Aug 31, 2018
Abstract:Many photography websites such as Flickr, 500px, Unsplash, and Adobe Behance are used by amateur and professional photography enthusiasts. Unlike content-based image search, such users of photography websites are not just looking for photos with certain content, but more generally for photos with a certain photographic "aesthetic". In this context, we explore personalized photo recommendation and propose two aesthetic feature extraction methods based on (i) color space and (ii) deep style transfer embeddings. Using a dataset from 500px, we evaluate how these features can be best leveraged by collaborative filtering methods and show that (ii) provides a significant boost in photo recommendation performance.
* In Proceedings of the Late-Breaking Results track part of the Twelfth
ACM Conference on Recommender Systems, Vancouver, BC, Canada, October 6,
2018, 2 pages
Via
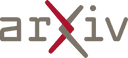