Jul 03, 2025
Abstract:We present an open-source system designed for multilingual translation and speech regeneration, addressing challenges in communication and accessibility across diverse linguistic contexts. The system integrates Whisper for speech recognition with Voice Activity Detection (VAD) to identify speaking intervals, followed by a pipeline of Large Language Models (LLMs). For multilingual applications, the first LLM segments speech into coherent, complete sentences, which a second LLM then translates. For speech regeneration, the system uses a text-to-speech (TTS) module with voice cloning capabilities to replicate the original speaker's voice, maintaining naturalness and speaker identity. The system's open-source components can operate locally or via APIs, offering cost-effective deployment across various use cases. These include real-time multilingual translation in Zoom sessions, speech regeneration for public broadcasts, and Bluetooth-enabled multilingual playback through personal devices. By preserving the speaker's voice, the system ensures a seamless and immersive experience, whether translating or regenerating speech. This open-source project is shared with the community to foster innovation and accessibility. We provide a detailed system performance analysis, including latency and word accuracy, demonstrating its potential to enable inclusive, adaptable communication solutions in real-world multilingual scenarios.
* Presented at Forum Acusticum Euronoise 2025
Via
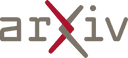