Apr 14, 2025
Abstract:Recent Open-Vocabulary Semantic Segmentation (OVSS) models extend the CLIP model to segmentation while maintaining the use of multiple templates (e.g., a photo of <class>, a sketch of a <class>, etc.) for constructing class-wise averaged text embeddings, acting as a classifier. In this paper, we challenge this status quo and investigate the impact of templates for OVSS. Empirically, we observe that for each class, there exist single-template classifiers significantly outperforming the conventional averaged classifier. We refer to them as class-experts. Given access to unlabeled images and without any training involved, we estimate these experts by leveraging the class-wise prediction entropy of single-template classifiers, selecting as class-wise experts those which yield the lowest entropy. All experts, each specializing in a specific class, collaborate in a newly proposed fusion method to generate more accurate OVSS predictions. Our plug-and-play method, coined FLOSS, is orthogonal and complementary to existing OVSS methods, offering a ''free lunch'' to systematically improve OVSS without labels and additional training. Extensive experiments demonstrate that FLOSS consistently boosts state-of-the-art methods on various OVSS benchmarks. Moreover, the selected expert templates can generalize well from one dataset to others sharing the same semantic categories, yet exhibiting distribution shifts. Additionally, we obtain satisfactory improvements under a low-data regime, where only a few unlabeled images are available. Our code is available at https://github.com/yasserben/FLOSS .
Via
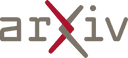