What is autonomous cars? Autonomous cars are self-driving vehicles that use artificial intelligence (AI) and sensors to navigate and operate without human intervention, using high-resolution cameras and lidars that detect what happens in the car's immediate surroundings. They have the potential to revolutionize transportation by improving safety, efficiency, and accessibility.
Papers and Code
Jun 19, 2024
Abstract:This paper presents the design of an autonomous race car that is self-designed, self-developed, and self-built by the Elefant Racing team at the University of Bayreuth. The system is created to compete in the Formula Student Driverless competition. Its primary focus is on the Acceleration track, a straight 75-meter-long course, and the Skidpad track, which comprises two circles forming an eight. Additionally, it is experimentally capable of competing in the Autocross and Trackdrive events, which feature tracks with previously unknown straights and curves. The paper details the hardware, software and sensor setup employed during the 2020/2021 season. Despite being developed by a small team with limited computer science expertise, the design won the Formula Student East Engineering Design award. Emphasizing simplicity and efficiency, the team employed streamlined techniques to achieve their success.
Via
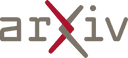
Oct 08, 2024
Abstract:Motion forecasting for agents in autonomous driving is highly challenging due to the numerous possibilities for each agent's next action and their complex interactions in space and time. In real applications, motion forecasting takes place repeatedly and continuously as the self-driving car moves. However, existing forecasting methods typically process each driving scene within a certain range independently, totally ignoring the situational and contextual relationships between successive driving scenes. This significantly simplifies the forecasting task, making the solutions suboptimal and inefficient to use in practice. To address this fundamental limitation, we propose a novel motion forecasting framework for continuous driving, named RealMotion. It comprises two integral streams both at the scene level: (1) The scene context stream progressively accumulates historical scene information until the present moment, capturing temporal interactive relationships among scene elements. (2) The agent trajectory stream optimizes current forecasting by sequentially relaying past predictions. Besides, a data reorganization strategy is introduced to narrow the gap between existing benchmarks and real-world applications, consistent with our network. These approaches enable exploiting more broadly the situational and progressive insights of dynamic motion across space and time. Extensive experiments on Argoverse series with different settings demonstrate that our RealMotion achieves state-of-the-art performance, along with the advantage of efficient real-world inference. The source code will be available at https://github.com/fudan-zvg/RealMotion.
* Accepted at NeurIPS 2024 Spotlight
Via
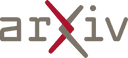
Oct 11, 2024
Abstract:In the evolving landscape of autonomous vehicles, ensuring robust in-vehicle network (IVN) security is paramount. This paper introduces an advanced intrusion detection system (IDS) called KD-XVAE that uses a Variational Autoencoder (VAE)-based knowledge distillation approach to enhance both performance and efficiency. Our model significantly reduces complexity, operating with just 1669 parameters and achieving an inference time of 0.3 ms per batch, making it highly suitable for resource-constrained automotive environments. Evaluations in the HCRL Car-Hacking dataset demonstrate exceptional capabilities, attaining perfect scores (Recall, Precision, F1 Score of 100%, and FNR of 0%) under multiple attack types, including DoS, Fuzzing, Gear Spoofing, and RPM Spoofing. Comparative analysis on the CICIoV2024 dataset further underscores its superiority over traditional machine learning models, achieving perfect detection metrics. We furthermore integrate Explainable AI (XAI) techniques to ensure transparency in the model's decisions. The VAE compresses the original feature space into a latent space, on which the distilled model is trained. SHAP(SHapley Additive exPlanations) values provide insights into the importance of each latent dimension, mapped back to original features for intuitive understanding. Our paper advances the field by integrating state-of-the-art techniques, addressing critical challenges in the deployment of efficient, trustworthy, and reliable IDSes for autonomous vehicles, ensuring enhanced protection against emerging cyber threats.
* In Proceedings of the Sixth Workshop on CPSIoT Security and Privacy
(CPSIoTSec 24), October 14-18, 2024, Salt Lake City, UT, USA. ACM, New York,
NY, USA
Via
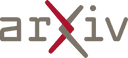
Oct 15, 2024
Abstract:The hype around self-driving cars has been growing over the past years and has sparked much research. Several modules in self-driving cars are thoroughly investigated to ensure safety, comfort, and efficiency, among which the controller is crucial. The controller module can be categorized into longitudinal and lateral controllers in which the task of the former is to follow the reference velocity, and the latter is to reduce the lateral displacement error from the reference path. Generally, a tuned controller is not sufficient to perform in all environments. Thus, a controller that can adapt to changing conditions is necessary for autonomous driving. Furthermore, these controllers often depend on vehicle models that also need to adapt over time due to varying environments. This paper uses graphs to present novel techniques to learn the vehicle model and the lateral controller online. First, a heterogeneous graph is presented depicting the current states of and inputs to the vehicle. The vehicle model is then learned online using known physical constraints in conjunction with the processing of the graph through a Graph Neural Network structure. Next, another heterogeneous graph - depicting the transition from current to desired states - is processed through another Graph Neural Network structure to generate the steering command on the fly. Finally, the performance of this self-learning model-based lateral controller is evaluated and shown to be satisfactory on an open-source autonomous driving platform called CARLA.
* The article has been published in the early access area on IEEE
Xplore for the IEEE Transactions on Intelligent Vehicles (2024). This is the
accepted version. Number of pages: 12 pages, Number of figures: 10
Via
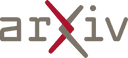
Oct 18, 2024
Abstract:In this work, we consider the problem of learning end to end perception to control for ground vehicles solely from aerial imagery. Photogrammetric simulators allow the synthesis of novel views through the transformation of pre-generated assets into novel views.However, they have a large setup cost, require careful collection of data and often human effort to create usable simulators. We use a Neural Radiance Field (NeRF) as an intermediate representation to synthesize novel views from the point of view of a ground vehicle. These novel viewpoints can then be used for several downstream autonomous navigation applications. In this work, we demonstrate the utility of novel view synthesis though the application of training a policy for end to end learning from images and depth data. In a traditional real to sim to real framework, the collected data would be transformed into a visual simulator which could then be used to generate novel views. In contrast, using a NeRF allows a compact representation and the ability to optimize over the parameters of the visual simulator as more data is gathered in the environment. We demonstrate the efficacy of our method in a custom built mini-city environment through the deployment of imitation policies on robotic cars. We additionally consider the task of place localization and demonstrate that our method is able to relocalize the car in the real world.
* Presented at IROS 2024
Via
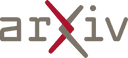
Aug 02, 2024
Abstract:Point cloud segmentation (PCS) aims to make per-point predictions and enables robots and autonomous driving cars to understand the environment. The range image is a dense representation of a large-scale outdoor point cloud, and segmentation models built upon the image commonly execute efficiently. However, the projection of the point cloud onto the range image inevitably leads to dropping points because, at each image coordinate, only one point is kept despite multiple points being projected onto the same location. More importantly, it is challenging to assign correct predictions to the dropped points that belong to the classes different from the kept point class. Besides, existing post-processing methods, such as K-nearest neighbor (KNN) search and kernel point convolution (KPConv), cannot be trained with the models in an end-to-end manner or cannot process varying-density outdoor point clouds well, thereby enabling the models to achieve sub-optimal performance. To alleviate this problem, we propose a trainable pointwise decoder module (PDM) as the post-processing approach, which gathers weighted features from the neighbors and then makes the final prediction for the query point. In addition, we introduce a virtual range image-guided copy-rotate-paste (VRCrop) strategy in data augmentation. VRCrop constrains the total number of points and eliminates undesirable artifacts in the augmented point cloud. With PDM and VRCrop, existing range image-based segmentation models consistently perform better than their counterparts on the SemanticKITTI, SemanticPOSS, and nuScenes datasets.
* No comments
Via
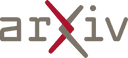
Jun 23, 2024
Abstract:Car-following (CF) modeling, a fundamental component in microscopic traffic simulation, has attracted increasing interest of researchers in the past decades. In this study, we propose an adaptable personalized car-following framework -MetaFollower, by leveraging the power of meta-learning. Specifically, we first utilize Model-Agnostic Meta-Learning (MAML) to extract common driving knowledge from various CF events. Afterward, the pre-trained model can be fine-tuned on new drivers with only a few CF trajectories to achieve personalized CF adaptation. We additionally combine Long Short-Term Memory (LSTM) and Intelligent Driver Model (IDM) to reflect temporal heterogeneity with high interpretability. Unlike conventional adaptive cruise control (ACC) systems that rely on predefined settings and constant parameters without considering heterogeneous driving characteristics, MetaFollower can accurately capture and simulate the intricate dynamics of car-following behavior while considering the unique driving styles of individual drivers. We demonstrate the versatility and adaptability of MetaFollower by showcasing its ability to adapt to new drivers with limited training data quickly. To evaluate the performance of MetaFollower, we conduct rigorous experiments comparing it with both data-driven and physics-based models. The results reveal that our proposed framework outperforms baseline models in predicting car-following behavior with higher accuracy and safety. To the best of our knowledge, this is the first car-following model aiming to achieve fast adaptation by considering both driver and temporal heterogeneity based on meta-learning.
Via
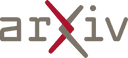
Aug 25, 2024
Abstract:Reinforcement learning is a widely used approach to autonomous navigation, showing potential in various tasks and robotic setups. Still, it often struggles to reach distant goals when safety constraints are imposed (e.g., the wheeled robot is prohibited from moving close to the obstacles). One of the main reasons for poor performance in such setups, which is common in practice, is that the need to respect the safety constraints degrades the exploration capabilities of an RL agent. To this end, we introduce a novel learnable algorithm that is based on decomposing the initial problem into smaller sub-problems via intermediate goals, on the one hand, and respects the limit of the cumulative safety constraints, on the other hand -- SPEIS(Safe Policy Exploration Improvement via Subgoals). It comprises the two coupled policies trained end-to-end: subgoal and safe. The subgoal policy is trained to generate the subgoal based on the transitions from the buffer of the safe (main) policy that helps the safe policy to reach distant goals. Simultaneously, the safe policy maximizes its rewards while attempting not to violate the limit of the cumulative safety constraints, thus providing a certain level of safety. We evaluate SPEIS in a wide range of challenging (simulated) environments that involve different types of robots in two different environments: autonomous vehicles from the POLAMP environment and car, point, doggo, and sweep from the safety-gym environment. We demonstrate that our method consistently outperforms state-of-the-art competitors and can significantly reduce the collision rate while maintaining high success rates (higher by 80% compared to the best-performing methods).
* 11 pages, 8 figures
Via
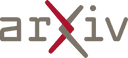
Jul 12, 2024
Abstract:Multi-agent systems (MAS) constitute a significant role in exploring machine intelligence and advanced applications. In order to deeply investigate complicated interactions within MAS scenarios, we originally propose "GNN for MBRL" model, which utilizes a state-spaced Graph Neural Networks with Model-based Reinforcement Learning to address specific MAS missions (e.g., Billiard-Avoidance, Autonomous Driving Cars). In detail, we firstly used GNN model to predict future states and trajectories of multiple agents, then applied the Cross-Entropy Method (CEM) optimized Model Predictive Control to assist the ego-agent planning actions and successfully accomplish certain MAS tasks.
Via
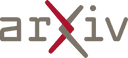
Jul 01, 2024
Abstract:In this technical report, we present our solution for the Vision-Centric 3D Occupancy and Flow Prediction track in the nuScenes Open-Occ Dataset Challenge at CVPR 2024. Our innovative approach involves a dual-stage framework that enhances 3D occupancy and flow predictions by incorporating adaptive forward view transformation and flow modeling. Initially, we independently train the occupancy model, followed by flow prediction using sequential frame integration. Our method combines regression with classification to address scale variations in different scenes, and leverages predicted flow to warp current voxel features to future frames, guided by future frame ground truth. Experimental results on the nuScenes dataset demonstrate significant improvements in accuracy and robustness, showcasing the effectiveness of our approach in real-world scenarios. Our single model based on Swin-Base ranks second on the public leaderboard, validating the potential of our method in advancing autonomous car perception systems.
* 2nd Place in the 3D Occupancy and Flow Prediction Challenge (CVPR24)
Via
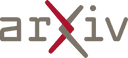