Topic:Information Extraction
What is Information Extraction? Information extraction is the process of automatically extracting structured information from unstructured text data.
Papers and Code
Jun 13, 2025
Abstract:With the growing number of users in multi-user multiple-input multiple-output (MU-MIMO) systems, demodulation reference signals (DMRSs) are efficiently multiplexed in the code domain via orthogonal cover codes (OCC) to ensure orthogonality and minimize pilot interference. In this paper, we investigate uplink DMRS-based channel estimation for MU-MIMO systems with Type II OCC pattern standardized in 3GPP Release 18, leveraging location-specific statistical channel state information (SCSI) to enhance performance. Specifically, we propose a SCSI-assisted Bayesian channel estimator (SA-BCE) based on the minimum mean square error criterion to suppress the pilot interference and noise, albeit at the cost of cubic computational complexity due to matrix inversions. To reduce this complexity while maintaining performance, we extend the scheme to a windowed version (SA-WBCE), which incorporates antenna-frequency domain windowing and beam-delay domain processing to exploit asymptotic sparsity and mitigate energy leakage in practical systems. To avoid the frequent real-time SCSI acquisition, we construct a grid-based location-specific SCSI database based on the principle of spatial consistency, and subsequently leverage the uplink received signals within each grid to extract the SCSI. Facilitated by the multilinear structure of wireless channels, we formulate the SCSI acquisition problem within each grid as a tensor decomposition problem, where the factor matrices are parameterized by the multi-path powers, delays, and angles. The computational complexity of SCSI acquisition can be significantly reduced by exploiting the Vandermonde structure of the factor matrices. Simulation results demonstrate that the proposed location-specific SCSI database construction method achieves high accuracy, while the SA-BCE and SA-WBCE significantly outperform state-of-the-art benchmarks in MU-MIMO systems.
* This work has been submitted to the IEEE for possible publication
Via
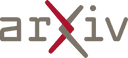
Jun 10, 2025
Abstract:Large Language Models (LLMs) are increasingly capable but often require significant guidance or extensive interaction history to perform effectively in complex, interactive environments. Existing methods may struggle with adapting to new information or efficiently utilizing past experiences for multi-step reasoning without fine-tuning. We introduce a novel LLM agent framework that enhances planning capabilities through in-context learning, facilitated by atomic fact augmentation and a recursive lookahead search. Our agent learns to extract task-critical ``atomic facts'' from its interaction trajectories. These facts dynamically augment the prompts provided to LLM-based components responsible for action proposal, latent world model simulation, and state-value estimation. Planning is performed via a depth-limited lookahead search, where the LLM simulates potential trajectories and evaluates their outcomes, guided by the accumulated facts and interaction history. This approach allows the agent to improve its understanding and decision-making online, leveraging its experience to refine its behavior without weight updates. We provide a theoretical motivation linking performance to the quality of fact-based abstraction and LLM simulation accuracy. Empirically, our agent demonstrates improved performance and adaptability on challenging interactive tasks, achieving more optimal behavior as it accumulates experience, showcased in tasks such as TextFrozenLake and ALFWorld.
* 9-page main paper, 1 figure. Accepted for an Oral presentation at the
First Workshop on Computer Use Agents (ICML 2025), Vancouver, Canada
Via
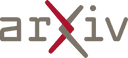
Jun 05, 2025
Abstract:Assessing scientific claims requires identifying, extracting, and reasoning with multimodal data expressed in information-rich figures in scientific literature. Despite the large body of work in scientific QA, figure captioning, and other multimodal reasoning tasks over chart-based data, there are no readily usable multimodal benchmarks that directly test claim verification abilities. To remedy this gap, we introduce a new benchmark MuSciClaims accompanied by diagnostics tasks. We automatically extract supported claims from scientific articles, which we manually perturb to produce contradicted claims. The perturbations are designed to test for a specific set of claim verification capabilities. We also introduce a suite of diagnostic tasks that help understand model failures. Our results show most vision-language models are poor (~0.3-0.5 F1), with even the best model only achieving 0.77 F1. They are also biased towards judging claims as supported, likely misunderstanding nuanced perturbations within the claims. Our diagnostics show models are bad at localizing correct evidence within figures, struggle with aggregating information across modalities, and often fail to understand basic components of the figure.
Via
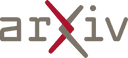
Jun 11, 2025
Abstract:Understanding cause and effect relationships remains a formidable challenge for Large Language Models (LLMs), particularly in specialized domains where reasoning requires more than surface-level correlations. Retrieval-Augmented Generation (RAG) improves factual accuracy, but standard RAG pipelines treat evidence as flat context, lacking the structure required to model true causal dependencies. We introduce Causal-Chain RAG (CC-RAG), a novel approach that integrates zero-shot triple extraction and theme-aware graph chaining into the RAG pipeline, enabling structured multi-hop inference. Given a domain specific corpus, CC-RAG constructs a Directed Acyclic Graph (DAG) of <cause, relation, effect> triples and uses forward/backward chaining to guide structured answer generation. Experiments on two real-world domains: Bitcoin price fluctuations and Gaucher disease, show that CC-RAG outperforms standard RAG and zero-shot LLMs in chain similarity, information density, and lexical diversity. Both LLM-as-a-Judge and human evaluations consistently favor CC-RAG. Our results demonstrate that explicitly modeling causal structure enables LLMs to generate more accurate and interpretable responses, especially in specialized domains where flat retrieval fails.
Via
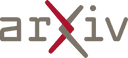
Jun 13, 2025
Abstract:Learning medical visual representations directly from paired images and reports through multimodal self-supervised learning has emerged as a novel and efficient approach to digital diagnosis in recent years. However, existing models suffer from several severe limitations. 1) neglecting the selection of negative samples, resulting in the scarcity of hard negatives and the inclusion of false negatives; 2) focusing on global feature extraction, but overlooking the fine-grained local details that are crucial for medical image recognition tasks; and 3) contrastive learning primarily targets high-level features but ignoring low-level details which are essential for accurate medical analysis. Motivated by these critical issues, this paper presents a Cross-Modal Cluster-Guided Negative Sampling (CM-CGNS) method with two-fold ideas. First, it extends the k-means clustering used for local text features in the single-modal domain to the multimodal domain through cross-modal attention. This improvement increases the number of negative samples and boosts the model representation capability. Second, it introduces a Cross-Modal Masked Image Reconstruction (CM-MIR) module that leverages local text-to-image features obtained via cross-modal attention to reconstruct masked local image regions. This module significantly strengthens the model's cross-modal information interaction capabilities and retains low-level image features essential for downstream tasks. By well handling the aforementioned limitations, the proposed CM-CGNS can learn effective and robust medical visual representations suitable for various recognition tasks. Extensive experimental results on classification, detection, and segmentation tasks across five downstream datasets show that our method outperforms state-of-the-art approaches on multiple metrics, verifying its superior performance.
* This work has been submitted to the IEEE TMI for possible
publication. Our code is available at https://github.com/violet-42/CM-CGNS
Via
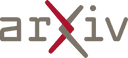
Jun 09, 2025
Abstract:Multimodal retrieval-augmented generation (RAG) systems enhance large vision-language models by integrating cross-modal knowledge, enabling their increasing adoption across real-world multimodal tasks. These knowledge databases may contain sensitive information that requires privacy protection. However, multimodal RAG systems inherently grant external users indirect access to such data, making them potentially vulnerable to privacy attacks, particularly membership inference attacks (MIAs). % Existing MIA methods targeting RAG systems predominantly focus on the textual modality, while the visual modality remains relatively underexplored. To bridge this gap, we propose MrM, the first black-box MIA framework targeted at multimodal RAG systems. It utilizes a multi-object data perturbation framework constrained by counterfactual attacks, which can concurrently induce the RAG systems to retrieve the target data and generate information that leaks the membership information. Our method first employs an object-aware data perturbation method to constrain the perturbation to key semantics and ensure successful retrieval. Building on this, we design a counterfact-informed mask selection strategy to prioritize the most informative masked regions, aiming to eliminate the interference of model self-knowledge and amplify attack efficacy. Finally, we perform statistical membership inference by modeling query trials to extract features that reflect the reconstruction of masked semantics from response patterns. Experiments on two visual datasets and eight mainstream commercial visual-language models (e.g., GPT-4o, Gemini-2) demonstrate that MrM achieves consistently strong performance across both sample-level and set-level evaluations, and remains robust under adaptive defenses.
Via
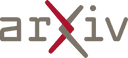
Jun 09, 2025
Abstract:BridgeNet is a novel hybrid framework that integrates convolutional neural networks with physics-informed neural networks to efficiently solve non-linear, high-dimensional Fokker-Planck equations (FPEs). Traditional PINNs, which typically rely on fully connected architectures, often struggle to capture complex spatial hierarchies and enforce intricate boundary conditions. In contrast, BridgeNet leverages adaptive CNN layers for effective local feature extraction and incorporates a dynamically weighted loss function that rigorously enforces physical constraints. Extensive numerical experiments across various test cases demonstrate that BridgeNet not only achieves significantly lower error metrics and faster convergence compared to conventional PINN approaches but also maintains robust stability in high-dimensional settings. This work represents a substantial advancement in computational physics, offering a scalable and accurate solution methodology with promising applications in fields ranging from financial mathematics to complex system dynamics.
Via
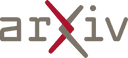
Jun 07, 2025
Abstract:As integrated circuit scale grows and design complexity rises, effective circuit representation helps support logic synthesis, formal verification, and other automated processes in electronic design automation. And-Inverter Graphs (AIGs), as a compact and canonical structure, are widely adopted for representing Boolean logic in these workflows. However, the increasing complexity and integration density of modern circuits introduce structural heterogeneity and global logic information loss in AIGs, posing significant challenges to accurate circuit modeling. To address these issues, we propose FuncGNN, which integrates hybrid feature aggregation to extract multi-granularity topological patterns, thereby mitigating structural heterogeneity and enhancing logic circuit representations. FuncGNN further introduces gate-aware normalization that adapts to circuit-specific gate distributions, improving robustness to structural heterogeneity. Finally, FuncGNN employs multi-layer integration to merge intermediate features across layers, effectively synthesizing local and global semantic information for comprehensive logic representations. Experimental results on two logic-level analysis tasks (i.e., signal probability prediction and truth-table distance prediction) demonstrate that FuncGNN outperforms existing state-of-the-art methods, achieving improvements of 2.06% and 18.71%, respectively, while reducing training time by approximately 50.6% and GPU memory usage by about 32.8%.
Via
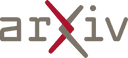
Jun 09, 2025
Abstract:Automated pollen recognition is vital to paleoclimatology, biodiversity monitoring, and public health, yet conventional methods are hampered by inefficiency and subjectivity. Existing deep learning models often struggle to achieve the requisite localization accuracy for microscopic targets like pollen, which are characterized by their minute size, indistinct edges, and complex backgrounds. To overcome this limitation, we introduce HieraEdgeNet, a multi-scale edge-enhancement framework. The framework's core innovation is the introduction of three synergistic modules: the Hierarchical Edge Module (HEM), which explicitly extracts a multi-scale pyramid of edge features that corresponds to the semantic hierarchy at early network stages; the Synergistic Edge Fusion (SEF) module, for deeply fusing these edge priors with semantic information at each respective scale; and the Cross Stage Partial Omni-Kernel Module (CSPOKM), which maximally refines the most detail-rich feature layers using an Omni-Kernel operator - comprising anisotropic large-kernel convolutions and mixed-domain attention - all within a computationally efficient Cross-Stage Partial (CSP) framework. On a large-scale dataset comprising 120 pollen classes, HieraEdgeNet achieves a mean Average Precision (mAP@.5) of 0.9501, significantly outperforming state-of-the-art baseline models such as YOLOv12n and RT-DETR. Furthermore, qualitative analysis confirms that our approach generates feature representations that are more precisely focused on object boundaries. By systematically integrating edge information, HieraEdgeNet provides a robust and powerful solution for high-precision, high-efficiency automated detection of microscopic objects.
Via
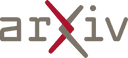
Jun 07, 2025
Abstract:Image Representation learning via input reconstruction is a common technique in machine learning for generating representations that can be effectively utilized by arbitrary downstream tasks. A well-established approach is using autoencoders to extract latent representations at the network's compression point. These representations are valuable because they retain essential information necessary for reconstructing the original input from the compressed latent space. In this paper, we propose an alternative learning objective. Instead of using the raw input as the reconstruction target, we employ the Discrete Fourier Transform (DFT) of the input. The DFT provides meaningful global information at each frequency level, making individual frequency components useful as separate learning targets. When dealing with multidimensional input data, the DFT offers remarkable flexibility by enabling selective transformation across specific dimensions while preserving others in the computation. Moreover, certain types of input exhibit distinct patterns in their frequency distributions, where specific frequency components consistently contain most of the magnitude, allowing us to focus on a subset of frequencies rather than the entire spectrum. These characteristics position the DFT as a viable learning objective for representation learning and we validate our approach by achieving 52.8% top-1 accuracy on CIFAR-10 with ResNet-50 and outperforming the traditional autoencoder by 12.8 points under identical architectural configurations. Additionally, we demonstrate that training on only the lower-frequency components - those with the highest magnitudes yields results comparable to using the full frequency spectrum, with only minimal reductions in accuracy.
* Preprint
Via
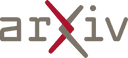