized feedback is generated using AI, synthesizing human-based evaluations and data-based multimodal insights such as posture, speech patterns, stress levels, and cognitive load, among others. Finally, students review their own performance through video recordings and engage in self-assessment and feedback visualization, comparing their own evaluations with peers and professors' assessments, class averages, and AI-generated recommendations. By combining human-based and data-based evaluation techniques, this framework enables more accurate, personalized and actionable feedback. We tested MOSAIC-F in the context of improving oral presentation skills.
* Accepted in LASI Spain 25: Learning Analytics Summer Institute Spain
2025
Via
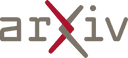