What is music generation? Music generation is the task of generating music or music-like sounds from a model or algorithm.
Papers and Code
May 05, 2025
Abstract:Independent learners often struggle with sustaining focus and emotional regulation in unstructured or distracting settings. Although some rely on ambient aids such as music, ASMR, or visual backgrounds to support concentration, these tools are rarely integrated into cohesive, learner-centered systems. Moreover, existing educational technologies focus primarily on content adaptation and feedback, overlooking the emotional and sensory context in which learning takes place. Large language models have demonstrated powerful multimodal capabilities including the ability to generate and adapt text, audio, and visual content. Educational research has yet to fully explore their potential in creating personalized audiovisual learning environments. To address this gap, we introduce an AI-powered system that uses LLMs to generate personalized multisensory study environments. Users select or generate customized visual themes (e.g., abstract vs. realistic, static vs. animated) and auditory elements (e.g., white noise, ambient ASMR, familiar vs. novel sounds) to create immersive settings aimed at reducing distraction and enhancing emotional stability. Our primary research question investigates how combinations of personalized audiovisual elements affect learner cognitive load and engagement. Using a mixed-methods design that incorporates biometric measures and performance outcomes, this study evaluates the effectiveness of LLM-driven sensory personalization. The findings aim to advance emotionally responsive educational technologies and extend the application of multimodal LLMs into the sensory dimension of self-directed learning.
Via
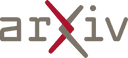
Apr 09, 2025
Abstract:Recent advances in generative models have made it possible to create high-quality, coherent music, with some systems delivering production-level output. Yet, most existing models focus solely on generating music from scratch, limiting their usefulness for musicians who want to integrate such models into a human, iterative composition workflow. In this paper we introduce STAGE, our STemmed Accompaniment GEneration model, fine-tuned from the state-of-the-art MusicGen to generate single-stem instrumental accompaniments conditioned on a given mixture. Inspired by instruction-tuning methods for language models, we extend the transformer's embedding matrix with a context token, enabling the model to attend to a musical context through prefix-based conditioning. Compared to the baselines, STAGE yields accompaniments that exhibit stronger coherence with the input mixture, higher audio quality, and closer alignment with textual prompts. Moreover, by conditioning on a metronome-like track, our framework naturally supports tempo-constrained generation, achieving state-of-the-art alignment with the target rhythmic structure--all without requiring any additional tempo-specific module. As a result, STAGE offers a practical, versatile tool for interactive music creation that can be readily adopted by musicians in real-world workflows.
Via
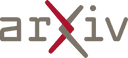
Mar 27, 2025
Abstract:Vision-to-music Generation, including video-to-music and image-to-music tasks, is a significant branch of multimodal artificial intelligence demonstrating vast application prospects in fields such as film scoring, short video creation, and dance music synthesis. However, compared to the rapid development of modalities like text and images, research in vision-to-music is still in its preliminary stage due to its complex internal structure and the difficulty of modeling dynamic relationships with video. Existing surveys focus on general music generation without comprehensive discussion on vision-to-music. In this paper, we systematically review the research progress in the field of vision-to-music generation. We first analyze the technical characteristics and core challenges for three input types: general videos, human movement videos, and images, as well as two output types of symbolic music and audio music. We then summarize the existing methodologies on vision-to-music generation from the architecture perspective. A detailed review of common datasets and evaluation metrics is provided. Finally, we discuss current challenges and promising directions for future research. We hope our survey can inspire further innovation in vision-to-music generation and the broader field of multimodal generation in academic research and industrial applications. To follow latest works and foster further innovation in this field, we are continuously maintaining a GitHub repository at https://github.com/wzk1015/Awesome-Vision-to-Music-Generation.
Via
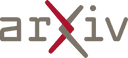
Mar 28, 2025
Abstract:Conditional diffusion models have gained increasing attention since their impressive results for cross-modal synthesis, where the strong alignment between conditioning input and generated output can be achieved by training a time-conditioned U-Net augmented with cross-attention mechanism. In this paper, we focus on the problem of generating music synchronized with rhythmic visual cues of the given dance video. Considering that bi-directional guidance is more beneficial for training a diffusion model, we propose to enhance the quality of generated music and its synchronization with dance videos by adopting both positive rhythmic information and negative ones (PN-Diffusion) as conditions, where a dual diffusion and reverse processes is devised. Specifically, to train a sequential multi-modal U-Net structure, PN-Diffusion consists of a noise prediction objective for positive conditioning and an additional noise prediction objective for negative conditioning. To accurately define and select both positive and negative conditioning, we ingeniously utilize temporal correlations in dance videos, capturing positive and negative rhythmic cues by playing them forward and backward, respectively. Through subjective and objective evaluations of input-output correspondence in terms of dance-music beat alignment and the quality of generated music, experimental results on the AIST++ and TikTok dance video datasets demonstrate that our model outperforms SOTA dance-to-music generation models.
Via
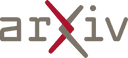
May 05, 2025
Abstract:Biofeedback is being used more recently as a general control paradigm for human-computer interfaces (HCIs). While biofeedback especially from breath has seen increasing uptake as a controller for novel musical interfaces, new interfaces for musical expression (NIMEs), the community has not given as much attention to the heart. The heart is just as intimate a part of music as breath and it is argued that the heart determines our perception of time and so indirectly our perception of music. Inspired by this I demonstrate a photoplethysmogram (PPG)-based NIME controller using heart rate as a 1D control parameter to transform the qualities of sounds in real-time over a Bluetooth wireless HCI. I apply time scaling to "warp" audio buffers inbound to the sound card, and play these transformed audio buffers back to the listener wearing the PPG sensor, creating a hypothetical perceptual biofeedback loop: changes in sound change heart rate to change PPG measurements to change sound. I discuss how a sound-heart-PPG biofeedback loop possibly affords greater control and/or variety of movements with a 1D controller, how controlling the space and/or time scale of sound playback with biofeedback makes for possibilities in performance ambience, and I briefly discuss generative latent spaces as a possible way to extend a 1D PPG control space.
Via
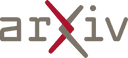
Mar 25, 2025
Abstract:Autoregressive (AR) models have demonstrated impressive capabilities in generating high-fidelity music. However, the conventional next-token prediction paradigm in AR models does not align with the human creative process in music composition, potentially compromising the musicality of generated samples. To overcome this limitation, we introduce MusiCoT, a novel chain-of-thought (CoT) prompting technique tailored for music generation. MusiCoT empowers the AR model to first outline an overall music structure before generating audio tokens, thereby enhancing the coherence and creativity of the resulting compositions. By leveraging the contrastive language-audio pretraining (CLAP) model, we establish a chain of "musical thoughts", making MusiCoT scalable and independent of human-labeled data, in contrast to conventional CoT methods. Moreover, MusiCoT allows for in-depth analysis of music structure, such as instrumental arrangements, and supports music referencing -- accepting variable-length audio inputs as optional style references. This innovative approach effectively addresses copying issues, positioning MusiCoT as a vital practical method for music prompting. Our experimental results indicate that MusiCoT consistently achieves superior performance across both objective and subjective metrics, producing music quality that rivals state-of-the-art generation models. Our samples are available at https://MusiCoT.github.io/.
* Preprint
Via
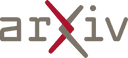
Apr 21, 2025
Abstract:We present Distributional RewArds for Generative OptimizatioN (DRAGON), a versatile framework for fine-tuning media generation models towards a desired outcome. Compared with traditional reinforcement learning with human feedback (RLHF) or pairwise preference approaches such as direct preference optimization (DPO), DRAGON is more flexible. It can optimize reward functions that evaluate either individual examples or distributions of them, making it compatible with a broad spectrum of instance-wise, instance-to-distribution, and distribution-to-distribution rewards. Leveraging this versatility, we construct novel reward functions by selecting an encoder and a set of reference examples to create an exemplar distribution. When cross-modality encoders such as CLAP are used, the reference examples may be of a different modality (e.g., text versus audio). Then, DRAGON gathers online and on-policy generations, scores them to construct a positive demonstration set and a negative set, and leverages the contrast between the two sets to maximize the reward. For evaluation, we fine-tune an audio-domain text-to-music diffusion model with 20 different reward functions, including a custom music aesthetics model, CLAP score, Vendi diversity, and Frechet audio distance (FAD). We further compare instance-wise (per-song) and full-dataset FAD settings while ablating multiple FAD encoders and reference sets. Over all 20 target rewards, DRAGON achieves an 81.45% average win rate. Moreover, reward functions based on exemplar sets indeed enhance generations and are comparable to model-based rewards. With an appropriate exemplar set, DRAGON achieves a 60.95% human-voted music quality win rate without training on human preference annotations. As such, DRAGON exhibits a new approach to designing and optimizing reward functions for improving human-perceived quality. Sound examples at https://ml-dragon.github.io/web.
Via
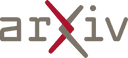
Apr 30, 2025
Abstract:Short video platforms like YouTube Shorts and TikTok face significant copyright compliance challenges, as infringers frequently embed arbitrary background music (BGM) to obscure original soundtracks (OST) and evade content originality detection. To tackle this issue, we propose a novel pipeline that integrates Music Source Separation (MSS) and cross-modal video-music retrieval (CMVMR). Our approach effectively separates arbitrary BGM from the original OST, enabling the restoration of authentic video audio tracks. To support this work, we introduce two domain-specific datasets: OASD-20K for audio separation and OSVAR-160 for pipeline evaluation. OASD-20K contains 20,000 audio clips featuring mixed BGM and OST pairs, while OSVAR160 is a unique benchmark dataset comprising 1,121 video and mixed-audio pairs, specifically designed for short video restoration tasks. Experimental results demonstrate that our pipeline not only removes arbitrary BGM with high accuracy but also restores OSTs, ensuring content integrity. This approach provides an ethical and scalable solution to copyright challenges in user-generated content on short video platforms.
* will be presented in IJCAI 2025, 9 pages, 4 tables, 3 figures
Via
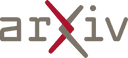
Mar 31, 2025
Abstract:In recent years, Large Language Models (LLMs) have enabled users to provide highly specific music recommendation requests using natural language prompts (e.g. "Can you recommend some old classics for slow dancing?"). In this setup, the recommended tracks are predicted by the LLM in an autoregressive way, i.e. the LLM generates the track titles one token at a time. While intuitive, this approach has several limitation. First, it is based on a general purpose tokenization that is optimized for words rather than for track titles. Second, it necessitates an additional entity resolution layer that matches the track title to the actual track identifier. Third, the number of decoding steps scales linearly with the length of the track title, slowing down inference. In this paper, we propose to address the task of prompt-based music recommendation as a generative retrieval task. Within this setting, we introduce novel, effective, and efficient representations of track identifiers that significantly outperform commonly used strategies. We introduce Text2Tracks, a generative retrieval model that learns a mapping from a user's music recommendation prompt to the relevant track IDs directly. Through an offline evaluation on a dataset of playlists with language inputs, we find that (1) the strategy to create IDs for music tracks is the most important factor for the effectiveness of Text2Tracks and semantic IDs significantly outperform commonly used strategies that rely on song titles as identifiers (2) provided with the right choice of track identifiers, Text2Tracks outperforms sparse and dense retrieval solutions trained to retrieve tracks from language prompts.
Via
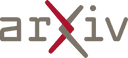
Mar 24, 2025
Abstract:Generative AI is radically changing the creative arts, by fundamentally transforming the way we create and interact with cultural artefacts. While offering unprecedented opportunities for artistic expression and commercialisation, this technology also raises ethical, societal, and legal concerns. Key among these are the potential displacement of human creativity, copyright infringement stemming from vast training datasets, and the lack of transparency, explainability, and fairness mechanisms. As generative systems become pervasive in this domain, responsible design is crucial. Whilst previous work has tackled isolated aspects of generative systems (e.g., transparency, evaluation, data), we take a comprehensive approach, grounding these efforts within the Ethics Guidelines for Trustworthy Artificial Intelligence produced by the High-Level Expert Group on AI appointed by the European Commission - a framework for designing responsible AI systems across seven macro requirements. Focusing on generative music AI, we illustrate how these requirements can be contextualised for the field, addressing trustworthiness across multiple dimensions and integrating insights from the existing literature. We further propose a roadmap for operationalising these contextualised requirements, emphasising interdisciplinary collaboration and stakeholder engagement. Our work provides a foundation for designing and evaluating responsible music generation systems, calling for collaboration among AI experts, ethicists, legal scholars, and artists. This manuscript is accompanied by a website: https://amresearchlab.github.io/raim-framework/.
Via
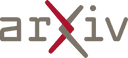