Jan 13, 2025
Abstract:It is now common to evaluate Large Language Models (LLMs) by having humans manually vote to evaluate model outputs, in contrast to typical benchmarks that evaluate knowledge or skill at some particular task. Chatbot Arena, the most popular benchmark of this type, ranks models by asking users to select the better response between two randomly selected models (without revealing which model was responsible for the generations). These platforms are widely trusted as a fair and accurate measure of LLM capabilities. In this paper, we show that if bot protection and other defenses are not implemented, these voting-based benchmarks are potentially vulnerable to adversarial manipulation. Specifically, we show that an attacker can alter the leaderboard (to promote their favorite model or demote competitors) at the cost of roughly a thousand votes (verified in a simulated, offline version of Chatbot Arena). Our attack consists of two steps: first, we show how an attacker can determine which model was used to generate a given reply with more than $95\%$ accuracy; and then, the attacker can use this information to consistently vote for (or against) a target model. Working with the Chatbot Arena developers, we identify, propose, and implement mitigations to improve the robustness of Chatbot Arena against adversarial manipulation, which, based on our analysis, substantially increases the cost of such attacks. Some of these defenses were present before our collaboration, such as bot protection with Cloudflare, malicious user detection, and rate limiting. Others, including reCAPTCHA and login are being integrated to strengthen the security in Chatbot Arena.
Via
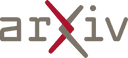