What is facial recognition? Facial recognition is an AI-based technique for identifying or confirming an individual's identity using their face. It maps facial features from an image or video and then compares the information with a collection of known faces to find a match.
Papers and Code
May 14, 2025
Abstract:Face anti-spoofing (FAS) is crucial for protecting facial recognition systems from presentation attacks. Previous methods approached this task as a classification problem, lacking interpretability and reasoning behind the predicted results. Recently, multimodal large language models (MLLMs) have shown strong capabilities in perception, reasoning, and decision-making in visual tasks. However, there is currently no universal and comprehensive MLLM and dataset specifically designed for FAS task. To address this gap, we propose FaceShield, a MLLM for FAS, along with the corresponding pre-training and supervised fine-tuning (SFT) datasets, FaceShield-pre10K and FaceShield-sft45K. FaceShield is capable of determining the authenticity of faces, identifying types of spoofing attacks, providing reasoning for its judgments, and detecting attack areas. Specifically, we employ spoof-aware vision perception (SAVP) that incorporates both the original image and auxiliary information based on prior knowledge. We then use an prompt-guided vision token masking (PVTM) strategy to random mask vision tokens, thereby improving the model's generalization ability. We conducted extensive experiments on three benchmark datasets, demonstrating that FaceShield significantly outperforms previous deep learning models and general MLLMs on four FAS tasks, i.e., coarse-grained classification, fine-grained classification, reasoning, and attack localization. Our instruction datasets, protocols, and codes will be released soon.
Via
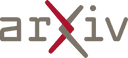
May 25, 2025
Abstract:Recognizing complex emotions linked to ambivalence and hesitancy (A/H) can play a critical role in the personalization and effectiveness of digital behaviour change interventions. These subtle and conflicting emotions are manifested by a discord between multiple modalities, such as facial and vocal expressions, and body language. Although experts can be trained to identify A/H, integrating them into digital interventions is costly and less effective. Automatic learning systems provide a cost-effective alternative that can adapt to individual users, and operate seamlessly within real-time, and resource-limited environments. However, there are currently no datasets available for the design of ML models to recognize A/H. This paper introduces a first Behavioural Ambivalence/Hesitancy (BAH) dataset collected for subject-based multimodal recognition of A/H in videos. It contains videos from 224 participants captured across 9 provinces in Canada, with different age, and ethnicity. Through our web platform, we recruited participants to answer 7 questions, some of which were designed to elicit A/H while recording themselves via webcam with microphone. BAH amounts to 1,118 videos for a total duration of 8.26 hours with 1.5 hours of A/H. Our behavioural team annotated timestamp segments to indicate where A/H occurs, and provide frame- and video-level annotations with the A/H cues. Video transcripts and their timestamps are also included, along with cropped and aligned faces in each frame, and a variety of participants meta-data. We include results baselines for BAH at frame- and video-level recognition in multi-modal setups, in addition to zero-shot prediction, and for personalization using unsupervised domain adaptation. The limited performance of baseline models highlights the challenges of recognizing A/H in real-world videos. The data, code, and pretrained weights are available.
* 41 pages, 13 figures, under review
Via
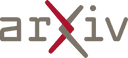
May 14, 2025
Abstract:Face Anti-Spoofing (FAS) is essential for the security of facial recognition systems in diverse scenarios such as payment processing and surveillance. Current multimodal FAS methods often struggle with effective generalization, mainly due to modality-specific biases and domain shifts. To address these challenges, we introduce the \textbf{M}ulti\textbf{m}odal \textbf{D}enoising and \textbf{A}lignment (\textbf{MMDA}) framework. By leveraging the zero-shot generalization capability of CLIP, the MMDA framework effectively suppresses noise in multimodal data through denoising and alignment mechanisms, thereby significantly enhancing the generalization performance of cross-modal alignment. The \textbf{M}odality-\textbf{D}omain Joint \textbf{D}ifferential \textbf{A}ttention (\textbf{MD2A}) module in MMDA concurrently mitigates the impacts of domain and modality noise by refining the attention mechanism based on extracted common noise features. Furthermore, the \textbf{R}epresentation \textbf{S}pace \textbf{S}oft (\textbf{RS2}) Alignment strategy utilizes the pre-trained CLIP model to align multi-domain multimodal data into a generalized representation space in a flexible manner, preserving intricate representations and enhancing the model's adaptability to various unseen conditions. We also design a \textbf{U}-shaped \textbf{D}ual \textbf{S}pace \textbf{A}daptation (\textbf{U-DSA}) module to enhance the adaptability of representations while maintaining generalization performance. These improvements not only enhance the framework's generalization capabilities but also boost its ability to represent complex representations. Our experimental results on four benchmark datasets under different evaluation protocols demonstrate that the MMDA framework outperforms existing state-of-the-art methods in terms of cross-domain generalization and multimodal detection accuracy. The code will be released soon.
Via
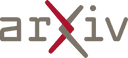
May 26, 2025
Abstract:In this work, we reveal the limitations of visual tokenizers and VAEs in preserving fine-grained features, and propose a benchmark to evaluate reconstruction performance for two challenging visual contents: text and face. Visual tokenizers and VAEs have significantly advanced visual generation and multimodal modeling by providing more efficient compressed or quantized image representations. However, while helping production models reduce computational burdens, the information loss from image compression fundamentally limits the upper bound of visual generation quality. To evaluate this upper bound, we focus on assessing reconstructed text and facial features since they typically: 1) exist at smaller scales, 2) contain dense and rich textures, 3) are prone to collapse, and 4) are highly sensitive to human vision. We first collect and curate a diverse set of clear text and face images from existing datasets. Unlike approaches using VLM models, we employ established OCR and face recognition models for evaluation, ensuring accuracy while maintaining an exceptionally lightweight assessment process <span style="font-weight: bold; color: rgb(214, 21, 21);">requiring just 2GB memory and 4 minutes</span> to complete. Using our benchmark, we analyze text and face reconstruction quality across various scales for different image tokenizers and VAEs. Our results show modern visual tokenizers still struggle to preserve fine-grained features, especially at smaller scales. We further extend this evaluation framework to video, conducting comprehensive analysis of video tokenizers. Additionally, we demonstrate that traditional metrics fail to accurately reflect reconstruction performance for faces and text, while our proposed metrics serve as an effective complement.
Via
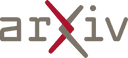
May 09, 2025
Abstract:The emergence of ConvNeXt and its variants has reaffirmed the conceptual and structural suitability of CNN-based models for vision tasks, re-establishing them as key players in image classification in general, and in facial expression recognition (FER) in particular. In this paper, we propose a new set of models that build on these advancements by incorporating a new set of attention mechanisms that combines Triplet attention with Squeeze-and-Excitation (TripSE) in four different variants. We demonstrate the effectiveness of these variants by applying them to the ResNet18, DenseNet and ConvNext architectures to validate their versatility and impact. Our study shows that incorporating a TripSE block in these CNN models boosts their performances, particularly for the ConvNeXt architecture, indicating its utility. We evaluate the proposed mechanisms and associated models across four datasets, namely CIFAR100, ImageNet, FER2013 and AffectNet datasets, where ConvNext with TripSE achieves state-of-the-art results with an accuracy of \textbf{78.27\%} on the popular FER2013 dataset, a new feat for this dataset.
Via
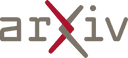
Apr 30, 2025
Abstract:Facial expression recognition (FER) is a subset of computer vision with important applications for human-computer-interaction, healthcare, and customer service. FER represents a challenging problem-space because accurate classification requires a model to differentiate between subtle changes in facial features. In this paper, we examine the use of multi-modal transfer learning to improve performance on a challenging video-based FER dataset, Dynamic Facial Expression in-the-Wild (DFEW). Using a combination of pretrained ResNets, OpenPose, and OmniVec networks, we explore the impact of cross-temporal, multi-modal features on classification accuracy. Ultimately, we find that these finely-tuned multi-modal feature generators modestly improve accuracy of our transformer-based classification model.
* 8 pages, 6 figures
Via
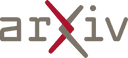
Apr 30, 2025
Abstract:Facial expression recognition (FER) is a key research area in computer vision and human-computer interaction. Despite recent advances in deep learning, challenges persist, especially in generalizing to new scenarios. In fact, zero-shot FER significantly reduces the performance of state-of-the-art FER models. To address this problem, the community has recently started to explore the integration of knowledge from Large Language Models for visual tasks. In this work, we evaluate a broad collection of locally executed Visual Language Models (VLMs), avoiding the lack of task-specific knowledge by adopting a Visual Question Answering strategy. We compare the proposed pipeline with state-of-the-art FER models, both integrating and excluding VLMs, evaluating well-known FER benchmarks: AffectNet, FERPlus, and RAF-DB. The results show excellent performance for some VLMs in zero-shot FER scenarios, indicating the need for further exploration to improve FER generalization.
Via
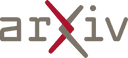
Apr 27, 2025
Abstract:The urging societal demand for fair AI systems has put pressure on the research community to develop predictive models that are not only globally accurate but also meet new fairness criteria, reflecting the lack of disparate mistreatment with respect to sensitive attributes ($\textit{e.g.}$ gender, ethnicity, age). In particular, the variability of the errors made by certain Facial Recognition (FR) systems across specific segments of the population compromises the deployment of the latter, and was judged unacceptable by regulatory authorities. Designing fair FR systems is a very challenging problem, mainly due to the complex and functional nature of the performance measure used in this domain ($\textit{i.e.}$ ROC curves) and because of the huge heterogeneity of the face image datasets usually available for training. In this paper, we propose a novel post-processing approach to improve the fairness of pre-trained FR models by optimizing a regression loss which acts on centroid-based scores. Beyond the computational advantages of the method, we present numerical experiments providing strong empirical evidence of the gain in fairness and of the ability to preserve global accuracy.
* Accepted at both the AFME and RegML Workshops at NeurIPS 2024. A
preliminary version has been accepted for publication by Springer Nature, in
the context of the ICPR 2024 conference
Via
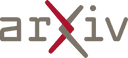
May 08, 2025
Abstract:Recognising expressive behaviours in face videos is a long-standing challenge in Affective Computing. Despite significant advancements in recent years, it still remains a challenge to build a robust and reliable system for naturalistic and in-the-wild facial expressive behaviour analysis in real time. This paper addresses two key challenges in building such a system: (1). The paucity of large-scale labelled facial affect video datasets with extensive coverage of the 2D emotion space, and (2). The difficulty of extracting facial video features that are discriminative, interpretable, robust, and computationally efficient. Toward addressing these challenges, we introduce xTrace, a robust tool for facial expressive behaviour analysis and predicting continuous values of dimensional emotions, namely valence and arousal, from in-the-wild face videos. To address challenge (1), our affect recognition model is trained on the largest facial affect video data set, containing ~450k videos that cover most emotion zones in the dimensional emotion space, making xTrace highly versatile in analysing a wide spectrum of naturalistic expressive behaviours. To address challenge (2), xTrace uses facial affect descriptors that are not only explainable, but can also achieve a high degree of accuracy and robustness with low computational complexity. The key components of xTrace are benchmarked against three existing tools: MediaPipe, OpenFace, and Augsburg Affect Toolbox. On an in-the-wild validation set composed of 50k videos, xTrace achieves 0.86 mean CCC and 0.13 mean absolute error values. We present a detailed error analysis of affect predictions from xTrace, illustrating (a). its ability to recognise emotions with high accuracy across most bins in the 2D emotion space, (b). its robustness to non-frontal head pose angles, and (c). a strong correlation between its uncertainty estimates and its accuracy.
Via
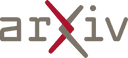
May 01, 2025
Abstract:Cross-spectral face recognition systems are designed to enhance the performance of facial recognition systems by enabling cross-modal matching under challenging operational conditions. A particularly relevant application is the matching of near-infrared (NIR) images to visible-spectrum (VIS) images, enabling the verification of individuals by comparing NIR facial captures acquired with VIS reference images. The use of NIR imaging offers several advantages, including greater robustness to illumination variations, better visibility through glasses and glare, and greater resistance to presentation attacks. Despite these claimed benefits, the robustness of NIR-based systems against presentation attacks has not been systematically studied in the literature. In this work, we conduct a comprehensive evaluation into the vulnerability of NIR-VIS cross-spectral face recognition systems to presentation attacks. Our empirical findings indicate that, although these systems exhibit a certain degree of reliability, they remain vulnerable to specific attacks, emphasizing the need for further research in this area.
* 10 pages
Via
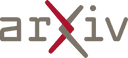