What is Sentiment Analysis? Sentiment analysis is the process of determining the sentiment of a piece of text, such as a tweet or a review.
Papers and Code
Feb 20, 2025
Abstract:In recent years, Large Language Models have garnered significant attention for their strong performance in various natural language tasks, such as machine translation and question answering. These models demonstrate an impressive ability to generalize across diverse tasks. However, their effectiveness in tackling domain-specific tasks, such as financial sentiment analysis and monetary policy understanding, remains a topic of debate, as these tasks often require specialized knowledge and precise reasoning. To address such challenges, researchers design various prompts to unlock the models' abilities. By carefully crafting input prompts, researchers can guide these models to produce more accurate responses. Consequently, prompt engineering has become a key focus of study. Despite the advancements in both models and prompt engineering, the relationship between the two-specifically, how prompt design impacts models' ability to perform domain-specific tasks-remains underexplored. This paper aims to bridge this research gap.
Via
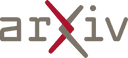
Feb 19, 2025
Abstract:Transformer-based models dominate NLP tasks like sentiment analysis, machine translation, and claim verification. However, their massive computational demands and lack of interpretability pose challenges for real-world applications requiring efficiency and transparency. In this work, we explore Graph Neural Networks (GNNs) and Hyperbolic Graph Neural Networks (HGNNs) as lightweight yet effective alternatives for Environmental Claim Detection, reframing it as a graph classification problem. We construct dependency parsing graphs to explicitly model syntactic structures, using simple word embeddings (word2vec) for node features with dependency relations encoded as edge features. Our results demonstrate that these graph-based models achieve comparable or superior performance to state-of-the-art transformers while using 30x fewer parameters. This efficiency highlights the potential of structured, interpretable, and computationally efficient graph-based approaches.
Via
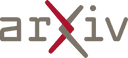
Feb 20, 2025
Abstract:The widespread dissemination of rumors on social media has a significant impact on people's lives, potentially leading to public panic and fear. Rumors often evoke specific sentiments, resonating with readers and prompting sharing. To effectively detect and track rumors, it is essential to observe the fine-grained sentiments of both source and response message pairs as the rumor evolves over time. However, current rumor detection methods fail to account for this aspect. In this paper, we propose MSuf, the first multi-task suffix learning framework for rumor detection and tracking using time series dual (coupled) sentiments. MSuf includes three modules: (1) an LLM to extract sentiment intensity features and sort them chronologically; (2) a module that fuses the sorted sentiment features with their source text word embeddings to obtain an aligned embedding; (3) two hard prompts are combined with the aligned vector to perform rumor detection and sentiment analysis using one frozen LLM. MSuf effectively enhances the performance of LLMs for rumor detection with only minimal parameter fine-tuning. Evaluating MSuf on four rumor detection benchmarks, we find significant improvements compared to other emotion-based methods.
* work in progress
Via
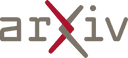
Feb 18, 2025
Abstract:Masked language modeling has become a widely adopted unsupervised technique to pre-train language models. However, the process of selecting tokens for masking is random, and the percentage of masked tokens is typically fixed for the entire training process. In this paper, we propose to adjust the masking ratio and to decide which tokens to mask based on a novel task-informed anti-curriculum learning scheme. First, we harness task-specific knowledge about useful and harmful tokens in order to determine which tokens to mask. Second, we propose a cyclic decaying masking ratio, which corresponds to an anti-curriculum schedule (from hard to easy). We exemplify our novel task-informed anti-curriculum by masking (TIACBM) approach across three diverse downstream tasks: sentiment analysis, text classification by topic, and authorship attribution. Our findings suggest that TIACBM enhances the ability of the model to focus on key task-relevant features, contributing to statistically significant performance gains across tasks. We release our code at https://github.com/JarcaAndrei/TIACBM.
Via
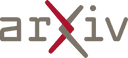
Feb 21, 2025
Abstract:As voice assistants (VAs) become increasingly integrated into daily life, the need for emotion-aware systems that can recognize and respond appropriately to user emotions has grown. While significant progress has been made in speech emotion recognition (SER) and sentiment analysis, effectively addressing user emotions-particularly negative ones-remains a challenge. This study explores human emotional response strategies in VA interactions using a role-swapping approach, where participants regulate AI emotions rather than receiving pre-programmed responses. Through speech feature analysis and natural language processing (NLP), we examined acoustic and linguistic patterns across various emotional scenarios. Results show that participants favor neutral or positive emotional responses when engaging with negative emotional cues, highlighting a natural tendency toward emotional regulation and de-escalation. Key acoustic indicators such as root mean square (RMS), zero-crossing rate (ZCR), and jitter were identified as sensitive to emotional states, while sentiment polarity and lexical diversity (TTR) distinguished between positive and negative responses. These findings provide valuable insights for developing adaptive, context-aware VAs capable of delivering empathetic, culturally sensitive, and user-aligned responses. By understanding how humans naturally regulate emotions in AI interactions, this research contributes to the design of more intuitive and emotionally intelligent voice assistants, enhancing user trust and engagement in human-AI interactions.
* 19 pages, 6 figures
Via
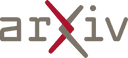
Feb 13, 2025
Abstract:Conversational AI chatbots have become increasingly common within the customer service industry. Despite improvements in their emotional development, they often lack the authenticity of real customer service interactions or the competence of service providers. By comparing emotion-sensitive and emotion-insensitive LLM-based chatbots across 30 participants, we aim to explore how emotional sensitivity in chatbots influences perceived competence and overall customer satisfaction in service interactions. Additionally, we employ sentiment analysis techniques to analyze and interpret the emotional content of user inputs. We highlight that perceptions of chatbot trustworthiness and competence were higher in the case of the emotion-sensitive chatbot, even if issue resolution rates were not affected. We discuss implications of improved user satisfaction from emotion-sensitive chatbots and potential applications in support services.
* 7 pages, 2 figures, 1 table
Via
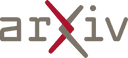
Feb 17, 2025
Abstract:Many existing approaches for learning from labeled data assume the existence of gold-standard labels. According to these approaches, inter-annotator disagreement is seen as noise to be removed, either through refinement of annotation guidelines, label adjudication, or label filtering. However, annotator disagreement can rarely be totally eradicated, especially on more subjective tasks such as sentiment analysis or hate speech detection where disagreement is natural. Therefore, a new approach to learning from labeled data, called data perspectivism, seeks to leverage inter-annotator disagreement to learn models that stay true to the inherent uncertainty of the task by treating annotations as opinions of the annotators, rather than gold-standard facts. Despite this conceptual grounding, existing methods under data perspectivism are limited to using disagreement as the sole source of annotation uncertainty. To expand the possibilities of data perspectivism, we introduce Subjective Logic Encodings (SLEs), a flexible framework for constructing classification targets that explicitly encodes annotations as opinions of the annotators. Based on Subjective Logic Theory, SLEs encode labels as Dirichlet distributions and provide principled methods for encoding and aggregating various types of annotation uncertainty -- annotator confidence, reliability, and disagreement -- into the targets. We show that SLEs are a generalization of other types of label encodings as well as how to estimate models to predict SLEs using a distribution matching objective.
* We make our code publicly available at
https://github.com/jvasilakes/SLEncodings
Via
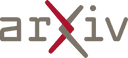
Mar 26, 2025
Abstract:Investigating the public experience of urgent care facilities is essential for promoting community healthcare development. Traditional survey methods often fall short due to limited scope, time, and spatial coverage. Crowdsourcing through online reviews or social media offers a valuable approach to gaining such insights. With recent advancements in large language models (LLMs), extracting nuanced perceptions from reviews has become feasible. This study collects Google Maps reviews across the DMV and Florida areas and conducts prompt engineering with the GPT model to analyze the aspect-based sentiment of urgent care. We first analyze the geospatial patterns of various aspects, including interpersonal factors, operational efficiency, technical quality, finances, and facilities. Next, we determine Census Block Group(CBG)-level characteristics underpinning differences in public perception, including population density, median income, GINI Index, rent-to-income ratio, household below poverty rate, no insurance rate, and unemployment rate. Our results show that interpersonal factors and operational efficiency emerge as the strongest determinants of patient satisfaction in urgent care, while technical quality, finances, and facilities show no significant independent effects when adjusted for in multivariate models. Among socioeconomic and demographic factors, only population density demonstrates a significant but modest association with patient ratings, while the remaining factors exhibit no significant correlations. Overall, this study highlights the potential of crowdsourcing to uncover the key factors that matter to residents and provide valuable insights for stakeholders to improve public satisfaction with urgent care.
Via
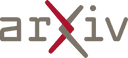
Feb 11, 2025
Abstract:While self-attention has been instrumental in the success of Transformers, it can lead to over-concentration on a few tokens during training, resulting in suboptimal information flow. Enforcing doubly-stochastic constraints in attention matrices has been shown to improve structure and balance in attention distributions. However, existing methods rely on iterative Sinkhorn normalization, which is computationally costly. In this paper, we introduce a novel, fully parallelizable doubly-stochastic attention mechanism based on sliced optimal transport, leveraging Expected Sliced Transport Plans (ESP). Unlike prior approaches, our method enforces double stochasticity without iterative Sinkhorn normalization, significantly enhancing efficiency. To ensure differentiability, we incorporate a temperature-based soft sorting technique, enabling seamless integration into deep learning models. Experiments across multiple benchmark datasets, including image classification, point cloud classification, sentiment analysis, and neural machine translation, demonstrate that our enhanced attention regularization consistently improves performance across diverse applications.
Via
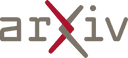
Feb 10, 2025
Abstract:A language can have different varieties. These varieties can affect the performance of natural language processing (NLP) models, including large language models (LLMs), which are often trained on data from widely spoken varieties. This paper introduces a novel and cost-effective approach to benchmark model performance across language varieties. We argue that international online review platforms, such as Booking.com, can serve as effective data sources for constructing datasets that capture comments in different language varieties from similar real-world scenarios, like reviews for the same hotel with the same rating using the same language (e.g., Mandarin Chinese) but different language varieties (e.g., Taiwan Mandarin, Mainland Mandarin). To prove this concept, we constructed a contextually aligned dataset comprising reviews in Taiwan Mandarin and Mainland Mandarin and tested six LLMs in a sentiment analysis task. Our results show that LLMs consistently underperform in Taiwan Mandarin.
* Accepted by 2025 Annual Conference of the Nations of the Americas
Chapter of the Association for Computational Linguistics (NAACL), theme track
Via
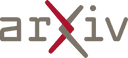