Topic:Information Extraction
What is Information Extraction? Information extraction is the process of automatically extracting structured information from unstructured text data.
Papers and Code
Jun 07, 2025
Abstract:As integrated circuit scale grows and design complexity rises, effective circuit representation helps support logic synthesis, formal verification, and other automated processes in electronic design automation. And-Inverter Graphs (AIGs), as a compact and canonical structure, are widely adopted for representing Boolean logic in these workflows. However, the increasing complexity and integration density of modern circuits introduce structural heterogeneity and global logic information loss in AIGs, posing significant challenges to accurate circuit modeling. To address these issues, we propose FuncGNN, which integrates hybrid feature aggregation to extract multi-granularity topological patterns, thereby mitigating structural heterogeneity and enhancing logic circuit representations. FuncGNN further introduces gate-aware normalization that adapts to circuit-specific gate distributions, improving robustness to structural heterogeneity. Finally, FuncGNN employs multi-layer integration to merge intermediate features across layers, effectively synthesizing local and global semantic information for comprehensive logic representations. Experimental results on two logic-level analysis tasks (i.e., signal probability prediction and truth-table distance prediction) demonstrate that FuncGNN outperforms existing state-of-the-art methods, achieving improvements of 2.06% and 18.71%, respectively, while reducing training time by approximately 50.6% and GPU memory usage by about 32.8%.
Via
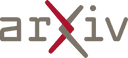
Jun 07, 2025
Abstract:Image Representation learning via input reconstruction is a common technique in machine learning for generating representations that can be effectively utilized by arbitrary downstream tasks. A well-established approach is using autoencoders to extract latent representations at the network's compression point. These representations are valuable because they retain essential information necessary for reconstructing the original input from the compressed latent space. In this paper, we propose an alternative learning objective. Instead of using the raw input as the reconstruction target, we employ the Discrete Fourier Transform (DFT) of the input. The DFT provides meaningful global information at each frequency level, making individual frequency components useful as separate learning targets. When dealing with multidimensional input data, the DFT offers remarkable flexibility by enabling selective transformation across specific dimensions while preserving others in the computation. Moreover, certain types of input exhibit distinct patterns in their frequency distributions, where specific frequency components consistently contain most of the magnitude, allowing us to focus on a subset of frequencies rather than the entire spectrum. These characteristics position the DFT as a viable learning objective for representation learning and we validate our approach by achieving 52.8% top-1 accuracy on CIFAR-10 with ResNet-50 and outperforming the traditional autoencoder by 12.8 points under identical architectural configurations. Additionally, we demonstrate that training on only the lower-frequency components - those with the highest magnitudes yields results comparable to using the full frequency spectrum, with only minimal reductions in accuracy.
* Preprint
Via
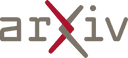
Jun 09, 2025
Abstract:BridgeNet is a novel hybrid framework that integrates convolutional neural networks with physics-informed neural networks to efficiently solve non-linear, high-dimensional Fokker-Planck equations (FPEs). Traditional PINNs, which typically rely on fully connected architectures, often struggle to capture complex spatial hierarchies and enforce intricate boundary conditions. In contrast, BridgeNet leverages adaptive CNN layers for effective local feature extraction and incorporates a dynamically weighted loss function that rigorously enforces physical constraints. Extensive numerical experiments across various test cases demonstrate that BridgeNet not only achieves significantly lower error metrics and faster convergence compared to conventional PINN approaches but also maintains robust stability in high-dimensional settings. This work represents a substantial advancement in computational physics, offering a scalable and accurate solution methodology with promising applications in fields ranging from financial mathematics to complex system dynamics.
Via
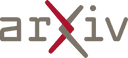
Jun 09, 2025
Abstract:Automated pollen recognition is vital to paleoclimatology, biodiversity monitoring, and public health, yet conventional methods are hampered by inefficiency and subjectivity. Existing deep learning models often struggle to achieve the requisite localization accuracy for microscopic targets like pollen, which are characterized by their minute size, indistinct edges, and complex backgrounds. To overcome this limitation, we introduce HieraEdgeNet, a multi-scale edge-enhancement framework. The framework's core innovation is the introduction of three synergistic modules: the Hierarchical Edge Module (HEM), which explicitly extracts a multi-scale pyramid of edge features that corresponds to the semantic hierarchy at early network stages; the Synergistic Edge Fusion (SEF) module, for deeply fusing these edge priors with semantic information at each respective scale; and the Cross Stage Partial Omni-Kernel Module (CSPOKM), which maximally refines the most detail-rich feature layers using an Omni-Kernel operator - comprising anisotropic large-kernel convolutions and mixed-domain attention - all within a computationally efficient Cross-Stage Partial (CSP) framework. On a large-scale dataset comprising 120 pollen classes, HieraEdgeNet achieves a mean Average Precision (mAP@.5) of 0.9501, significantly outperforming state-of-the-art baseline models such as YOLOv12n and RT-DETR. Furthermore, qualitative analysis confirms that our approach generates feature representations that are more precisely focused on object boundaries. By systematically integrating edge information, HieraEdgeNet provides a robust and powerful solution for high-precision, high-efficiency automated detection of microscopic objects.
Via
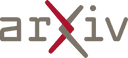
Jun 13, 2025
Abstract:Learning medical visual representations directly from paired images and reports through multimodal self-supervised learning has emerged as a novel and efficient approach to digital diagnosis in recent years. However, existing models suffer from several severe limitations. 1) neglecting the selection of negative samples, resulting in the scarcity of hard negatives and the inclusion of false negatives; 2) focusing on global feature extraction, but overlooking the fine-grained local details that are crucial for medical image recognition tasks; and 3) contrastive learning primarily targets high-level features but ignoring low-level details which are essential for accurate medical analysis. Motivated by these critical issues, this paper presents a Cross-Modal Cluster-Guided Negative Sampling (CM-CGNS) method with two-fold ideas. First, it extends the k-means clustering used for local text features in the single-modal domain to the multimodal domain through cross-modal attention. This improvement increases the number of negative samples and boosts the model representation capability. Second, it introduces a Cross-Modal Masked Image Reconstruction (CM-MIR) module that leverages local text-to-image features obtained via cross-modal attention to reconstruct masked local image regions. This module significantly strengthens the model's cross-modal information interaction capabilities and retains low-level image features essential for downstream tasks. By well handling the aforementioned limitations, the proposed CM-CGNS can learn effective and robust medical visual representations suitable for various recognition tasks. Extensive experimental results on classification, detection, and segmentation tasks across five downstream datasets show that our method outperforms state-of-the-art approaches on multiple metrics, verifying its superior performance.
* This work has been submitted to the IEEE TMI for possible
publication. Our code is available at https://github.com/violet-42/CM-CGNS
Via
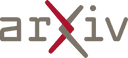
Jun 07, 2025
Abstract:Audio-visual speech separation (AVSS) aims to extract a target speech signal from a mixed signal by leveraging both auditory and visual (lip movement) cues. However, most existing AVSS methods exhibit complex architectures and rely on future context, operating offline, which renders them unsuitable for real-time applications. Inspired by the pipeline of RTFSNet, we propose a novel streaming AVSS model, named Swift-Net, which enhances the causal processing capabilities required for real-time applications. Swift-Net adopts a lightweight visual feature extraction module and an efficient fusion module for audio-visual integration. Additionally, Swift-Net employs Grouped SRUs to integrate historical information across different feature spaces, thereby improving the utilization efficiency of historical information. We further propose a causal transformation template to facilitate the conversion of non-causal AVSS models into causal counterparts. Experiments on three standard benchmark datasets (LRS2, LRS3, and VoxCeleb2) demonstrated that under causal conditions, our proposed Swift-Net exhibited outstanding performance, highlighting the potential of this method for processing speech in complex environments.
* 8 pages, 5 figures
Via
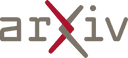
Jun 09, 2025
Abstract:Recent advancements in diffusion models have enabled high-fidelity and photorealistic image generation across diverse applications. However, these models also present security and privacy risks, including copyright violations, sensitive information leakage, and the creation of harmful or offensive content that could be exploited maliciously. In this study, we uncover a novel security threat where an attacker leverages diffusion model APIs to generate synthetic images, which are then used to train a high-performing substitute model. This enables the attacker to execute model extraction and transfer-based adversarial attacks on black-box classification models with minimal queries, without needing access to the original training data. The generated images are sufficiently high-resolution and diverse to train a substitute model whose outputs closely match those of the target model. Across the seven benchmarks, including CIFAR and ImageNet subsets, our method shows an average improvement of 27.37% over state-of-the-art methods while using just 0.01 times of the query budget, achieving a 98.68% success rate in adversarial attacks on the target model.
Via
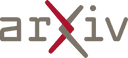
Jun 12, 2025
Abstract:Music-to-dance generation aims to synthesize human dance motion conditioned on musical input. Despite recent progress, significant challenges remain due to the semantic gap between music and dance motion, as music offers only abstract cues, such as melody, groove, and emotion, without explicitly specifying the physical movements. Moreover, a single piece of music can produce multiple plausible dance interpretations. This one-to-many mapping demands additional guidance, as music alone provides limited information for generating diverse dance movements. The challenge is further amplified by the scarcity of paired music and dance data, which restricts the model\^a\u{A}\'Zs ability to learn diverse dance patterns. In this paper, we introduce DanceChat, a Large Language Model (LLM)-guided music-to-dance generation approach. We use an LLM as a choreographer that provides textual motion instructions, offering explicit, high-level guidance for dance generation. This approach goes beyond implicit learning from music alone, enabling the model to generate dance that is both more diverse and better aligned with musical styles. Our approach consists of three components: (1) an LLM-based pseudo instruction generation module that produces textual dance guidance based on music style and structure, (2) a multi-modal feature extraction and fusion module that integrates music, rhythm, and textual guidance into a shared representation, and (3) a diffusion-based motion synthesis module together with a multi-modal alignment loss, which ensures that the generated dance is aligned with both musical and textual cues. Extensive experiments on AIST++ and human evaluations show that DanceChat outperforms state-of-the-art methods both qualitatively and quantitatively.
Via
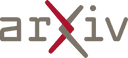
Jun 11, 2025
Abstract:Recent approaches have shown impressive proficiency in extracting and leveraging parametric knowledge from Large-Language Models (LLMs) and Vision-Language Models (VLMs). In this work, we consider how we can improve the identification and retrieval of videos related to complex real-world events by automatically extracting latent parametric knowledge about those events. We present Q2E: a Query-to-Event decomposition method for zero-shot multilingual text-to-video retrieval, adaptable across datasets, domains, LLMs, or VLMs. Our approach demonstrates that we can enhance the understanding of otherwise overly simplified human queries by decomposing the query using the knowledge embedded in LLMs and VLMs. We additionally show how to apply our approach to both visual and speech-based inputs. To combine this varied multimodal knowledge, we adopt entropy-based fusion scoring for zero-shot fusion. Through evaluations on two diverse datasets and multiple retrieval metrics, we demonstrate that Q2E outperforms several state-of-the-art baselines. Our evaluation also shows that integrating audio information can significantly improve text-to-video retrieval. We have released code and data for future research.
Via
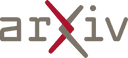
Jun 09, 2025
Abstract:Role-playing has emerged as an effective technique for enhancing the reasoning capabilities of large language models (LLMs). However, existing methods primarily rely on prompt engineering, which often lacks stability and interpretability. In this paper, we introduce Sparse Autoencoder Role-Playing Steering (SRPS), a novel framework that identifies and manipulates internal model features associated with role-playing behavior. Our approach extracts latent representations from role-play prompts, selects the most relevant features based on activation patterns, and constructs a steering vector that can be injected into the model's residual stream with controllable intensity. Our method enables fine-grained control over role-specific behavior and offers insights into how role information influences internal model activations. Extensive experiments across various reasoning benchmarks and model sizes demonstrate consistent performance gains. Notably, in the zero-shot chain-of-thought (CoT) setting, the accuracy of Llama3.1-8B on CSQA improves from 31.86% to 39.80%, while Gemma2-9B on SVAMP increases from 37.50% to 45.10%. These results highlight the potential of SRPS to enhance reasoning ability in LLMs, providing better interpretability and stability compared to traditional prompt-based role-playing.
* 21 pages, 8 figures, 8 tables
Via
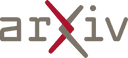