May 19, 2025
Abstract:Providing personalized, detailed feedback at scale in large undergraduate STEM courses remains a persistent challenge. We present an empirically evaluated practice exam system that integrates AI generated feedback with targeted textbook references, deployed in a large introductory biology course. Our system encourages metacognitive behavior by asking students to explain their answers and declare their confidence. It uses OpenAI's GPT-4o to generate personalized feedback based on this information, while directing them to relevant textbook sections. Through interaction logs from consenting participants across three midterms (541, 342, and 413 students respectively), totaling 28,313 question-student interactions across 146 learning objectives, along with 279 surveys and 23 interviews, we examined the system's impact on learning outcomes and engagement. Across all midterms, feedback types showed no statistically significant performance differences, though some trends suggested potential benefits. The most substantial impact came from the required confidence ratings and explanations, which students reported transferring to their actual exam strategies. About 40 percent of students engaged with textbook references when prompted by feedback -- far higher than traditional reading rates. Survey data revealed high satisfaction (mean rating 4.1 of 5), with 82.1 percent reporting increased confidence on practiced midterm topics, and 73.4 percent indicating they could recall and apply specific concepts. Our findings suggest that embedding structured reflection requirements may be more impactful than sophisticated feedback mechanisms.
* 10 pages, 3 figures, to appear in Proceedings of the Twelfth ACM
Conference on Learning @ Scale (L@S 2025), July 2025, Palermo, Italy
Via
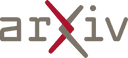