What is Recommendation? Recommendation is the task of providing personalized suggestions to users based on their preferences and behavior.
Papers and Code
Jun 18, 2025
Abstract:Loss functions play a pivotal role in optimizing recommendation models. Among various loss functions, Softmax Loss (SL) and Cosine Contrastive Loss (CCL) are particularly effective. Their theoretical connections and differences warrant in-depth exploration. This work conducts comprehensive analyses of these losses, yielding significant insights: 1) Common strengths -- both can be viewed as augmentations of traditional losses with Distributional Robust Optimization (DRO), enhancing robustness to distributional shifts; 2) Respective limitations -- stemming from their use of different distribution distance metrics in DRO optimization, SL exhibits high sensitivity to false negative instances, whereas CCL suffers from low data utilization. To address these limitations, this work proposes a new loss function, DrRL, which generalizes SL and CCL by leveraging R\'enyi-divergence in DRO optimization. DrRL incorporates the advantageous structures of both SL and CCL, and can be demonstrated to effectively mitigate their limitations. Extensive experiments have been conducted to validate the superiority of DrRL on both recommendation accuracy and robustness.
* AAAI 2025
Via
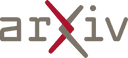
Jun 18, 2025
Abstract:Ensuring equitable public transit access remains challenging, particularly in densely populated cities like New York City (NYC), where low-income and minority communities often face limited transit accessibility. Bike-sharing systems (BSS) can bridge these equity gaps by providing affordable first- and last-mile connections. However, strategically expanding BSS into underserved neighborhoods is difficult due to uncertain bike-sharing demand at newly planned ("cold-start") station locations and limitations in traditional accessibility metrics that may overlook realistic bike usage potential. We introduce Transit for All (TFA), a spatial computing framework designed to guide the equitable expansion of BSS through three components: (1) spatially-informed bike-sharing demand prediction at cold-start stations using region representation learning that integrates multimodal geospatial data, (2) comprehensive transit accessibility assessment leveraging our novel weighted Public Transport Accessibility Level (wPTAL) by combining predicted bike-sharing demand with conventional transit accessibility metrics, and (3) strategic recommendations for new bike station placements that consider potential ridership and equity enhancement. Using NYC as a case study, we identify transit accessibility gaps that disproportionately impact low-income and minority communities in historically underserved neighborhoods. Our results show that strategically placing new stations guided by wPTAL notably reduces disparities in transit access related to economic and demographic factors. From our study, we demonstrate that TFA provides practical guidance for urban planners to promote equitable transit and enhance the quality of life in underserved urban communities.
Via
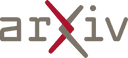
Jun 18, 2025
Abstract:Family caregivers of individuals with Alzheimer's Disease and Related Dementia (AD/ADRD) face significant emotional and logistical challenges that place them at heightened risk for stress, anxiety, and depression. Although recent advances in generative AI -- particularly large language models (LLMs) -- offer new opportunities to support mental health, little is known about how caregivers perceive and engage with such technologies. To address this gap, we developed Carey, a GPT-4o-based chatbot designed to provide informational and emotional support to AD/ADRD caregivers. Using Carey as a technology probe, we conducted semi-structured interviews with 16 family caregivers following scenario-driven interactions grounded in common caregiving stressors. Through inductive coding and reflexive thematic analysis, we surface a systemic understanding of caregiver needs and expectations across six themes -- on-demand information access, emotional support, safe space for disclosure, crisis management, personalization, and data privacy. For each of these themes, we also identified the nuanced tensions in the caregivers' desires and concerns. We present a mapping of caregiver needs, AI chatbot's strengths, gaps, and design recommendations. Our findings offer theoretical and practical insights to inform the design of proactive, trustworthy, and caregiver-centered AI systems that better support the evolving mental health needs of AD/ADRD caregivers.
Via
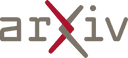
Jun 18, 2025
Abstract:Generative recommendation is emerging as a powerful paradigm that directly generates item predictions, moving beyond traditional matching-based approaches. However, current methods face two key challenges: token-item misalignment, where uniform token-level modeling ignores item-level granularity that is critical for collaborative signal learning, and semantic-collaborative signal entanglement, where collaborative and semantic signals exhibit distinct distributions yet are fused in a unified embedding space, leading to conflicting optimization objectives that limit the recommendation performance. To address these issues, we propose DiscRec, a novel framework that enables Disentangled Semantic-Collaborative signal modeling with flexible fusion for generative Recommendation.First, DiscRec introduces item-level position embeddings, assigned based on indices within each semantic ID, enabling explicit modeling of item structure in input token sequences.Second, DiscRec employs a dual-branch module to disentangle the two signals at the embedding layer: a semantic branch encodes semantic signals using original token embeddings, while a collaborative branch applies localized attention restricted to tokens within the same item to effectively capture collaborative signals. A gating mechanism subsequently fuses both branches while preserving the model's ability to model sequential dependencies. Extensive experiments on four real-world datasets demonstrate that DiscRec effectively decouples these signals and consistently outperforms state-of-the-art baselines. Our codes are available on https://github.com/Ten-Mao/DiscRec.
Via
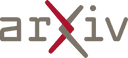
Jun 17, 2025
Abstract:This paper focuses on small-scale one-class classification with some negative samples available. We propose Generalized Reference Kernel with Negative Samples (GRKneg) for One-class Support Vector Machine (OC-SVM). We study different ways to select/generate the reference vectors and recommend an approach for the problem at hand. It is worth noting that the proposed method does not use any labels in the model optimization but uses the original OC-SVM implementation. Only the kernel used in the process is improved using the negative data. We compare our method with the standard OC-SVM and with the binary Support Vector Machine (SVM) using different amounts of negative samples. Our approach consistently outperforms the standard OC-SVM using Radial Basis Function kernel. When there are plenty of negative samples, the binary SVM outperforms the one-class approaches as expected, but we show that for the lowest numbers of negative samples the proposed approach clearly outperforms the binary SVM.
* Accepted to EUSIPCO2025
Via
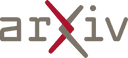
Jun 17, 2025
Abstract:Off-policy learning (OPL) in contextual bandits aims to learn a decision-making policy that maximizes the target rewards by using only historical interaction data collected under previously developed policies. Unfortunately, when rewards are only partially observed, the effectiveness of OPL degrades severely. Well-known examples of such partial rewards include explicit ratings in content recommendations, conversion signals on e-commerce platforms that are partial due to delay, and the issue of censoring in medical problems. One possible solution to deal with such partial rewards is to use secondary rewards, such as dwelling time, clicks, and medical indicators, which are more densely observed. However, relying solely on such secondary rewards can also lead to poor policy learning since they may not align with the target reward. Thus, this work studies a new and general problem of OPL where the goal is to learn a policy that maximizes the expected target reward by leveraging densely observed secondary rewards as supplemental data. We then propose a new method called Hybrid Policy Optimization for Partially-Observed Reward (HyPeR), which effectively uses the secondary rewards in addition to the partially-observed target reward to achieve effective OPL despite the challenging scenario. We also discuss a case where we aim to optimize not only the expected target reward but also the expected secondary rewards to some extent; counter-intuitively, we will show that leveraging the two objectives is in fact advantageous also for the optimization of only the target reward. Along with statistical analysis of our proposed methods, empirical evaluations on both synthetic and real-world data show that HyPeR outperforms existing methods in various scenarios.
* International Conference on Learning Representations (ICLR) 2025
* 10 pages, 5 figures. Published as a conference paper at ICLR 2025
Via
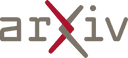
Jun 17, 2025
Abstract:This study aims at comparing two sequential recommender systems: Self-Attention based Sequential Recommendation (SASRec), and Beyond Self-Attention based Sequential Recommendation (BSARec) in order to check the improvement frequency enhancement - the added element in BSARec - has on recommendations. The models in the study, have been re-implemented with a common base-structure from EasyRec, with the aim of obtaining a fair and reproducible comparison. The results obtained displayed how BSARec, by including bias terms for frequency enhancement, does indeed outperform SASRec, although the increases in performance obtained, are not as high as those presented by the authors. This work aims at offering an overview on existing methods, and most importantly at underlying the importance of implementation details for performance comparison.
Via
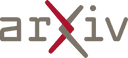
Jun 17, 2025
Abstract:Generative AI tools have become more prevalent in engineering workflows, particularly through chatbots and code assistants. As the perceived accuracy of these tools improves, questions arise about whether and how those who work in high-precision domains might maintain vigilance for errors, and what other aspects of using such tools might trouble their work. This paper analyzes interviews with hardware and software engineers, and their collaborators, who work in integrated circuit design to identify the role accuracy plays in their use of generative AI tools and what other forms of trouble they face in using such tools. The paper inventories these forms of trouble, which are then mapped to elements of generative AI systems, to conclude that controlling the context of interactions between engineers and the generative AI tools is one of the largest challenges they face. The paper concludes with recommendations for mitigating this form of trouble by increasing the ability to control context interactively.
Via
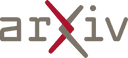
Jun 17, 2025
Abstract:Human language production exhibits remarkable richness and variation, reflecting diverse communication styles and intents. However, this variation is often overlooked in summarization evaluation. While having multiple reference summaries is known to improve correlation with human judgments, the impact of using different reference sets on reference-based metrics has not been systematically investigated. This work examines the sensitivity of widely used reference-based metrics in relation to the choice of reference sets, analyzing three diverse multi-reference summarization datasets: SummEval, GUMSum, and DUC2004. We demonstrate that many popular metrics exhibit significant instability. This instability is particularly concerning for n-gram-based metrics like ROUGE, where model rankings vary depending on the reference sets, undermining the reliability of model comparisons. We also collect human judgments on LLM outputs for genre-diverse data and examine their correlation with metrics to supplement existing findings beyond newswire summaries, finding weak-to-no correlation. Taken together, we recommend incorporating reference set variation into summarization evaluation to enhance consistency alongside correlation with human judgments, especially when evaluating LLMs.
* 17 pages, 13 figures
Via
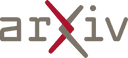
Jun 17, 2025
Abstract:Recent advancements in Large Language Models (LLMs) have significantly propelled the development of Conversational Recommendation Agents (CRAs). However, these agents often generate short-sighted responses that fail to sustain user guidance and meet expectations. Although preference optimization has proven effective in aligning LLMs with user expectations, it remains costly and performs poorly in multi-turn dialogue. To address this challenge, we introduce a novel multi-turn preference optimization (MTPO) paradigm ECPO, which leverages Expectation Confirmation Theory to explicitly model the evolution of user satisfaction throughout multi-turn dialogues, uncovering the underlying causes of dissatisfaction. These causes can be utilized to support targeted optimization of unsatisfactory responses, thereby achieving turn-level preference optimization. ECPO ingeniously eliminates the significant sampling overhead of existing MTPO methods while ensuring the optimization process drives meaningful improvements. To support ECPO, we introduce an LLM-based user simulator, AILO, to simulate user feedback and perform expectation confirmation during conversational recommendations. Experimental results show that ECPO significantly enhances CRA's interaction capabilities, delivering notable improvements in both efficiency and effectiveness over existing MTPO methods.
* Accepted to Findings of ACL 2025
Via
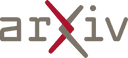