What is facial recognition? Facial recognition is an AI-based technique for identifying or confirming an individual's identity using their face. It maps facial features from an image or video and then compares the information with a collection of known faces to find a match.
Papers and Code
Oct 16, 2024
Abstract:How is identity constructed and performed in the digital via face-based artificial intelligence technologies? While questions of identity on the textual Internet have been thoroughly explored, the Internet has progressed to a multimedia form that not only centers the visual, but specifically the face. At the same time, a wealth of scholarship has and continues to center the topics of surveillance and control through facial recognition technologies (FRTs), which have extended the logics of the racist pseudoscience of physiognomy. Much less work has been devoted to understanding how such face-based artificial intelligence technologies have influenced the formation and performance of identity. This literature review considers how such technologies interact with faciality, which entails the construction of what a face may represent or signify, along axes of identity such as race, gender, and sexuality. In grappling with recent advances in AI such as image generation and deepfakes, I propose that we are now in an era of "post-facial" technologies that build off our existing culture of facility while eschewing the analog face, complicating our relationship with identity vis-a-vis the face. Drawing from previous frameworks of identity play in the digital, as well as trans practices that have historically played with or transgressed the boundaries of identity classification, we can develop concepts adequate for analyzing digital faciality and identity given the current landscape of post-facial artificial intelligence technologies that allow users to interface with the digital in an entirely novel manner. To ground this framework of transgression, I conclude by proposing an interview study with VTubers -- online streamers who perform using motion-captured avatars instead of their real-life faces -- to gain qualitative insight on how these sociotechnical experiences.
Via
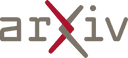
Oct 29, 2024
Abstract:Facial expressions are crucial to human communication, offering insights into emotional states. This study examines how specific facial features influence emotion classification, using facial perturbations on the Fer2013 dataset. As expected, models trained on data with the removal of some important facial feature experienced up to an 85% accuracy drop when compared to baseline for emotions like happy and surprise. Surprisingly, for the emotion disgust, there seem to be slight improvement in accuracy for classifier after mask have been applied. Building on top of this observation, we applied a training scheme to mask out facial features during training, motivating our proposed Perturb Scheme. This scheme, with three phases-attention-based classification, pixel clustering, and feature-focused training, demonstrates improvements in classification accuracy. The experimental results obtained suggests there are some benefits to removing individual facial features in emotion recognition tasks.
* 10 pages. 5 figures
Via
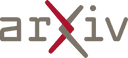
Oct 07, 2024
Abstract:Student expression recognition has become an essential tool for assessing learning experiences and emotional states. This paper introduces xLSTM-FER, a novel architecture derived from the Extended Long Short-Term Memory (xLSTM), designed to enhance the accuracy and efficiency of expression recognition through advanced sequence processing capabilities for student facial expression recognition. xLSTM-FER processes input images by segmenting them into a series of patches and leveraging a stack of xLSTM blocks to handle these patches. xLSTM-FER can capture subtle changes in real-world students' facial expressions and improve recognition accuracy by learning spatial-temporal relationships within the sequence. Experiments on CK+, RAF-DF, and FERplus demonstrate the potential of xLSTM-FER in expression recognition tasks, showing better performance compared to state-of-the-art methods on standard datasets. The linear computational and memory complexity of xLSTM-FER make it particularly suitable for handling high-resolution images. Moreover, the design of xLSTM-FER allows for efficient processing of non-sequential inputs such as images without additional computation.
* The paper, consisting of 10 pages and 3 figures, has been accepted by
the AIEDM Workshop at the 8th APWeb-WAIM Joint International Conference on
Web and Big Data
Via
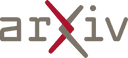
Nov 10, 2024
Abstract:In an era where user interaction with technology is ubiquitous, the importance of user interface (UI) design cannot be overstated. A well-designed UI not only enhances usability but also fosters more natural, intuitive, and emotionally engaging experiences, making technology more accessible and impactful in everyday life. This research addresses this growing need by introducing an advanced emotion recognition system to significantly improve the emotional responsiveness of UI. By integrating facial expressions, speech, and textual data through a multi-branch Transformer model, the system interprets complex emotional cues in real-time, enabling UIs to interact more empathetically and effectively with users. Using the public MELD dataset for validation, our model demonstrates substantial improvements in emotion recognition accuracy and F1 scores, outperforming traditional methods. These findings underscore the critical role that sophisticated emotion recognition plays in the evolution of UIs, making technology more attuned to user needs and emotions. This study highlights how enhanced emotional intelligence in UIs is not only about technical innovation but also about fostering deeper, more meaningful connections between users and the digital world, ultimately shaping how people interact with technology in their daily lives.
Via
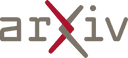
Sep 28, 2024
Abstract:Advanced facial recognition technologies and recommender systems with inadequate privacy technologies and policies for facial interactions increase concerns about bioprivacy violations. With the proliferation of video and live-streaming websites, public-face video distribution and interactions pose greater privacy risks. Existing techniques typically address the risk of sensitive biometric information leakage through various privacy enhancement methods but pose a higher security risk by corrupting the information to be conveyed by the interaction data, or by leaving certain biometric features intact that allow an attacker to infer sensitive biometric information from them. To address these shortcomings, in this paper, we propose a neural network framework, CausalVE. We obtain cover images by adopting a diffusion model to achieve face swapping with face guidance and use the speech sequence features and spatiotemporal sequence features of the secret video for dynamic video inference and prediction to obtain a cover video with the same number of frames as the secret video. In addition, we hide the secret video by using reversible neural networks for video hiding so that the video can also disseminate secret data. Numerous experiments prove that our CausalVE has good security in public video dissemination and outperforms state-of-the-art methods from a qualitative, quantitative, and visual point of view.
* Submitted to ICLR 2025
Via
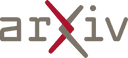
Oct 07, 2024
Abstract:Major Depressive Disorder and anxiety disorders affect millions globally, contributing significantly to the burden of mental health issues. Early screening is crucial for effective intervention, as timely identification of mental health issues can significantly improve treatment outcomes. Artificial intelligence (AI) can be valuable for improving the screening of mental disorders, enabling early intervention and better treatment outcomes. AI-driven screening can leverage the analysis of multiple data sources, including facial features in digital images. However, existing methods often rely on controlled environments or specialized equipment, limiting their broad applicability. This study explores the potential of AI models for ubiquitous depression-anxiety screening given face-centric selfies. The investigation focuses on high-risk pregnant patients, a population that is particularly vulnerable to mental health issues. To cope with limited training data resulting from our clinical setup, pre-trained models were utilized in two different approaches: fine-tuning convolutional neural networks (CNNs) originally designed for facial expression recognition and employing vision-language models (VLMs) for zero-shot analysis of facial expressions. Experimental results indicate that the proposed VLM-based method significantly outperforms CNNs, achieving an accuracy of 77.6% and an F1-score of 56.0%. Although there is significant room for improvement, the results suggest that VLMs can be a promising approach for mental health screening, especially in scenarios with limited data.
Via
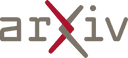
Nov 12, 2024
Abstract:This paper proposes a process for a classification model for the facial expressions. The proposed process would aid in specific categorisation of children's emotions from 2 emotions namely 'Happy' and 'Sad'. Since the existing emotion recognition systems algorithms primarily train on adult faces, the model developed is achieved by using advanced concepts of models with Squeeze-andExcitation blocks, Convolutional Block Attention modules, and robust data augmentation. Stable Diffusion image synthesis was used for expanding and diversifying the data set generating realistic and various training samples. The model designed using Batch Normalisation, Dropout, and SE Attention mechanisms for the classification of children's emotions achieved an accuracy rate of 89\% due to these methods improving the precision of emotion recognition in children. The relative importance of this issue is raised in this study with an emphasis on the call for a more specific model in emotion detection systems for the young generation with specific direction on how the young people can be assisted to manage emotions while online.
Via
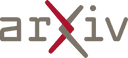
Oct 29, 2024
Abstract:Face verification is a well-known image analysis application and is widely used to recognize individuals in contemporary society. However, most real-world recognition systems ignore the importance of protecting the identity-sensitive facial images that are used for verification. To address this problem, we investigate how to implement a secure face verification system that protects the facial images from being imitated. In our work, we use the DeepID2 convolutional neural network to extract the features of a facial image and an EM algorithm to solve the facial verification problem. To maintain the privacy of facial images, we apply homomorphic encryption schemes to encrypt the facial data and compute the EM algorithm in the ciphertext domain. We develop three face verification systems for surveillance (or entrance) control of a local community based on three levels of privacy concerns. The associated timing performances are presented to demonstrate their feasibility for practical implementation.
* accepted by International Conference on Digital Image and Signal
Processing (DISP) 2019
Via
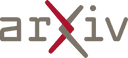
Nov 07, 2024
Abstract:Sign languages are the language of hearing-impaired people who use visuals like the hand, facial, and body movements for communication. There are different signs and gestures representing alphabets, words, and phrases. Nowadays approximately 300 sign languages are being practiced worldwide such as American Sign Language (ASL), Chinese Sign Language (CSL), Indian Sign Language (ISL), and many more. Sign languages are dependent on the vocal language of a place. Unlike vocal or spoken languages, there are no helping words in sign language like is, am, are, was, were, will, be, etc. As only a limited population is well-versed in sign language, this lack of familiarity of sign language hinders hearing-impaired people from communicating freely and easily with everyone. This issue can be addressed by a sign language recognition (SLR) system which has the capability to translate the sign language into vocal language. In this paper, a continuous SLR system is proposed using a deep learning model employing Long Short-Term Memory (LSTM), trained and tested on an ISL primary dataset. This dataset is created using MediaPipe Holistic pipeline for tracking face, hand, and body movements and collecting landmarks. The system recognizes the signs and gestures in real-time with 88.23% accuracy.
* Wireless Personal Communication, 2024
* 14 pages, 4 figures, Wireless Pers Commun
Via
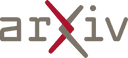
Sep 30, 2024
Abstract:We introduce Facial Expression Category Discovery (FECD), a novel task in the domain of open-world facial expression recognition (O-FER). While Generalized Category Discovery (GCD) has been explored in natural image datasets, applying it to facial expressions presents unique challenges. Specifically, we identify two key biases to better understand these challenges: Theoretical Bias-arising from the introduction of new categories in unlabeled training data, and Practical Bias-stemming from the imbalanced and fine-grained nature of facial expression data. To address these challenges, we propose FER-GCD, an adversarial approach that integrates both implicit and explicit debiasing components. In the implicit debiasing process, we devise F-discrepancy, a novel metric used to estimate the upper bound of Theoretical Bias, helping the model minimize this upper bound through adversarial training. The explicit debiasing process further optimizes the feature generator and classifier to reduce Practical Bias. Extensive experiments on GCD-based FER datasets demonstrate that our FER-GCD framework significantly improves accuracy on both old and new categories, achieving an average improvement of 9.8% over the baseline and outperforming state-of-the-art methods.
Via
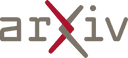