Topic:Text Classification
What is Text Classification? Text classification is the process of categorizing text documents into predefined categories or labels.
Papers and Code
May 24, 2025
Abstract:Machine learning models for text classification are trained to predict a class for a given text. To do this, training and validation samples must be prepared: a set of texts is collected, and each text is assigned a class. These classes are usually assigned by human annotators with different expertise levels, depending on the specific classification task. Collecting such samples from scratch is labor-intensive because it requires finding specialists and compensating them for their work; moreover, the number of available specialists is limited, and their productivity is constrained by human factors. While it may not be too resource-intensive to collect samples once, the ongoing need to retrain models (especially in incremental learning pipelines) to address data drift (also called model drift) makes the data collection process crucial and costly over the model's entire lifecycle. This paper proposes several approaches to replace human annotators with Large Language Models (LLMs) to test classifier predictions for correctness, helping ensure model quality and support high-quality incremental learning.
Via
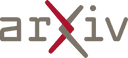
May 28, 2025
Abstract:We revisit the classical, full-fledged Bayesian model averaging (BMA) paradigm to ensemble pre-trained and/or lightly-finetuned foundation models to enhance the classification performance on image and text data. To make BMA tractable under foundation models, we introduce trainable linear classifiers that take frozen features from the pre-trained foundation models as inputs. The model posteriors over the linear classifiers tell us which linear heads and frozen features are better suited for a given dataset, resulting in a principled model ensembling method. Furthermore, we propose a computationally cheaper, optimizable model averaging scheme (OMA). In OMA, we directly optimize the model ensemble weights, just like those weights based on model posterior distributions in BMA, by reducing the amount of surprise (expected entropy of the predictions) we get from predictions of ensembled models. With the rapid development of foundation models, these approaches will enable the incorporation of future, possibly significantly better foundation models to enhance the performance of challenging classification tasks.
Via
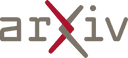
May 23, 2025
Abstract:Despite the widely recognized success of residual connections in modern neural networks, their design principles remain largely heuristic. This paper introduces KITINet (Kinetics Theory Inspired Network), a novel architecture that reinterprets feature propagation through the lens of non-equilibrium particle dynamics and partial differential equation (PDE) simulation. At its core, we propose a residual module that models feature updates as the stochastic evolution of a particle system, numerically simulated via a discretized solver for the Boltzmann transport equation (BTE). This formulation mimics particle collisions and energy exchange, enabling adaptive feature refinement via physics-informed interactions. Additionally, we reveal that this mechanism induces network parameter condensation during training, where parameters progressively concentrate into a sparse subset of dominant channels. Experiments on scientific computation (PDE operator), image classification (CIFAR-10/100), and text classification (IMDb/SNLI) show consistent improvements over classic network baselines, with negligible increase of FLOPs.
Via
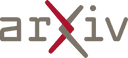
May 20, 2025
Abstract:This study introduces a framework for evaluating consistency in large language model (LLM) binary text classification, addressing the lack of established reliability assessment methods. Adapting psychometric principles, we determine sample size requirements, develop metrics for invalid responses, and evaluate intra- and inter-rater reliability. Our case study examines financial news sentiment classification across 14 LLMs (including claude-3-7-sonnet, gpt-4o, deepseek-r1, gemma3, llama3.2, phi4, and command-r-plus), with five replicates per model on 1,350 articles. Models demonstrated high intra-rater consistency, achieving perfect agreement on 90-98% of examples, with minimal differences between expensive and economical models from the same families. When validated against StockNewsAPI labels, models achieved strong performance (accuracy 0.76-0.88), with smaller models like gemma3:1B, llama3.2:3B, and claude-3-5-haiku outperforming larger counterparts. All models performed at chance when predicting actual market movements, indicating task constraints rather than model limitations. Our framework provides systematic guidance for LLM selection, sample size planning, and reliability assessment, enabling organizations to optimize resources for classification tasks.
* 25 pages
Via
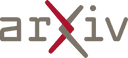
May 22, 2025
Abstract:We address the problem of incremental sequence classification, where predictions are updated as new elements in the sequence are revealed. Drawing on temporal-difference learning from reinforcement learning, we identify a temporal-consistency condition that successive predictions should satisfy. We leverage this condition to develop a novel loss function for training incremental sequence classifiers. Through a concrete example, we demonstrate that optimizing this loss can offer substantial gains in data efficiency. We apply our method to text classification tasks and show that it improves predictive accuracy over competing approaches on several benchmark datasets. We further evaluate our approach on the task of verifying large language model generations for correctness in grade-school math problems. Our results show that models trained with our method are better able to distinguish promising generations from unpromising ones after observing only a few tokens.
Via
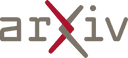
May 26, 2025
Abstract:Aiming at the problems of cross-modal feature fusion, low efficiency of long text modeling and lack of hierarchical semantic coherence in patent text semantic mining, this study proposes HGM-Net, a deep learning framework that integrates Hierarchical Comparative Learning (HCL), Multi-modal Graph Attention Network (M-GAT) and Multi-Granularity Sparse Attention (MSA), which builds a dynamic mask, contrast and cross-structural similarity constraints on the word, sentence and paragraph hierarchies through HCL. Contrast and cross-structural similarity constraints are constructed at the word and paragraph levels by HCL to strengthen the local semantic and global thematic consistency of patent text; M-GAT models patent classification codes, citation relations and text semantics as heterogeneous graph structures, and achieves dynamic fusion of multi-source features by cross-modal gated attention; MSA adopts a hierarchical sparsity strategy to optimize the computational efficiency of long text modeling at word, phrase, sentence and paragraph granularity. Experiments show that the framework demonstrates significant advantages over existing deep learning methods in tasks such as patent classification and similarity matching, and provides a solution with both theoretical innovation and practical value for solving the problems of patent examination efficiency improvement and technology relevance mining.
Via
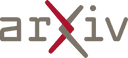
May 29, 2025
Abstract:We present a new adaptation method MaCP, Minimal yet Mighty adaptive Cosine Projection, that achieves exceptional performance while requiring minimal parameters and memory for fine-tuning large foundation models. Its general idea is to exploit the superior energy compaction and decorrelation properties of cosine projection to improve both model efficiency and accuracy. Specifically, it projects the weight change from the low-rank adaptation into the discrete cosine space. Then, the weight change is partitioned over different levels of the discrete cosine spectrum, and each partition's most critical frequency components are selected. Extensive experiments demonstrate the effectiveness of MaCP across a wide range of single-modality tasks, including natural language understanding, natural language generation, text summarization, as well as multi-modality tasks such as image classification and video understanding. MaCP consistently delivers superior accuracy, significantly reduced computational complexity, and lower memory requirements compared to existing alternatives.
* arXiv admin note: substantial text overlap with arXiv:2410.09103
Via
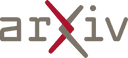
May 26, 2025
Abstract:Multimodal Large Language Models (MLLMs) have demonstrated impressive capabilities across tasks, yet they often exhibit difficulty in distinguishing task-relevant from irrelevant signals, particularly in tasks like Visual Question Answering (VQA), which can lead to susceptibility to misleading or spurious inputs. We refer to this broader limitation as the Cross-Modality Competency Problem: the model's inability to fairly evaluate all modalities. This vulnerability becomes more evident in modality-specific tasks such as image classification or pure text question answering, where models are expected to rely solely on one modality. In such tasks, spurious information from irrelevant modalities often leads to significant performance degradation. We refer to this failure as Modality Interference, which serves as a concrete and measurable instance of the cross-modality competency problem. We further design a perturbation-based causal diagnostic experiment to verify and quantify this problem. To mitigate modality interference, we propose a novel framework to fine-tune MLLMs, including perturbation-based data augmentations with both heuristic perturbations and adversarial perturbations via Projected Gradient Descent (PGD), and a consistency regularization strategy applied to model outputs with original and perturbed inputs. Experiments on multiple benchmark datasets (image-heavy, text-heavy, and VQA tasks) and multiple model families with different scales demonstrate significant improvements in robustness and cross-modality competency, indicating our method's effectiveness in boosting unimodal reasoning ability while enhancing performance on multimodal tasks.
Via
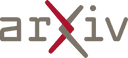
May 30, 2025
Abstract:Large language models and vision-language models (which we jointly call LMs) have transformed NLP and CV, demonstrating remarkable potential across various fields. However, their capabilities in affective analysis (i.e. sentiment analysis and emotion detection) remain underexplored. This gap is largely due to the absence of comprehensive evaluation benchmarks, and the inherent complexity of affective analysis tasks. In this paper, we introduce MMAFFBen, the first extensive open-source benchmark for multilingual multimodal affective analysis. MMAFFBen encompasses text, image, and video modalities across 35 languages, covering four key affective analysis tasks: sentiment polarity, sentiment intensity, emotion classification, and emotion intensity. Moreover, we construct the MMAFFIn dataset for fine-tuning LMs on affective analysis tasks, and further develop MMAFFLM-3b and MMAFFLM-7b based on it. We evaluate various representative LMs, including GPT-4o-mini, providing a systematic comparison of their affective understanding capabilities. This project is available at https://github.com/lzw108/MMAFFBen.
* Work in progress
Via
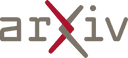
Jun 05, 2025
Abstract:Composed Video Retrieval (CoVR) retrieves a target video given a query video and a modification text describing the intended change. Existing CoVR benchmarks emphasize appearance shifts or coarse event changes and therefore do not test the ability to capture subtle, fast-paced temporal differences. We introduce TF-CoVR, the first large-scale benchmark dedicated to temporally fine-grained CoVR. TF-CoVR focuses on gymnastics and diving and provides 180K triplets drawn from FineGym and FineDiving. Previous CoVR benchmarks focusing on temporal aspect, link each query to a single target segment taken from the same video, limiting practical usefulness. In TF-CoVR, we instead construct each <query, modification> pair by prompting an LLM with the label differences between clips drawn from different videos; every pair is thus associated with multiple valid target videos (3.9 on average), reflecting real-world tasks such as sports-highlight generation. To model these temporal dynamics we propose TF-CoVR-Base, a concise two-stage training framework: (i) pre-train a video encoder on fine-grained action classification to obtain temporally discriminative embeddings; (ii) align the composed query with candidate videos using contrastive learning. We conduct the first comprehensive study of image, video, and general multimodal embedding (GME) models on temporally fine-grained composed retrieval in both zero-shot and fine-tuning regimes. On TF-CoVR, TF-CoVR-Base improves zero-shot mAP@50 from 5.92 (LanguageBind) to 7.51, and after fine-tuning raises the state-of-the-art from 19.83 to 25.82.
Via
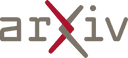